"I am looking forward to sharing my unique experiences working as a Data Scientist in a male-dominated world and valuable lessons that I learned in a workplace and that are not taught in any higher education institution, in particular, for those of us who have graduated with STEM degrees.", says Nataliya. "I would like to highlight some of the challenging situations that I found myself in when working as a Lead Data Scientist and the only woman on the team at the same time."
Ahead of the RE•WORK - Women in AI Reception - Toronto, we asked Nataliya Portman, Senior Data Scientist at Cineplex Digital Media to share her thoughts on the topic. Here's what she had to say:
What was the biggest challenge you faced in your journey to your current role and how did you overcome them?
"The biggest challenge for me was to continue practicing my expertise in Data Science in industry after my 1 year contract role as a Data Scientist at a biotech startup ended. Even with my one year of Data Science experience working in industry that I added with such pride to my resume (back in 2015), my overall work experience was heavily outweighed by academia. My resume did not look so appealing to employers, and I felt that they were looking for a very specific experience, like geospatial or GPS data analysis, for example.
During my first interview with a company looking for these specific skills, I had to be creative in how I relate my academic experience working with three-dimensional brain MRI data to the GPS kind. And I tried to persuade them that a 3D human brain MRI also has a stereotaxic coordinate system for referencing spatial locations of brain structures, similar to the Earth with its spherical GPS coordinates that measure locations. And I claimed that semi-supervised and unsupervised ML methods I used for 3D brain segmentation carry over to the analysis of geospatial data. Well, I did not get that job. And I decided to upskill my knowledge of statistics while actively searching for a job in industry (any sector!) and I took a challenging Statistics in Medicine online course from a well-known Stanford University. A course from Stanford really made my resume stand out! I used hired.com job search platform at that time, and their resume consultant highlighted this piece of my most recent education bringing attention to potential employers.
But my resume was still heavy on academic accomplishments, and no wonder that it attracted an employer from academia. Since Deep Learning methods and big data technologies were on the rise at that time, I decided to take a DL researcher job offer from Ontario Tech University in Oshawa. It was not a job in industry that I wanted, but it gave me a great opportunity to delve into the world of convolutional Neural Networks and its implementation challenges, specifically, parallel data processing technologies (for example, open source Python libraries like PySpark) for the preparation of training datasets and DL model training on a Blue Gene IBM supercomputer. And when I was 10 months into this researcher role, I came across a Data Scientist job opening at TradeRev, a startup in the automotive industry that offers an innovative way to sell fleets of used cars through auctioning using TradeReb mobile app.
I actually wrote a cover letter expressing a genuine interest in this opportunity and I sent it by mail to the CEO of the company along with my resume. And they really liked this gesture and invited me for an interview. I was not really hoping to get that job, I was very relaxed when I arrived. The interview lasted for two hours, I met with all important decision-makers and they offered me a job the next day. This is when my real Data Scientist journey began! I had to start from scratch to build an AI-powered mobile app that would recognize vehicle views and damages while walking around that vehicle and automatically take their photos to supplement a vehicle condition report."
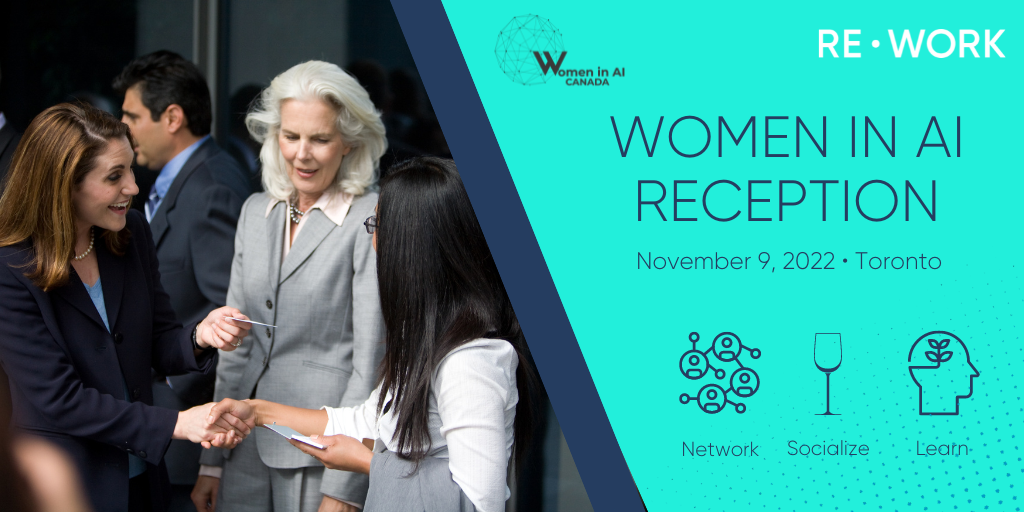
What are some wins from a completed project you worked on? What challenges did you face during it? How did you overcome them?
"As soon as the first version of a so-called Autovision mobile app was created and went into hands of our testers, and the Marketing team advertised this product as an innovative AI-powered feature that automatically generates a car condition report, the company shares went up in value. That was a big win, as we started with a vision for this product, having no training data or experience building a Deep Learning pipeline on Google Cloud Platform. It was a long-term project that lasted for two years, and it was a colossal amount of work figuring out the data collection protocol for data augmentation purposes, building the PoC model and persuading the leadership that it yields accurate and reliable predictions, building the pipeline for training data preparation that leverages photos of vehicle views accumulated over years to enhance the performance of DL model (a lot of manual work went into the verification of picture labels).
