It feels as though 2019 has gone by in a flash, that said, it has been a year in which we have seen great advancement in AI application methods and technical discovery, paving the way for future development. We are incredibly grateful to have had the leading minds in AI & Deep Learning present their latest work at our summits in San Francisco, Boston, Montreal and more, so we thought we would share thirty of our highlight videos with you as we think everybody needs to see them!. (Some are hosted on our Videohub and some on our YouTube, but all are free to view!).
You can also see our top video presentations of Q1 2020 here.
1) Secure Deep Reinforcement Learning
Dawn Song, Professor, UC Berkeley.
We were delighted to be joined by Dawn at the Deep Reinforcement Learning Summit in June of 2019, presenting the latest industry research on Secure Deep Reinforcement Learning, covering both the lessons leant in the lead up to her presentation, current challenges faced for advancement, and the future direction of which her research is set to take. You can see Dawn's full presentation from June here.
2) Building Knowledge For AI Agents With Reinforcement Learning
Doina Precup, Research Team Lead, DeepMind.
Reinforcement Learning is somewhat of a hotbed for research, this year alone we have seen several presentations that have broken down the ins and outs of RL, that said, Doina's talk just last month gave us some new angles on the latest algorithmic development. Watch Doina discuss RL development at DeepMind here.
3) Developing Innovative Data Science Solutions To Increase Customer Retention
Natalie Jakomis, Group Director of Data, goCompare.
Customer retention is the set of actions that companies take to stop customers from leaving and to retain and grow as many as possible into loyal customers. This talk from Natalie shares information about the exciting journey GoCo group is on and the opportunities they have found to improve customer retention across its multiple brands. See Natalie's full talk here.
4) Generative Adversarial Networks - An Overview of Development
Ian Goodfellow, Director, Apple.
At the time of his presentation, Ian was a Senior Staff Research Scientist at Google and gave an insight into some of the latest breakthroughs in GANs. Dubbed the 'Godfather of GANs', who better to get an overview from than Ian? Post discussion, Ian had one of the longest question queues that we have seen at one of our summits, skip the queue and watch his presentation from the comfort of your PC here.
5) Understanding the limitations of AI: When Algorithms Fail
Timnit Gebru, Ethical AI Team, Google.
When presenting in San Francisco, Timnit showed some examples to examine the dire consequences of basing decisions entirely on machine learning based systems, and discussed recent work on auditing and exposing the gender and skin tone bias found in commercial gender classification systems. Ending her talk with the concept of an AI datasheet to standardize information for datasets and pre-trained models, in order to push the field as a whole towards transparency and accountability, this is not a presentation you want to miss! Watch the full presentation here.
6) Why aren't our AI Assistants smarter?
Cathy Pearl, Head of Conversation Design Outreach, Google.
Do our current crop of AI Assistants really use AI? If not, are they still useful? In this talk, Cathy walked the audience through the current state of AI Assistants, the challenges of building them, and speculated on what the future might bring. You can see more on her presentation here. We were also lucky enough to have Cathy on the Women in AI podcast after her talk, so make sure to give it a listen!
7) The Myth of the interpretable, Robust, Compact and High Performance Deep Neural Network
Sara Hooker, Artificial Intelligence Resident, Google Brain.
Between infancy and adulthood, the number of synapses in our brain first multiply and then fall. Despite losing 50% of all synapses between ages two and ten, the brain continues to function. The phrase “use it or lose it” is frequently used to describe the environmental influence of the learning process on synaptic pruning, however, there is a little scientific consensus on what exactly is lost. In this talk, Sara explored what is lost when we prune a deep neural network. Watch here.
8) 3D Perception of Human Appearance and Geometry in the Wild
Natalia Neverova, Research Scientist, Facebook AI Research (FAIR).
In this talk, Natalia focussed on recent work at Facebook on learning object point correspondences for dense human pose estimation "in the wild" from images and videos, performed in real time. Natalia also covered some of applications of the DensePose framework to dense pose transfer and avatar synthesis, as well as real time reconstruction of 3D geometry of a human body. See more from Natalia here.
9) Few-Shot Learning: Thoughts On Where We Should Be Going
Hugo Larochelle, Director, Google Brain Montreal
Few-shot learning is the problem of learning new tasks from little amounts of labeled data. This is achieved by performing a form of transfer learning, from the data of many other existing tasks. This topic has gained tremendous interest in the past few years, and you can see more of it in Hugo's talk here.
