“Artificial Intelligence is going to steal our jobs.” “Killer robots are coming to take over the earth.” “Your doctor will be replaced by a machine.” - all phrases in the media in the past few months, and each as inaccurate as the last. Whilst it is indeed true that AI is transforming every industry it touches, the way it is portrayed in the news is often one-sided and without considering the bigger picture.
At each of our RE•WORK summits, we showcase the most current breakthroughs in AI and focus on how they’re impacting both business and society. For the first time, we’re taking a look at the way industry, academia and society work hand in hand to ensure that the technological advancements are able to be applied in industry, and for the overall benefit of society.
Expanding on the ever popular Deep Learning Summit Series, we have returned to San Francisco today and tomorrow for a triple-track event. The Applied AI Summit is focusing on implementing the most cutting edge AI methods in a real-world setting; the AI for Good Summit looks at how we can ensure all departments are assuming responsibility in leveraging AI to benefit society to tackle global challenges; and the Deep Reinforcement Learning Summit draws on the most current research breakthroughs through the combination of deep learning and reinforcement learning to achieve human-level intelligence. We have also seen experts host Deep Dive Sessions which are designed to allow attendees to delve into more detail on some of the key topics explored across the two days. These sessions vary from interactive hands-on workshops to demonstrations and lecture-style presentations.
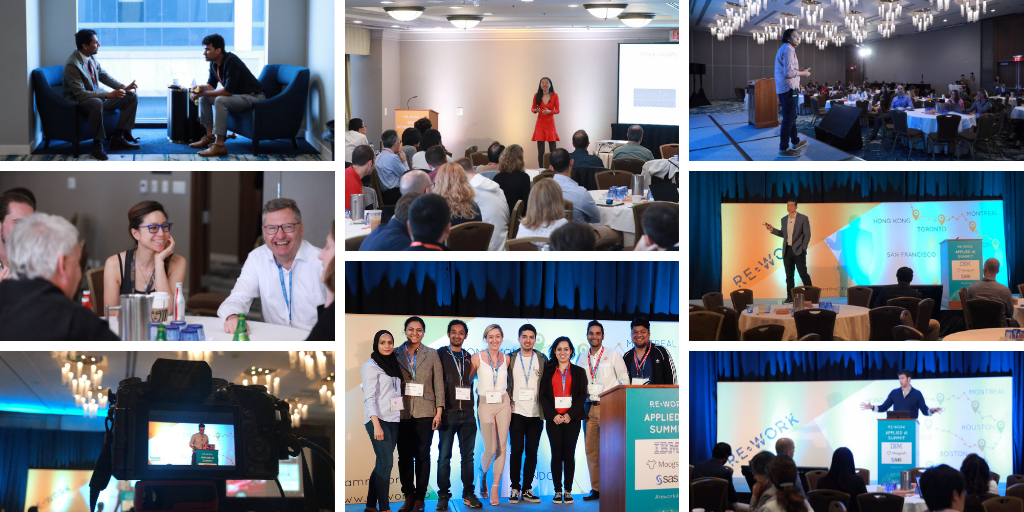
This morning kicked off with attendees getting to know each other over breakfast before selecting their first session of the day. With the attendee app up and running in advance of the summit, everyone had the chance to personalize their schedules to ensure they didn’t miss the most relevant sessions. There was also plenty of chatter on the app with people setting up meetings, engaging in polls, and arranging catch ups for the coffee breaks.
I found myself in the Deep Reinforcement Learning (DRL) Summit listening to the fantastic Dawn Song, Professor at UC Berkeley this morning speaking about secure DRL. She explained how DRL has emerged as an important family of techniques for training autonomous agents and has led to the achievement of human-level performance on complex games such as Atari, Go, and Starcraft. Dawn also explained that DRL is also vulnerable to adversarial examples and can overfit.
DRL has been making great advancements like winning over one of the world’s top players on AlphaStar, but as we deploy this, we need to be aware that there is a presence of attackers. Attackers follow the footsteps of new technology, and with AI the stakes are higher because the consequences will be more severe. We need to measure the right goals to approach better generalization, integrity and confidentiality. - Dawn Song
I also spoke with Dawn in an interview for the Women in AI podcast where we discussed this in more detail, as well as some of the challenges and successes in using DRL to train agents. You can subscribe to the podcast here and we’ll let you know when Dawn’s episode becomes available.
