Training computer vision models require a constant feed of large and accurately labeled datasets. However, this typically requires large time and capital commitments, especially since most of the labeling and quality assurance is done manually by humans. Can most, if not all, of this workflow be automated intelligently?
Last week, Superb AI's CEO, Hyun Ki joined the MLOps Summit discussing Superb AI uses advanced ML techniques like transfer and few-shot learning to help teams automate the labeling and auditing of computer vision datasets. You can see Hyun's full presentation on our Video Library here.
What will you learn?
- Data labeling is a big bottleneck for teams, in both time and cost
- Labeling automation isn't of much value on it's own if auditing is not intelligently automated as well
- Even with automation, teams need to craft precise and repeatable workflows around data preparation and data-ops
What questions do you have for Hyun and the Superb AI team?
Watch a preview 👇
The concept behind their custom Auto-Label is simple: instead of having to create massive ground truth datasets by hand, teams can now build much smaller ground truth or “golden” sets, quickly spin up and train an auto-labeling model with a few clicks and label large datasets in a short timeframe. Coupling the workflow with our proprietary Uncertain Estimation AI and management tools, teams can immediately identify hard labels, build active learning workflows for auditing and deliver datasets in a matter of days.
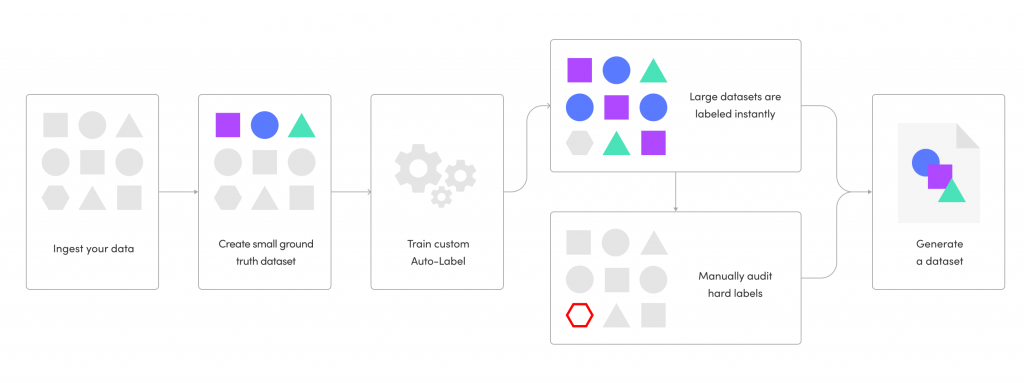
Read about their advanced Transfer Learning Auto-Label here.
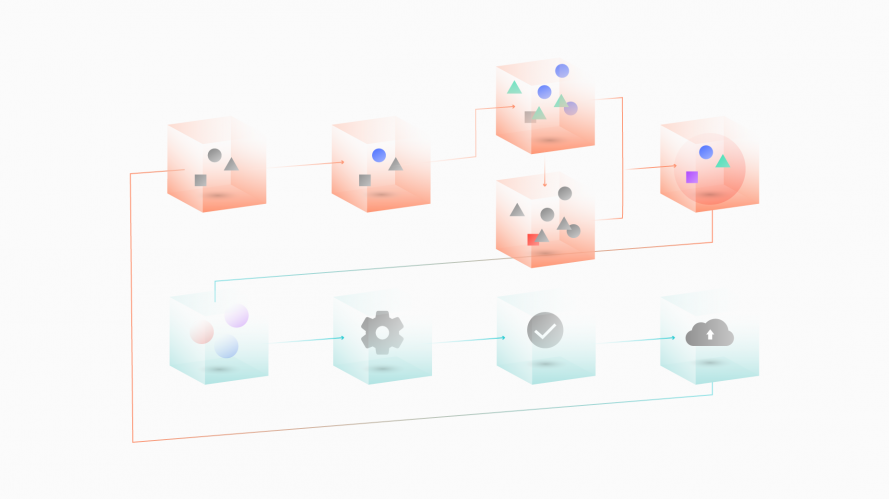
📌 Further reading: You can also read more about their End-to-End Solution for Developing Computer Vision Applications here.
➡️ Recap the MLOps Summit from last week on the Video Library here!