10) Safety First: AI To Detect Distracted Driving
Priya Sundararaman, Principal Data Scientist, State Farm
Distracted driving is one of the leading causes of auto accidents according to the National Highway Traffic Safety Administration (NHTSA). In this presentation, Priya demonstrated the use of Artificial Intelligence (AI) to analyze driver images and identify distracted driving behavior autonomously. See Priya's full presentation here.
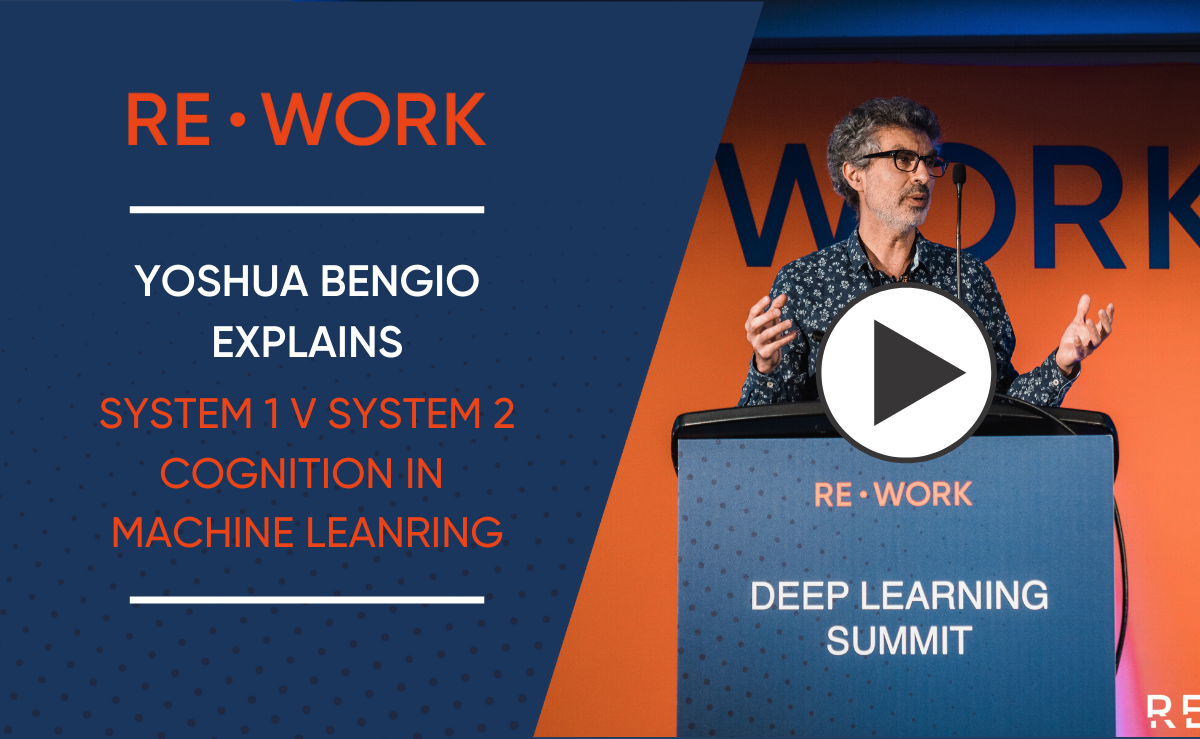
11) AI for Humanity: How AI Could Benefit Us All
Myriam Cote, Director, AI for Humanity, MILA
In the past 50 years, we have gone through many technological changes which have brought us a big deal of surprises and consequences. The advent of AI could be scary sometimes because we naturally fear the unknown. What if another story could be told? The story of AI that is good for us all. Myriam tried to calm us all down in Montreal, see what she had to say here.
12) Generalizing The Lottery Ticket Hypothesis Across Datasets and Optimizers and Beyond Supervised Image Classification
Ari Morcos, Research Scientist, Facebook AI Research (FAIR).
Do winning tickets contain generic inductive biases for training or are they just overfitted to a particular problem? Is the lottery ticket phenomenon simply an artifact of image classification or is it present in other domains as well? In this talk, Ari discusses recent work at Facebook to address both of these critical questions! See Ari's full talk here.