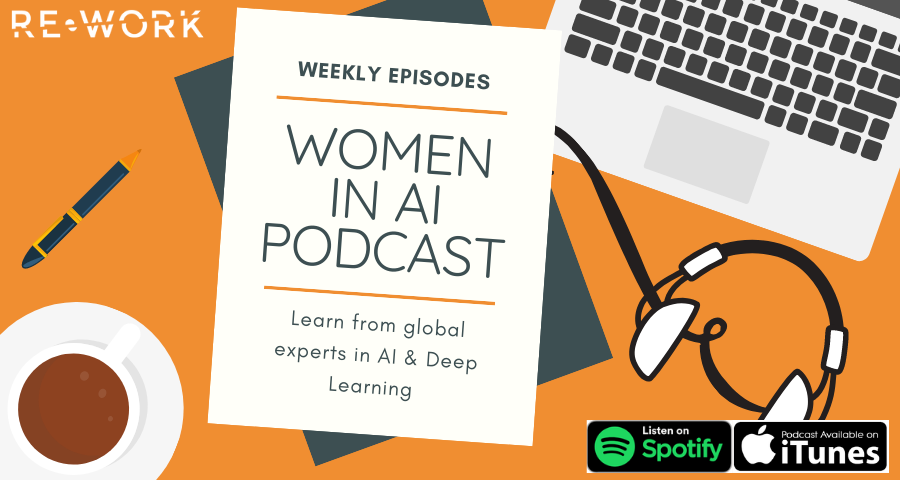
With DRL being a relatively new field of study, it was great to have Joshua Achiam, Research Scientist at OpenAI hosting the Deep Dive session “An Introduction to Deep Reinforcement Learning” where he took attendees through an introduction to DRL models, algorithms and techniques; examples of DRL systems; and ran us through case studies on DRL can be used for practical applications.
“When do you want to use deep RL? You want to do it when there’s a complex high dimensional non-sequential situation. For example when you want to control sophisticated robots or play video games from raw pixels or be the best at a strategy game. Deep RL has the potential to succeed in these tasks.” - Joshua Achiam
Building on this session later in the afternoon, SAS hosted a session focusing on ‘What You Didn’t Learn About Machine Learning in School' where Wayne Thompson, Chief Data Scientist at SAS filled in some of the blanks that may have been missed from college or online courses by mapping out how to tune and evaluate models and how to actually put these models into practice and model them once they’re deployed. C-level attendees, as well as technical experts, joined this session, all engaging in the more interactive Q&A session: When asked about generalization, Wayne explained that from the machine learning pipeline, what has to happen is everything has to be blueprinted, and “that is the number one reason why models don’t get deployed.”
He explained that once these models are deployed, they immediately begin to degrade. It is important to monitor model drift, retrain champion models and evaluate new challenges. Model fairness and bias must be addressed. Wayne suggested that it’s important to regulate and repeat the models to harvest and build many models with similar features.
Back in the session rooms, Lucas Ives, Engineering Manager at Apple was speaking on “The Art of Applied AI”, explaining that there is a particular approach to the problem-solving space that he thinks is missing from deploying AI in the real-world. “It needs to be driven by the creative person, not from the technical person”. He spoke about the importance of companies developing AI to actually serve their consumers, and more often than not this needs to come from a natural and creative perspective, rather than from a technology standpoint. “There’s been a quantum leap forward in the last 5 or 6 years in AI. With Siri, the word error rate was sat at about 10% before the introduction of neural networks in 2010; now if the environment is right, it performs better than humans. In his presentation, Lucas took some time identifying ‘Appied AI’ and explained that ‘some people see it as an incremental step towards AGI, some people see it as a narrow use of AI, some people prefer the term Machine Learning, but really it needs to be a combination of a variety of things that can be presented to a user to help solve their real-world problems.’
