We're joined for a second time on our Women in AI Podcast Series by Sara Hooker, Researcher at Google Brain, who spoke with us at the Deep Learning Summit in San Francisco. The discussion focused on ’What Does a Pruned Neural Network ‘Forget’'.
Sara’s main interests gravitate towards interpretability, predictive uncertainty, model compression and security.
Topics explored in the interview include:
- Sara’s Current Work at Google Brain
- The Results From Research on Brain Damage
- Accra Lab in Ghana, by Google
- Inspiring Women in AI
- Diversity and Inclusion in AI
- Advice for Those Starting a Non-Traditional Career Path Into Research
- How to Deal With Imposter Syndrome and Survivorship Bias
🎧 Listen to the podcast here.
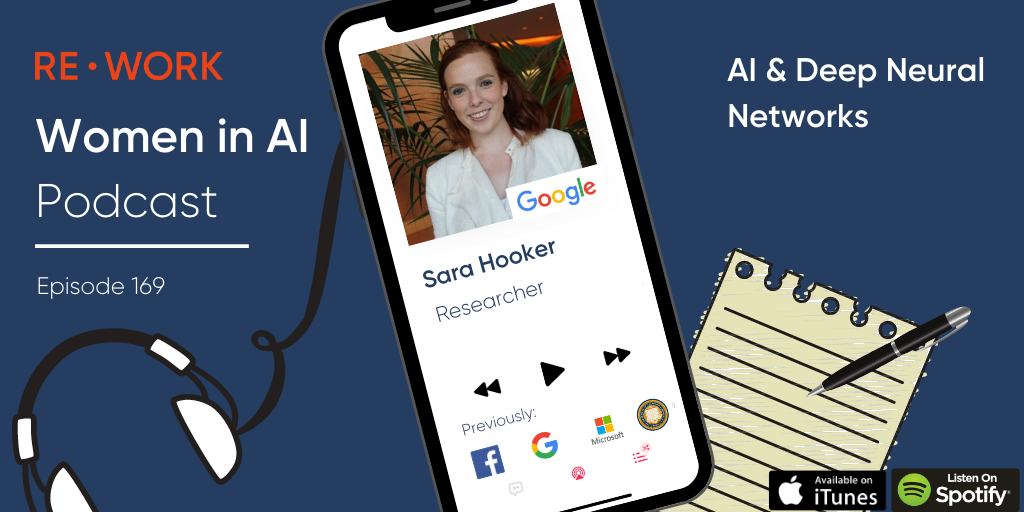
Can you just tell us a little bit more about yourself?
Absolutely. So I am a Researcher at Google Brain. My research interests tend to be like, how do we train models that fulfil criteria beyond just tested accuracy. So how do we think about models and not just high performance, but maybe interpretable, or fair, or be compact so that we can deploy them to resource-constrained environments? And in parallel, like a lot of what I've been working on is how do we build technical capacity around the world. And how do we bridge the entry gap for people who want to come into machine learning and who want to do the research? So that's meant that I travel quite a bit last year, but I also get to teach fairly often, which is a part of my job that I really enjoy.
You recently presented about your recent work about selective brain damage. Can you tell us a little bit more about the direction of this research?
Oh, selective brain damage. So I just give a talk about that, selective brain damage is a very snazzy title. But really, it's about like, how do we measure the trade-off of like compressing a model to different degrees of sparsity? And does this have implications for other things that we may care about? So in many ways, like we biologically have this trade-off, where we prune a lot of our neurons over the course of early childhood, adolescence, and then into adulthood, and between ages 2 and 10, we lose 50% of our neurons. It is a wow. But at the same time, like we, this is largely invisible, like we don't actually notice any change to our behavioural cognitive ability. And in many ways, this suggests that we're measuring what is reweighted, in a way, that's not precise enough. And so we asked us for deep neural networks, can we understand why deep neural network forgets, as we vary capacity? We asked what examples and classes are more impacted, and it turns out that the classes and examples impacted, are more challenging from auto and even more challenging for human if you show that subset of images to a human. So it's really interesting and poses a lot of additional like research questions. Like, can we use this as a way to surface challenging examples to domain experts, so they can have an understanding of what the trade-offs are when they deploy a compressed model to like a resource-constrained environment.
Your work has also seen you head out to the new Accra Lab in Ghana, which I believe you were preparing for during your last talk here. And can you tell us a little bit more about your time there?