13) Tricks for Deep Learning Implemented at British Petroleum
Huma Lodhi, Data Scientist, British Petroleum.
Intelligent methodologies driven by deep learning have been focus of academic researchers and industry practitioners. The systems based on these techniques are showing state-of-the-art performance in areas ranging from Insurance to Oil & Gas. This talk presents a few tricks to learn informative representation from unstructured and structured including time series data using various deep Neural Networks. Watch Huma's full talk here.
14) The New Social Contract - Humanizing Artificial Intelligence
Rana el Kaliouby, Co-Founder and Chief Executive Officer, Affectiva.
AI today has high IQ but no EQ, no emotional intelligence. We’re forging a new kind of partnership with technology. A new social contract that is based on mutual trust. In this talk, Dr. el Kaliouby discusses in the video below, the 5 tenets of this new social contract including how to build AI that has empathy, the ethical considerations of AI and the importance of guarding against data and algorithmic bias. See Rana's talk here.
15) Machine Learning systems Design
Neil Lawrence, Professor of Machine Learning, DeepMind.
Machine learning solutions, in particular those based on deep learning methods, form an underpinning of the current revolution in “artificial intelligence” that has dominated popular press headlines and is having a significant influence on the wider tech agenda. In this talk, Neil gives an overview of where we are now with machine learning solutions, and what challenges we face both in the near and far future. See Neil's full talk here.
16) Predicting What Drives Human Attention in Photographs, Visualizations, and Graphic Designs
Zoya Bylinskii, Research Scientist, Adobe Research
Knowing where a person looks in an image can provide us with important clues about what captures their attention and what may eventually enter their memory. In this talk, Zoya covered both what we know about human attention and how we capture human attention and interest in images at a large data scale using novel crowdsourcing interfaces. Interested in hearing more from Zoya, click here.
17) How Do You Know What A Deep Network Has Learned?
Simon Lucey, Associate Research Professor/Principal Research Scientist, Carnegie Mellon University/Argo AI.
In this discussion, Simon walked us through recent advances his group has made towards making better guarantees over the generalization of deep learning methods for visual tasks where the relative relationship between images is important - most notably object tracking and VSLAM. In particular, Simon discussed a new paradigm for efficient and generalizable object tracking which we refer to as Deep-LK and its extension to 3D PointNet-LK. See the full talk from Simon here.
18) New Optimization Perspectives on Generative Adversarial Networks
Simon Lacoste-Julien, VP Lab Director/Associate Professor SAIT AI Lab/Université de Montréal.
Generative adversarial networks (GANs) form a generative modeling approach known for producing appealing samples, but they are notably difficult to train. In this talk, Simon walks us through how best we can get around these issues and tackle the many objectives set for GAN development. Hear what Simon had to say here.
19) Rewards, Resets, Exploration: Bottlenecks for Scaling Deep RL in Robotics
Shane Gu, Research Scientist, Google Brain
In this talk, Shane focused on three critical components required for massively scaling deep RL in simulation or real-world: rewards, resets, and exploration. Discuss centred around the recent work at Google Brain on the universal reward definitions through natural languages; the concurrent learning of reset policy for safe and continual learning; and the efficient exploration through goal-driven or empowerment-based action abstractions.Hear what Shane and his team at Google Brain have been working on here.
20) A Neural Collaborative Filtering Model for Product Size Recommendations in Retail
Kallirroi Dogani, Machine Learning Scientist, ASOS.
Providing customers with accurate size guidance is one of the main challenges in the online fashion industry. In this talk, Kallirroi discussed recent research we have conducted on the size prediction problem to help customers discover products that are available in their size. Kallirroi went on to explain how through using past purchases we can train a neural network model to learn latent size representations and predict customer sizes. See Kallirroi's full talk here.