Following on from Lucas, Chul Lee from Samsung Electronics spoke about “The Challenges of Implementing” and explained how recent advances in AI have enabled consumer and mobile device companies to greatly automate their existing operations, and enable more seamless and compelling user experiences around their devices. He explained how recent trends and algorithmic advances in personalization, data analytics, audience science, and human-computer-interactions related to IoT, personal assistance, device interaction/control, media discovery and logistics. Chul explained that they are using on-device AI processing that improves “the picture quality of our TV's as well as our TVs as an AI assistant as a universal guide that can make personalized suggestions and target specifically. It’s important for us to understand what kind of content is being served so we can personalize it accordingly.”
Also discussing the applications of AI, Carla Bromberg, Co-Founder & Program Lead of AI for Social Good at Google gave an overview of the program, discussing examples and techniques Google and others are using to apply AI research and engineering efforts to social, humanitarian, and environmental projects, and hope to inspire others to apply AI for good. Today we’ve heard about people working in poaching predictions for conservation, natural disaster prediction, using AI in education and much more.
In her presentation, Carla spoke about her work in migration prediction in whales to help preserve endangered species: “We’re working with Noah who has underwater recording equipment - It would take a human 19 years to listen to the recordings, and they may never even hear a whale! Machine learning takes the 100,000 hours of recordings and finds the whale sounds. We took the underwater audio and turned it into visualization and annotated them with the species name. The more annotated examples we can show it, the better it gets. We can now see on a map where there’s a higher chance of finding the whales.”
At the Summit, attendees are welcome to attend sessions on all four tracks, and several attendees and speakers alike were enjoying the flexibility, finding that they were learning plenty of cross-transferable skills of their current work:
“You have such a great line-up in there and it's not just the organizations, it's the people from those organizations like those who I was sitting with at lunch.” Jeff Clune, Uber AI Labs
“It was a great talk from Anna from Intel speaking about using AI to protect wildlife. It’s amazing how AI can do things like this as well as crop prediction and other social endeavours people are working on.” Lisa Green, Data Science for Social Good
"I’ve not been to many events where you can go from super technical to looking at the bigger picture. We’re working with deep learning but are investing more and more in ethics and responsibility." - Mitchell, Microsoft Azure
We also hosted the increasingly popular Talent & Talk session during the coffee break and hear about vacancies from SAS, Moogsoft, Amazon, Bayer, Dolby Digital and many more. Matt from Numenta shared his vacancy and explained that their mission is to understand how intelligence works in the brain: “You don’t need a neuroscience background, but you need to be interested in it. We livestream all of our research meetings on Twitch and everything’s open-sourced. Only 2% of the neurones in your brain are firing at once which is sparse - most DL models are very dense which is the opposite to the brain, so we’re building DL models that only fire at 2% and they work!”
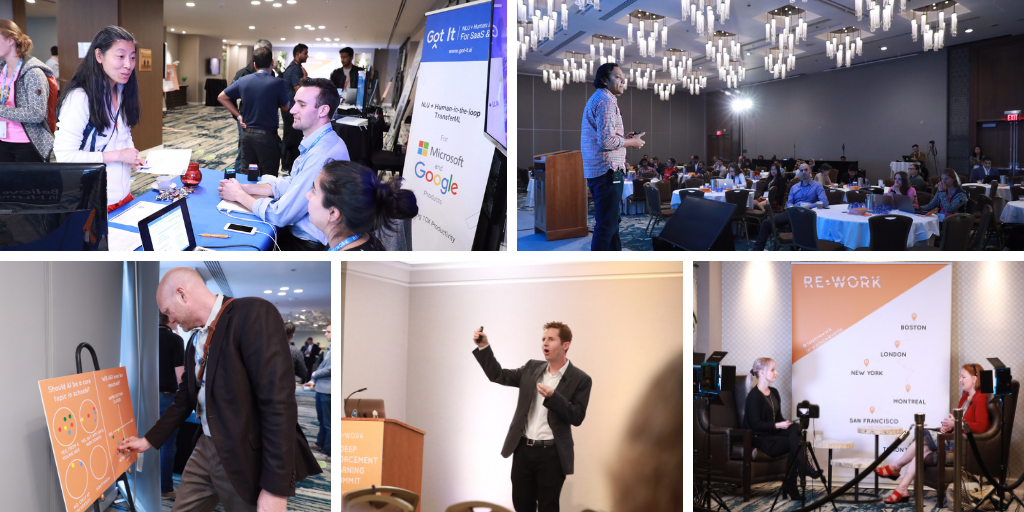
Back in the DRL summit room, we heard about “Learning to Act by Learning to Describe“ from Jacob Andreas from Microsoft Semantic Machines. He explained how there are a few problems at the intersection of language and RL - using interaction with the world to improve language generation and using models for language generation to efficiently train reinforcement learners. “When we move into our RL phase, we have no information, just an instruction following model. So what can we do with it? We know the parameters, but no instructions. So we have to search for instructions to identify what the DRL model wants us to do. We keep plugging them in to find the instruction and fine tune. We’re using the structure in the language learning data to tell us what’s important when searching for policies. We restrict ourselves to what’s relevant and meaningful.”
