Who’s the ruler of this new decade? AI. Every industry, company, and consumer is impacted by AI. For businesses, AI will prove to be the biggest competitive advantage. One in ten enterprises currently use ten or more AI applications and 75% of businesses are expected to shift from piloting to operationalizing AI by 2024. AI has the potential for productive, efficient, and innovative outcomes, but it comes with risks. As adoption advances, data science, AI/ML teams, and business leaders should widen the scope of focus to the broader implications of AI systems: are they trustworthy, transparent, and responsible? Are outcomes reliable over time? Is there bias built into models? Are models standing up to regulatory and compliance requirements?
What are the potential risks with AI?
We’ve seen multiple examples in the past few years of alleged bias in AI. In 2019, it was the Apple Card/Goldman Sachs issue. What started as a tweet thread with multiple reports of alleged bias, eventually led to a regulator opening an investigation into Goldman Sachs and their algorithm-prediction practices. And this isn’t an isolated instance: Amazon’s biased hiring algorithm, racial bias in healthcare algorithms, and bias in AI for judicial decisions are just a few more examples of rampant and hidden bias in AI algorithms.
In the credit card example above, this issue could have been avoided if humans had visibility into every stage of the AI lifecycle. In the model validation stage, teams could have unearthed instances of unwanted model behaviour. With visibility into production systems, humans-in-the-loop could have uncovered production model issues and quickly solved them to stop issues from spiralling out of control.
For each high-profile case that comes under public scrutiny, how many are silently operating and negatively impacting lives? One of the main problems with AI today is that issues are detected after-the-fact, usually when people have already been impacted. This is a foundational problem in AI that needs a foundational fix.
What is Responsible AI?
Responsible AI is the practice of building AI that is transparent, accountable, ethical, and reliable. When AI is developed responsibly, stakeholders have insight into how decisions are made by the AI system, the system is governable and auditable through human oversight. As a result, outcomes are fair to its end users, stakeholders have visibility into AI post deployment, and the AI system continuously performs as expected in production i.e. fairness is maintained and models are high-performant.
Diving into the 4 key principles: Transparency ensures that stakeholders have visibility into what the AI system is doing. They understand the ‘why’ behind decisions and the predictions they make and build systems with privacy and security built-in. Accountability provides much-needed checks and balances for AI systems with guardrails, guidelines, and governance frameworks in place. Most importantly there is human oversight and the ability to override decisions where needed. Ethics broadly refers to ensuring that AI is doing well by all impacted with a focus on fairness and inclusion. Reliability is one of the most important principles because it is what ensures that responsible models are maintained over time, continuously.
How Explainable AI enables teams to build Responsible AI
Complex AI algorithms today are black-boxes; while they can work well, their inner workings are unknown and unexplainable. Explainable AI works to make these AI black-boxes more like AI glass-boxes. Explainability is the most effective way to ensure AI solutions are transparent, accountable, responsible, fair, and ethical across use cases and industries. To address the foundational issues I discussed above that contribute to ‘irresponsible AI’, explainability needs to be a fundamental part of any AI solution, from model validation to production model monitoring. Teams need visibility into the inner workings of models throughout the lifecycle of AI.
Explainable AI in the AI Lifecycle
To better understand Explainable AI, let’s consider a couple of use cases in the AI lifecycle to see how XAI is beneficial. I’ll use a credit lending example to walk through this.
A credit lending agency wants to build an AI model to generate credit decisions for their customers. These decisions could range anywhere from increasing credit card limits to selling new credit products. In order to build this model, the firm uses existing historical data they have access to – data built over their many years of providing credit products to their customers. The firm uses this data to train and build a credit lending AI model.
Once they build the model, they use explanations to validate the model. Before moving the model to production, validation helps teams quality-check the model. What sorts of credit predictions is the model making? The model creators can access individual explanations for each prediction to better understand what influenced that prediction. For example, it could be that the annual income of an applicant influenced the AI model’s prediction by 20%. What potential does this have for negative outcomes in production models?