Another example is building a time-series forecasting model that makes predictions of product sales based on the selected promotional campaigns and calculates lift in revenue associated with each campaign. In this way, the clients were able to gain an insight into the near future and to select the most profitable campaign. And the prediction accuracy of the sales forecasting model matters a lot as a business has to adjust the annual budget or go-to-market strategy based on the monthly estimates of revenue from sales!
When I started working at 360insights, the company was about to lose part of a business with an automotive industry client in the US due to highly inaccurate overly estimated revenue forecasts that the Data Analytics and Insights team was delivering. I was able to win the client’s trust by introducing an experimental approach for the discovery of methodology that yields the most accurate results. In about three months, we were able to develop and to validate a trustworthy forecasting model. And during these three months, I established a weekly reporting cadence with the client showing him the results of our exploratory analysis and outlining the next steps and thus rebuilding the client’s trust in our Insights services."
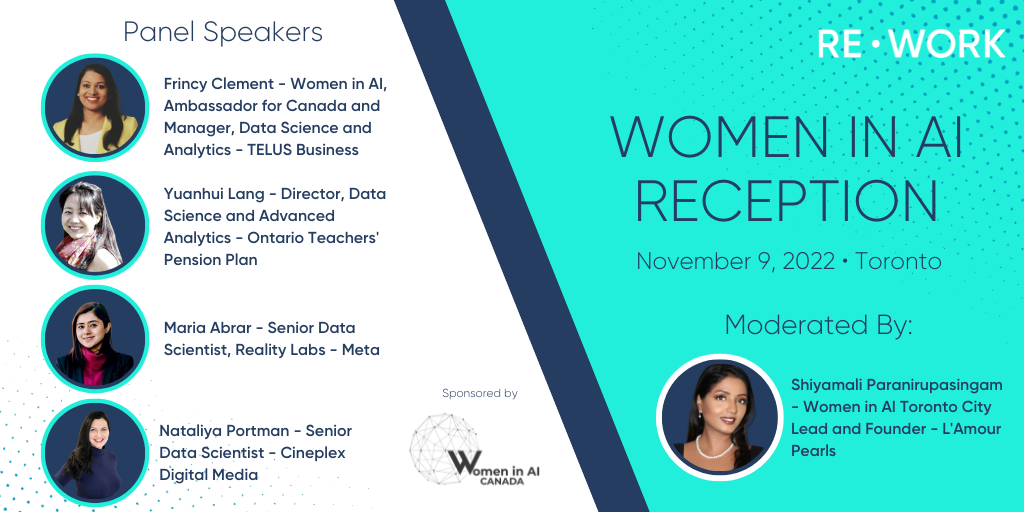
What advances and future trends do you envision within your industry as it pertains to AI, ML, data science?
"The world of Digital Out of Home (DOOH) advertising is a relatively new, emerging field for Data Science applications. Its remarkable richness of data, combining mobile trajectories of movement, anonymous videoanalytics metrics such as attention time, a number of people who had the opportunity to see an ad, consumer behavior and demographic characteristics, weather forecast, creates a playground for Data Scientists to experiment and to build audience discovery and content recommendation tools for brands to reach out to their target audiences.
Another important asset of DOOH ads is human creativity. Human creativity coupled with advanced anonymous video analytics technology has a global outreach effect! One such example of a DOOH creative that I could never forget, is a campaign to stop domestic violence against women in the UK. A bruised woman’s face, a victim of domestic violence, on a video wall gets healed every time a passer-by looks at it. In this way, this ad sends a message that everyone can make a change. Can you imagine combining the art of digital out of home with the art of Data Science?
Data Scientists can turn these digital media players into intelligent machines that will make decisions in real-time on what content to play in response to a changing audience and/or environment. And this is where the future of the DOOH industry lies, static posters and programmatically served ads on digital billboards will be replaced by AI-powered ads tailored to common human behaviors detected in front of the digital screens."
What are you most looking forward to while speaking at the Women in AI Reception?
"I am looking forward to sharing my unique experiences working as a Data Scientist in a male-dominated world and valuable lessons that I learned in a workplace and that are not taught in any higher education institution, in particular, for those of us who have graduated with STEM degrees. I would like to highlight some of the challenging situations that I found myself in when working as a Lead Data Scientist and the only woman on the team at the same time.
1. How to react when your ideas are stolen by your male co-worker?
2. How to communicate professionally when you are confronted by a competitive male co-worker?
3. What are microaggressions, how to recognize and to react to them?
4. How to handle ego-centric individuals on the team?
5. How to handle incompetency of your manager or a manager who does not understand your work and assigns unrealistic timelines?
6. How to handle male characters who want to dominate or to lead efforts to resolve a certain issue instead of you?"
Want to learn more?
Nataliya will be speaking at the upcoming RE•WORK - Women in AI Reception - Toronto on November 9, 2022, in Toronto, ON. Join her and many others to hear from leading female experts in machine learning & AI and discuss the impact of this rapidly advancing technology.
For more information, contact [email protected].