We're nearly at the halfway point of 2021, and we have seen some great developments utilizing AI in the Financial sector. We’ve handpicked our top videos from the AI Library which share the big advancements in finance using AI, NLP, Machine Learning, and more from the biggest financial institutions, including JPMorgan Chase, Vanguard, Scotiabank & Nasdaq.
Alongside the video presentations below, we've included chapter timestamps so you can jump to the key takeaways. Enjoy!
AI Beyond Pattern Recognition: Decision Making Systems
Kevin Kim, Data Scientist, Nasdaq
While Machine Learning and AI technologies are now advanced enough to outperform humans in a variety of tasks, how we make decisions with models varies by practitioner. Reinforcement Learning is promising but it is limited to adversarial settings; or, in vernacular, situations where decisions directly impact the environment. Without figuring out how AI systems can make good decisions in environments they cannot influence, we may forever be stuck in a limbo of pattern recognition, prediction, and analytics. What if we can develop a “theory” of AI decision making? Can we view different decision-making situations as a set of engineering systems? Can we define the key components of an AI decision-maker? Kevin answers these questions and more in his talk below.
Key Takeaways:
1) AI systems are far more valuable in making decisions than making simple predictions and pattern recognitions.
2) We need a “theory of design” for AI decision-making systems: For AI to become a trusted part of decision-making both in and out of the industry, we need to understand and generalize components of AI systems and define what it means to be “robust”.
3) This starts with looking at actual use cases, identifying similarities, and quantifying key parameters – so we may use standard design techniques to design AI systems.
Video Chapters:
- 00:00 - Use cases, decision-makers and human beings
- 04:01 - Data scientist, benefit and designer
- 05:43 - Reinforcement learning, perception and model
- 08:26 - Trait, constraint and rationality
- 10:44 - Portfolio optimization, index and variance
- 14:19 - Finance, poker and hierarchy
- 18:25 - Models, futures and wrong way of implementing
The Future of AI Research in Finance
Manuela Veloso, Head of AI Research, JPMorgan Chase & Co.
Manuela's talk covered her work at JPMorgan, including projects utilizing Natural Language Processing (NLP), Speech Recognition, Computer Vision and more. During her talk, Manuela explained how JPMorgan predicts and effect economic systems, how to liberate the data, how to eradicate financial crime, and then also how to empower employees, protect life experience, and eventually also help with policy compliance.
Key Topics Covered:
- AI to support human decision through NN-based image classification
- Mondrian-C, Mondrian-P, Mondrian-V, Mondrian-A
- Multiagent Systems
- Agent-based simulation; learning agents & more!
Video Chapters:
- 00:00 - Domain, component and finance
- 01:45 - Image classification (reasoning through images, Mondrian models, event simulation & more)
- 11:02 - Summary and project
- 13:28 - Advertisement
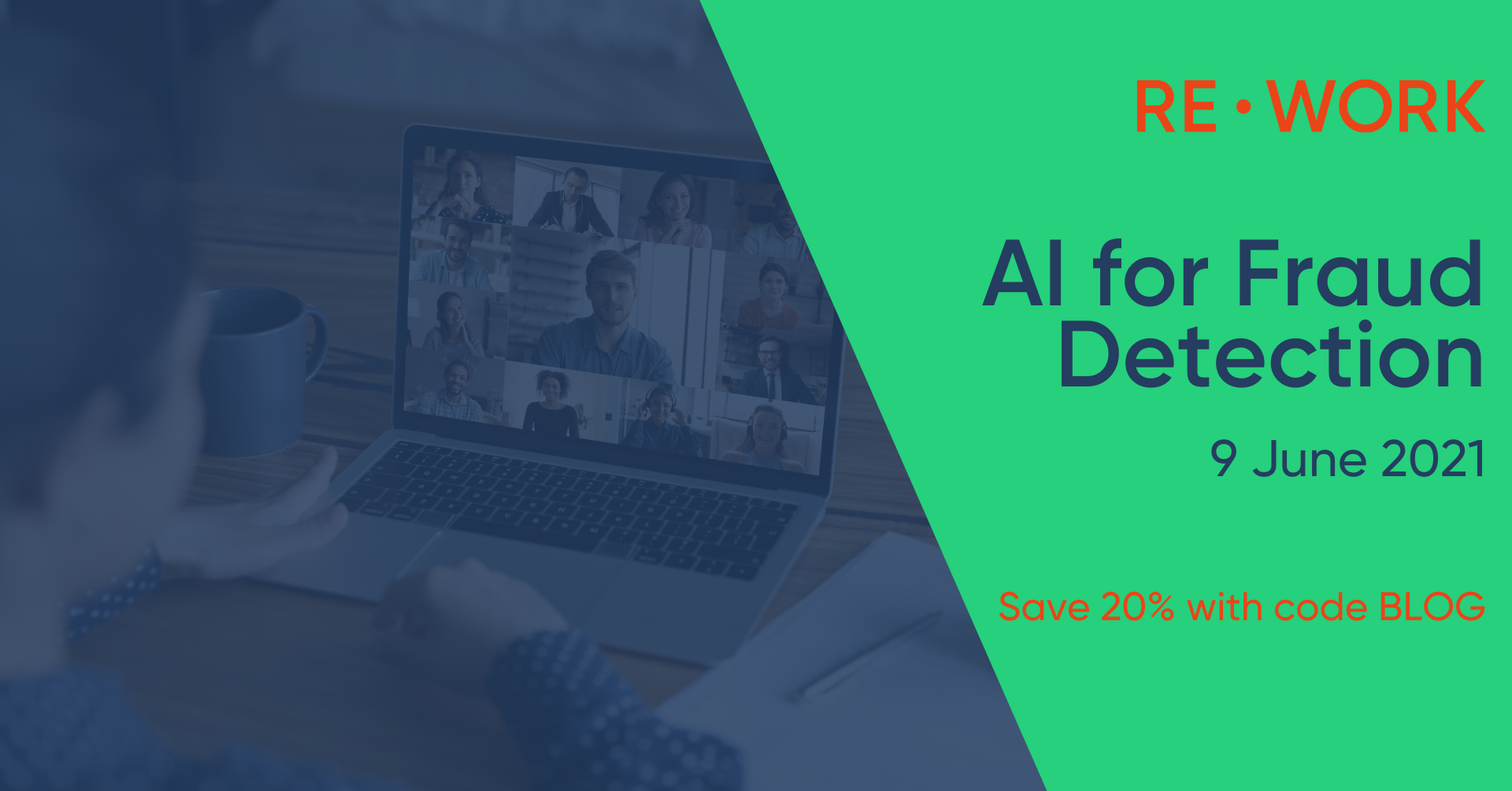
Fund2Vec: Mutual Funds Similarity Using Graph Learning (cc)
Dr Dhagash Mehta, Machine Learning and Asset Allocation, Vanguard
We were delighted to be joined by Dhagash Mehta from Vanguard to discuss how those working in the industry can identify mutual funds, including exchange-traded funds. In this presentation, Dhagash proposes a radically new approach based on the weighted bipartite network representation of funds and their underlying assets data using a sophisticated machine learning method called Node2Vec to learn an embedded low-dimensional representation of the network.