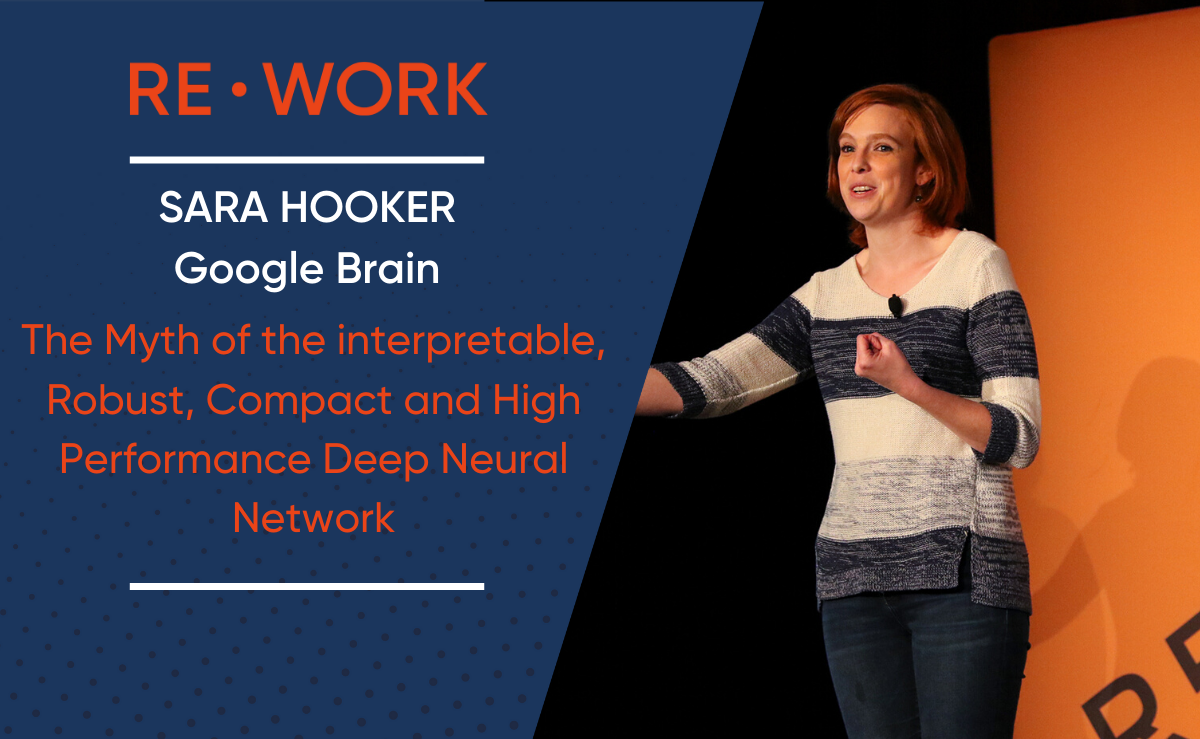
21) Data Products at Scale: Credit Card Fraud Detection for Over 800,000 Businesses
Nevena Francetic, Data Science Lead, Shopify.
It is a long road from having an amazing first model to deploying it in production and maintaining its efficiency over time. Finding extremely rare events in near real time adds additional complexity. In this talk, Nevena spoke how at Shopify, one of the leading e-commerce platforms, are making orders safe to fulfil for over 800,000 merchants operating in 175 different countries. See Nevena's full talk here.
22) POET: Endlessly Generating Increasingly Complex and Diverse Learning Environments and their Solutions through the Paired Open-Ended Trailblazer
Jeff Clune, Senior Research Scientist, Uber AI Labs.
While the history of machine learning so far largely encompasses a series of problems posed by researchers and algorithms that learn their solutions, an important question is whether the problems themselves can be generated by the algorithm at the same time as they are being solved. Such a process would in effect build its own diverse and expanding curricula, and the solutions to problems at various stages would become stepping stones towards solving even more challenging problems later in the process. Hear more from Jeff on the POET algorithm here.
23) Using Deep Learning to Support Customer Operations
Neil Lathia, Machine Learning Lead, Monzo.
In this talk, Neil gave an insight into how Monzo is using deep learning to empower all the customer support, across the bank's various departments. With Monzo becoming incredibly popular in the past two years, we thought it vital that we got some further insight into the behind the scenes workings at Monzo! See more on Neil's talk here.
24) Deep Learning and Cognition - An Overview of 2019 with Yoshua Bengio
Yoshua Bengio, Full Professor, Université de Montréal.
Ongoing research is now suggesting that brains may use a process similar to backpropagation for estimating gradients and new inspiration from cognition suggests how to learn deep representations which disentangle the underlying factors of variation, by allowing agents to intervene in their environment and explore how to control some of its elements. In this hour-long keynote, Yoshua explains where we are, where we have been and what we have to look forward to with Deep Learning. Hear more from Yoshua here.