So I think we last spoke with RE•WORK, I think it was a year before last maybe. Okay, so that was when the new Accra Lab was starting. And Accra Lab is really special because it's the first research lab that Google has started in Africa in the African continent. And it is really exciting, it is still early stages but I spent a lot of last year in our craft, with the researchers who are building out the lab. And the focus of the lab has really been on this combination of pure research. But also, how do we connect research with applied problems? So how do we work with family imagery? How do we work with the classification of crop disease? And I think this is also an important move for Google Brain. Because as a pure research lab, we have tended to be focusing on pushing out the field of research in terms of these theoretical advances. But we also have this kind of very exciting position where we can tackle really big problems that have implications for society. And that's what this lab has really shown that it will focus on. And Accra is just a beautiful city. So the lab has been built out currently, it's, I believe, like nine researchers, but growing this year again. So that's really exciting.
Can you tell us of any current inspirations of yours or any colleagues, industry personnel that we should be watching the work of?
This is a good question, I think as many. So it depends on, really the area of interest, but I think a few people that immediately come to mind Andrea Frome, she's working on disinformation at Facebook, how can we better target and identify like disinformation posts. There is Catherine Ruff, who's working on healthcare in AI. There is a whole host of Uber AI early-career researchers like Hattie and Jane Hung, and some more senior researchers like Roseanne. There is also amazing researchers who are in Nigeria and doing very interesting work on like, how do we create better-tailored datasets. So the list goes on and on and on, I actually think like, the big thing we should do is just have better directories for like many of these groups, because it often feels like because in some of these contexts, like women are more working in silos. Like silos have not been able to connect with other female researchers. Some of those communities are missing. But there's a lot of amazing work being done by women right now.
What's your advice for following a non-traditional career path into research?
I think the reason that question was asked is that my path has been a little bit atypical. So I started out working as an economist and I largely taught myself, machine learning, mainly driven by I think, at the time, I was just really excited to work on problems with different nonprofits. And I was working with all these different nonprofits with both engineers, as well as researchers. And I started to become excited, like, I want to learn this toolkit. And I think that, in some ways, like my story is one of perhaps like brute force. I just decided, I'm really passionate about this thing, and I'm going to start working towards it.
I think that my advice for other researchers or people who want to potentially go into the field, there are a few things. One is that, have a clear sense of what you want to achieve. I actually don't think that doing research for Google Brain is the only finish line. I think, in fact, there are many possible ways to engage in machine learning. And I did both, applied machine learning and before I went to Google Brain but I am now doing pure research. And I think that getting a taste of both is also important in calibrating what you are passionate about because the two workflows are actually quite different. I think the other thing is, is to try and find communities outside of your individual like efforts to grow because what I've seen a lot particularly in the communities where you are isolated in the sense that there's not like a classroom where you're really self-teaching yourself. It's quite lonely because you're really pushing yourself every day. And so finding both mentors as well as like some type of either virtual community, or even creating your own Meetup group is really important to stay focused, but also to get some type of accountability. Like as humans, we're very social creatures, which means that like a lot of how we motivate ourselves towards goals is not just like on transit passion, but also the accountability that we feel from doing things and achieving things with others.
And I think the big thing, if I would say, I've kind of mentioned a few things now, which is, sample different types of projects, find a community. But the last one is work on an applied project, that even if you're not working in a startup, you should just craft your own project to work on. Like, for me, I was working a lot with nonprofits because I started a nonprofit six years ago, and I was working with many different nonprofits in different countries doing machine learning. And that by far was like the biggest driver in me understanding that this was something I enjoy, as well as like giving me tangible skills, and making it easier to measure progress, because one of the things that are very overwhelming about coming in as a beginner is just the amount of resources available, and almost the information overload of what video do I look at and what paper do I read and by giving yourself a task or like a focused angle, you're much more likely to be able to measure incremental progress, which is also very good for people who are new to the field. You need to feel like you're achieving something incremental every day. So those are the few the main things.
But also, I would finally say like, don't be too hard on yourself. I think it's really hard. I think that people who try to engage with new material, honestly, are very brave people, because you're motivated at first by curiosity. And first is like no reward besides your curiosity. And I think that there's something that that is very, both exciting about that. But as well can feel quite lonely and quite like disheartening sometimes, and you have to almost persevere through it's like learning a language, you have to persevere through learning like odd phrases until you know how to link sentences together. And sometimes that takes quite a bit of time. And a lot of people get stuck on the words before they make it to the phrases. But the words are brute force like most people learn a language initially by just repeating enough iterations and spending enough time on it, they finally get to the phrases, and that's where it gets fun.
