Bias, understood to be an inclination or prejudice for or against one person or group, especially in a way considered to be unfair, is rife in AI and ML. In fact, algorithmic bias in AI systems can take varied forms such as gender bias, racial prejudice and age discrimination.
In regard to bias in AI, it is widely recognized that there are two prominent types. These manifest themselves as cognitive bias, meaning that bias is inserted into algorithms through designers introducing them into models or using training data already inclusive of bias, and also lack of complete data, therefore not being representative of the population, culture and encompassing of all demographics.
Kishore Karra, Executive Director, Model Risk Governance & Review at J.P. Morgan recently discussed bias in AI models, suggesting that it is at an individual level which bias can creep in:
"We need to be aware of what kind of biases can be introduced into the models. Our inherent human vices, which have found their way into the data, into the model training data, will eventually find a way into the models outcome and the machine's decisions."
Kishore also recognized that bias can creep into machine learning models unintentionally and it's important to identify sources of bias. This however, may also be a case of substantial backdating. With AI Ethics being a buzzword at present, the spotlight is on large organizations and their practices, however, Kishore further suggested that the algorithms today, could be perpetuating bias from years previous, using Amazon's recruitment mishap as an example:
"The past 10 years of training data that the model was trained on was dominated by male applicants for these positions, and the machine quickly learned similar behavior. That is clear based on these algorithms, that bias can creep into machine learning models unintentionally and it's important to identify the sources of bias before turning the model and eliminate the possibility of the biases in the initial stages itself."
Diverse, Racial and Gender Bias
Alongside the hiring case at Amazon, we have seen examples of bias in Facebook ads, discrimination in healthcare focused AI and more. Another aspect of bias which often isn't recognized, is that it may well be due to the lack of diversity in the industry itself. Neeta Mundra of Salesforce recently discussed this, suggesting:
"When you talk about AI and as the technology is evolving, unconscious bias, or as I call it at times, subconscious bias, too, can also impact algorithms which are built in tech industry. Right? And if industry isn't diverse, then these algorithms which are built will not adapt to diverse ways of thinking.
It is also suggested that the use of larger data collection tools may not be the answer. Secretly utilized, or well disguised AI systems, prevalent in the US and China can be dangerous for marginalized people. Consequently, people don't have the option to opt out of these AI systems' biased surveillance. The tracking of people without their consent and knowledge, ultimately undermines those discriminated against, and it can also mitigate individuals' willingness to partake in the economy and culture.
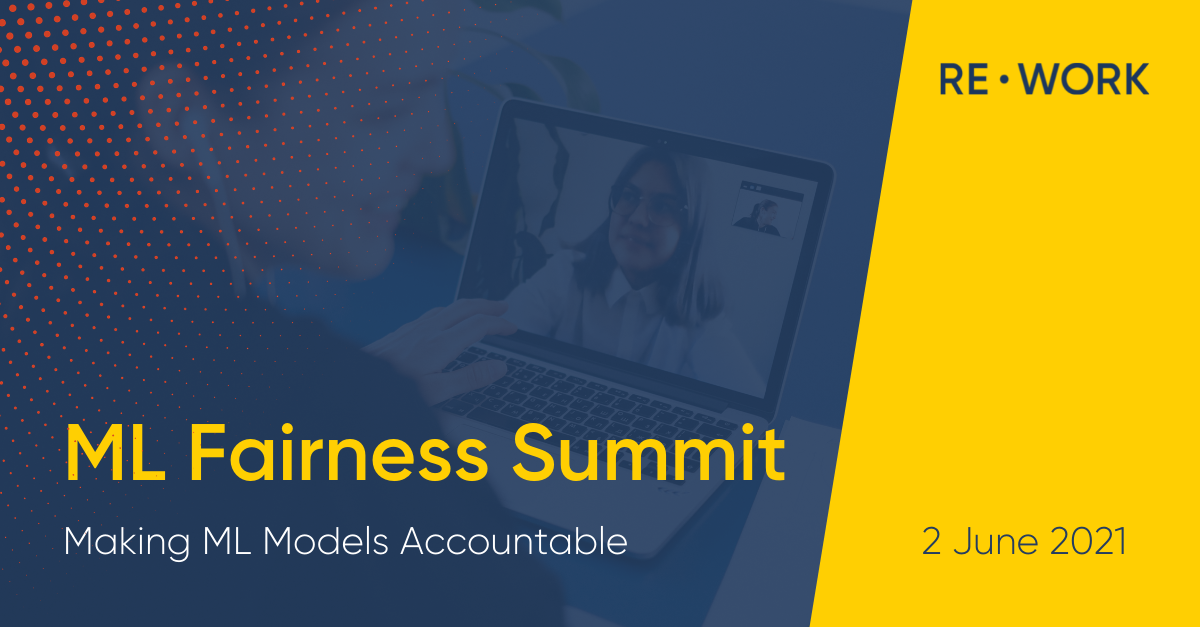
Alongside this, it also needs to be studied from the viewpoint of trust, which we spoke to Rachel Alexander about on a recent Women in AI Podcast:
"People will trust an artificial intelligence solution if they believe it makes decisions that are fair, respects their values, respects social norms, and they understand how the decision is made. They understand how to contest the decisions and they know their data is handled with respect and is secure” - Rachel Alexander, CEO, Omina
What is the answer?
If the answer was easy, there wouldn't be thousands of special minds deployed to work on this, however, there have been a few suggestions which could no doubt get us back on the correct path. The first, and arguably most critical question to be asked is "What is the root cause for introducing bias in AI systems, and how can it be prevented?" Whilst numerous forms of bias can infiltrate AI, the exclusion of sensitive data from AI systems, including gender, ethnicity or sexual identity, AI systems them learn to make decisions based on a lesser and still skewed data set.
Recent work at UC Berkeley (video below) suggested that training data has historically been built on male input data:
"There is indeed more men than women in the training data. So that alone creates a certain bias towards just more frequently predicting men" - Anna Rohrbach, Research Scientist, UC Berkeley
Whilst this can all paint somewhat of a stark picture, it is also key to remember that unlike human bias, algorithmic bias is ultimately able to be quantified and fixable if dealt with appropriately. We must keep trying to understand the true cases & reasons behind the bias, finding answers to allow for the deployment of safe and trustworthy AI.
Could all of this be simplified with regulatory input? Possibly, however, making AI models fairer and more ethical comes with a cost. Without regulatory pressure, companies find it easier to go without, which a research study from Gartner predicted would cause 85% of AI projects to deliver wrong outcomes due to bias in data, algorithms, or the teams responsible for managing them. Another potential barrier is the differentiation in regulation around the globe. The EU sees AI regulation under existing protections for human liberties. “Artificial intelligence must serve people, and therefore artificial intelligence must always comply with people’s rights” said Ursula von der Leyen, president of the European Commission, in a speech ahead of the release. However, this is not mirrored in the US or any other country, meaning that there is a stark difference in both understanding and limitations/restrictions of use.
There is still some skepticism surrounding the ethical implementation of AI going forward. Could it be impossible to totally eliminate bias? Not only can bias enter models and the pipeline at every stage, the cost can be vast and fairness/ethics are very much subjective without regulation. There is, however, a growing amount of thought being put into Ethical AI, with many organizations now on the case, but it could be some time before we not only have a royal standard of ethics and fairness in AI, but also organizations willing to put progress before profits.
Want to read more from RE•WORK? Here are the top read blogs of 2021:
- 5 AI Advancements in Finance, Insurance, & RegTech
- AI Experts Predict 2021 Trends
- 13 ‘Must-Read’ Papers from AI Experts
- The Difference Between Symbolic AI and Connectionist AI
- 30 Influential AI Presentations from 2019
You can also access all of our summit content on the AI Library here.