Key Takeaways:
1) Machine Learning for mutual funds analytics (sales, marketing, portfolio diversification, etc.)
2) An interesting use case for the application of graph Machine Learning in the investment management industry.
3) Technical details on a specific graph machine learning algorithm called Node2Vec.
Video Chapters:
- 00:00 - Mutual funds, portfolio and risk-return
- 07:35 - Categorization, linear relationship and Euclidean distance
- 13:47 - Asset, equity fund and raw data
- 19:15 - Node, nearest neighbor and similarity
- 22:47 - Machine learning, network and representation
Identifying and Addressing Bias in Machine Learning Models Used in Banking (cc)
Kishore Karra, Executive Director, Model Review Group at JP Morgan Chase
Banks are increasingly relying on Machine Learning models as decision support systems in various areas such as fraud detection, credit scoring, and optimal order execution. When a model makes a decision on a client application, it is important to ensure that the decision is unbiased and explainable, both from a regulatory and moral standpoint. In this talk, Kishore focussed on relevant regulations and some of the ways in which these biases can be identified and addressed.
Key Takeaways:
1) The types of applications of ML models where ‘biases’ could influence model decisions.
2) Applicable regulations in the banking domain.
3) Some statistical techniques that will help identify the biases and possible course of actions to address the biases.
Video Chapters:
- 00:00 - Algorithm, support system and outcome
- 01:51 - Applicant, income and behaviour
- 04:12 - Model, subset and output
- 05:16 - Regulator, regulation and disparity
- 13:10 - Potential Solutions to Use - Disparate Impact Analysis etc
- 13:39 - Datum, delay and shop
Transformation of Core Business Function Areas Using Advanced Analytics and AI (cc)
Dr. Nitesh Soni, Director - Advanced Analytics & AI, Scotiabank
Financial institutions have already started to embrace AI as a part of their core business strategy. The AI investments are not only focused on enhancing the customer and risk controls analytics but also to transform and modernize their core business function areas. The AI technologies (Automation, ML, NLP, Deep Learning etc) combined with the unprecedented amount of data are helping to increase the operational efficiencies and reduction in cost while mitigating Risk and Compliance issues by creating timely awareness and preventative measures. In this talk, Nitesh touches on the challenges to establish the AI framework i.e. how to start a journey from foundational analytics to advanced analytics; processes and methodologies to identify and prioritize the business problems that can be solved and scale quickly across business function areas.
Key Takeaways:
1) Modernization of Business Core Functions is a must and should be a part of overall corporate strategy.
2) A variety of AI technologies are been leveraged to achieve this.
3) AI solutions are bringing Gain in operational efficiencies and mitigation in operation and reputational risks.
Video Chapters:
- 00:00 - Sustainable solutions, Technology and bank
- 07:33 - VPN use
- 09:11 - Network failure, vulnerability and remediation
- 13:22 - Email, alert and complaint
Interested in watching more video presentations from AI experts in various industries including Finance, Healthcare, Insurance, Retail and more? The RE•WORK AI Library has 500+ hours of content from over 1,500 AI experts. You can get a free trial here and browse any video you'd like!
Are you interested in reading more AI content from RE•WORK and our AI experts? Read these articles below:
- Girl Decoded and Emotion AI - Rana el Kaliouby
- How Chatbots Fail & Conversational AI Supersedes 2020
- Starting a Career in AI and Giving Back to the Community - Diana Murgulet
- An Introduction to Federated Learning
- AI Experts Discuss The Possibility of Another AI Winter
- 20+ Pieces Of Advice From AI Experts To Those Starting Out In The Field
- How Has COVID-19 Accelerated Digital Transformation? - Claire Calmejane
- How can Startups & Enterprise Leverage Each Other? - Shaloo Garg
- How to Overcome Main Challenges in Implementing AI in the Insurance Industry and Improve Claims Management Process
- Why AI Ethics Matter - Kay Firth-Butterfield, Head of AI and ML at WEF
- AI in Loyalty & Subscription Products - Emily Bailey