One topic, which comes up a lot, particularly for new people to research is feeling like an imposter or being sensitive to survivorship bias? And can you explain these terms and any advice on how to handle them?
Imposter syndrome is this idea really, that you don't have the qualifications or criteria for the role that you're doing, so you often don't feel like you belong. Or you question whether you belong, and survivorship biases tend to feed into imposter syndrome in the sense that like, you only see what people are achieving and not necessarily failures. I think part of addressing the lack of a sense of belonging is honestly difficult because it honestly stems from feeling vulnerable and different parts of your career, about different parts of your identity. I think partly like how I've seen, at least for myself, like what has helped is being part of multiple communities.
So yes, I'm doing research at Google Brain but sometimes I feel very insecure about whether I'm as good as my colleagues, and I think that's a natural part of the process. But I also complement that with teaching. And if I'm not feeling like I'm the most amazing researcher, I'm also teaching and getting this experience that I'm achieving something with my students. It's also important to think about why imposter syndrome tends to be trickier in research, which is that, in research has not many good feedback loops of what your value is. And that's for a few reasons. When I was working in applied machine learning, a lot of what you do is you deploy algorithms, so you get an instant feedback loop of really how the metrics have changed in response to your contribution. I think in research, it's harder to get that clean feedback loop because often you're working on a project over multiple months. And in fact, like when you do finally publish a project, few research ideas are truly ground-breaking.
I mean, for a good reason is that like most research builds towards your ground-breaking idea. But most research then tends to be in the middle. And when it's in the middle, it depends a lot on whether other people perceive it to be valuable as moving towards that ground-breaking idea. And that can be hard because it can lead to things like imposter syndrome because you're not getting the clean daily feedback, you're going for long stretches of time without any signalling about whether you belong, your work is valuable. And so really, for researchers, I think the challenge is to be engaged in multiple workflows, where they are getting more signalling. So complementing their daily research with teaching, or complementing their daily research with contributing via mentoring, start-up, or someone who needs technical expertise.
It's also very critical spaces, finding safe spaces where they have communities, I think that at different stages of our career, we need different communities more. And that's largely because at different stages to create this, there are things that surface more insecurities like people going to Europe for the first time, who are attending the conference are probably insecure about what to event expect. Like what are they going to be questioned if somebody's going to say, you know, how many years of machine learning research, and these are questions that are very much on the mind? And so for those people, it's very important that there is a community of fellow newcomers where they can connect, but also for people who are coming from entirely different geographies. Even for something like applying for an internship at like Google or another research lab, it's often very intimidating, because it's not even sure what is expected after the application process. And a lot of that, like, you know, we talked about the person addressing imposter syndrome, but it's also on the company and on the organisation and on the community.
The way to address it is to just make it transparent what are the expectations because a lot of imposter syndrome is this combined effect of not feeling what you belong and not knowing what success looks like. And if we can be more transparent about what success looks like, we really have better tools to then put people to measure where they're making progress towards success. So what that looks like, for example, for people who are coming in and who are not sure what a process looks like, it's being transparent about what it is to look for, how you can build skills towards certain requirements, how to prepare your CV, like if someone's from a different cultural background. And these touchpoints, I do think help, but also be more transparent about our failures, like ultimately. I think the best conversations I've had with senior researchers are those who have been very transparent about times they have failed quite badly. And it's for good reasons, perhaps, that these conversations are never public because maybe they also feel like they don't want to reveal these things publicly. But there is an excellent series on How I Fail, I believe it's called. And there's also an excellent blog post about Failure. I believe the author is Charles Sutton and Veronika. But both of these are like great resources for talking about failure and for making it more acceptable for us to share our failures as a community, which is the final step as well.
Further reading - Experts Blogs & More
Female Pioneers in Computer Science You May Not Know - Part 2
AI Experts Talk Roadblocks in AI
Experts Predict The Next AI Hub
The AI Overview - 5 Influential Presentations Q3 2020
13 ‘Must-Read’ Papers from AI Experts
‘Must-Read’ AI Papers Suggested by Experts - Pt 2
10 Must-Read AI Books in 2020 - Part 2
Change Detection and ATR using Similarity Search in Satellites
Top AI Resources - Directory for Remote Learning
Top AI & Data Science Podcasts
30 Influential Women Advancing AI in 2019
30 Influential AI Presentations from 2019
AI Across the World: Top 10 Cities in AI 2020
Female Pioneers in Computer Science You May Not Know
Top Women in AI 2020 - Texas Edition
2020 University/College Rankings - Computer Science, Engineering & Technology