Explainable AI works for production models similarly. The data encountered in production models might be different from training data, and explanations provide a way to solve critical issues. Consider the case of explainable monitoring: when a model is in production it needs continuous monitoring to watch out for operational challenges like data drift, data integrity, model decay, outliers, and bias. With explainability, teams get information showing them the ‘why’ behind these operational challenges like data drift or outliers, so they can solve them.
In a recent survey conducted by Fiddler, when respondents were asked how important it is to be able to explain models, the majority, at 75%, said that the ability to do so is mission-critical:
Model Performance Management powered by Explainable AI
Explainable AI is the future of business decision-making. It plays a role in every aspect of AI solutions from training, QA, deployment, predictions, testing, monitoring, and debugging.
In short, Explainable AI plays a critical role in Model Performance Management (MPM).
Building responsible AI means model builders, validators, and extended team members pay attention to some of the potential risks to consider like:
- Design and interpretation: Is the model serving its intended purpose? Are the people interpreting the model’s results aware of any assumptions made by the people who designed the model?
- Data: Do the data sources for the model meet privacy regulations? Are they inclusive? Are there any data quality issues?
- Monitoring and incident response: Do the model’s predictions continue to perform well in production? How does the model perform differently in production? How do we respond when there is a failure?
- Transparency and bias: Are the model’s decisions explainable to a compliance or regulatory body? Have we ensured that the model is not inherently biased against certain groups of people?
- Governance: Who is responsible for the model? Who is held accountable for errors in the model? Is the model operating according to industry standard regulation?
To get answers to these questions and more, XAI will become a prerequisite for deploying any AI solution in business. Explainable AI enables businesses to build trustworthy, ethical, and responsible AI solutions.
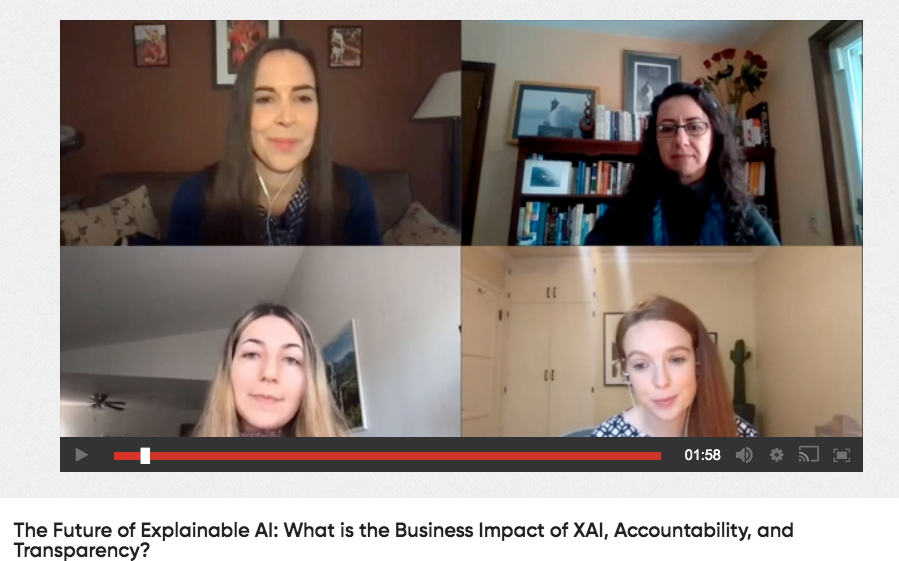
Author Bio:
Anusha Sethuraman is a technology product marketing executive with over 12 years of experience across various startups and big-tech companies like New Relic, Xamarin, and Microsoft. She’s taken multiple new B2B products to market successfully with a focus on storytelling and thought leadership. She’s passionate about AI ethics and building AI responsibly, and works with organizations like ForHumanity and Women in AI Ethics to help build better AI auditing systems. She’s currently at Fiddler AI, a Model Performance Management and Explainable AI startup, as VP of Marketing.