25) Learning How the Genome Folds in 3D
Neva Durand, Chief Computational Scientist, Baylor College of Medicine.
"The promised revolution in medicine is still yet to come; there is still much we do not know about how DNA regulates cell function. At Aiden lab, we explore how the two-meter long DNA molecule folds inside the cell. Our assay uses proximity ligation to determine which loci in the 1D genome are close together in 3D". Interested in how AI is aiding the advancement of healthcare? Hear Neva explain here.
26) Advancing State-of-the-art Image Recognition with Deep Learning on Hashtags
Yixuan Li, Research Scientist, Facebook AI.
At Facebook everyday hundreds of millions of users interact with billions of visual contents. By understanding what's in an image, our systems can help connect users with the things that matter most to them. To improve the recognition system, Yixuan talked about two main research challenges: how we train models at the scale of billions, and how we improve the reliability of the model prediction. Watch Yixuan's full talk here.
27) Solve for H: Leveraging AI to Solve Problems for Human Kind
Anna Bethke, Head of AI for Social Good, Intel.
Anna's talk gave an overview of several Solve for H projects at Intel. The use cases of AI is limitless, the trick is determining how to use or modify existing algorithms and systems in such a way that they truly aid the end users. Even more so than typical AI systems, it is important to ensure that user’s want and trust an algorithm’s aid. Anna went on to highlight the steps necessary for creating AI for social good projects and various ways to become involved in a growing community around this type of work. See Anna's full presentation here.
28) Human and Artificial Intelligence in Healthcare
Maithra Raghu, Research Scientist, Google Brain/Cornell University.
In this talk, Maithra outlined one such problem, that of medical expert disagreement. We study the application of machine learning to predict patient cases which are likely to give rise to maximal expert disagreement. Watch Maithra's full talk including Q&A here.
29) Collaboratively Building Trust in AI
Ashley Casovan, Executive Director, AI Global.
There are several good reasons why there's increasing wariness and a limited degree of trust in the use and adoption of AI, especially in the public sector. We've all read the news stories about predictive policing, the use of affective computing in the education systems, and inaccurate health diagnosis made by automated systems. In this presentation Ashley highlighted work underway to build the practical tools needed to support the responsible implementation of AI in the public sector and beyond. See Ashley's full discussion here.
30) Building Generative Models of Symptomatic Health Data for Autonomous Deep Space Missions
Krittika D'Silva, AI Researcher, NASA.
Last but certainly not least, Krittika D'Silva spoke on her work at NASA FDL in which she examined how AI can be used to support medical care in space. Future NASA deep space missions will require advanced medical capabilities, including continuous monitoring of astronaut vital signs to ensure optimal crew health. See Krittika's full talk here.
We hope that you have found the above videos useful and informative. We regularly release summit content from industry leaders on our youtube channel, which you can see here. Interested in attending a future summit? You can see our full listings for 2020 here.
Thank you for your support in 2019 and we hope to see you next year.
The RE•WORK Team.
Top AI Resources
Experts Predict The Next Roadblocks in AI
Experts Predict The Next AI Hub
The AI Overview - 5 Influential Presentations Q3 2020
13 ‘Must-Read’ Papers from AI Experts
‘Must-Read’ AI Papers Suggested by Experts - Pt 2
10 Must-Read AI Books in 2020
10 Must-Read AI Books in 2020 - Part 2
Change Detection and ATR using Similarity Search in Satellites
Top AI Resources - Directory for Remote Learning
Top AI & Data Science Podcasts
30 Influential Women Advancing AI in 2019
30 Influential AI Presentations from 2019
AI Across the World: Top 10 Cities in AI 2020
Female Pioneers in Computer Science You May Not Know
Top Women in AI 2020 - Texas Edition
2020 University/College Rankings - Computer Science, Engineering & Technology
How Netflix uses AI to Predict Your Next Series Binge - 2020
Top 5 Technical AI Presentation Videos from January 2020
20 Free AI Courses & eBooks
5 Applications of GANs - Video Presentations You Need To See
250+ Directory of Influential Women Advancing AI in 2020
The Isolation Insight - Top 50 AI Articles, Papers & Videos from Q1
Reinforcement Learning 101 - Experts Explain
The 5 Most in Demand Programming Languages in 2020
Generative Models - Top Videos & New Papers
Applying AI in Clinical Development of Drugs
What is AI Assurance?