During the coffee and lunch breaks, I was fortunate enough to interview several of our speakers including Douglas Eck from Google, Karl Cobbe from OpenAI, Dawn Song from UC Berkeley and we had some really interesting discussions you can watch on our YouTube channel in a couple of weeks. With several press in attendance from various publications, Sonja Ried, CEO of OMGitsirefoxx also helped out with several interviews, speaking with Danielle Deibler from Jurispect, Alicia Kavelaars from OffWorld and Jeffrey Shih from Unity Technologies.
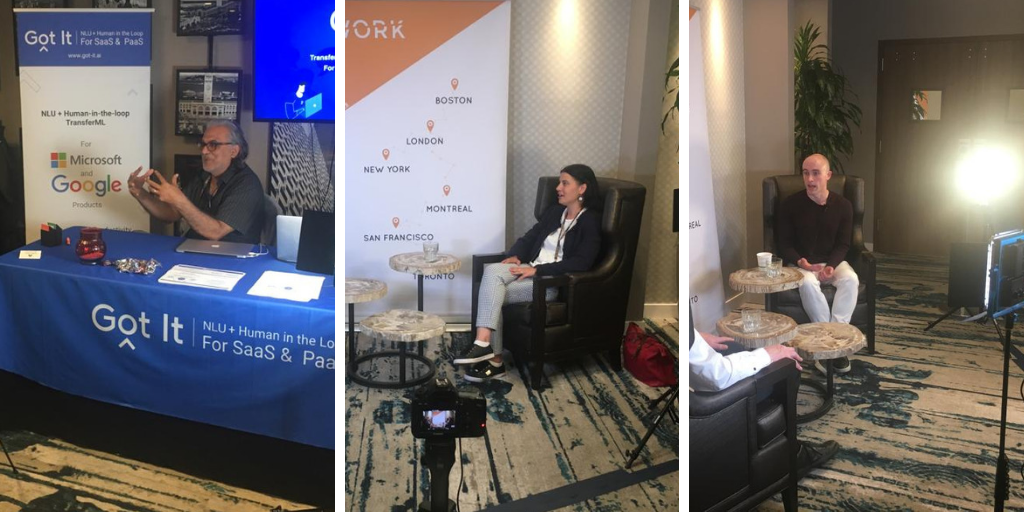
Back for this afternoon’s sessions, Junhyuk Oh, Research Scientist at DeepMind spoke about some deep reinforcement learning approaches that have been shown to perform well on domains where tasks and rewards and well-defined. Junhyuk is working on AlphaStar which is the first AI to defeat a top professional player in the game of Starcraft, one of the most challenging Real-Time Strategy (RTS) games. He explained that new agents tend to be strictly stronger than all of the previous agents.
One of the common themes of today’s presentations has been personalization, and speaking about how this can be used in business was Ankit Jain, Senior Data Scientist at Uber. He explained that whilst these techniques have been used in areas such as e-commerce and social media, it’s transferable to Uber by using past ride data to predict future journeys and patterns on a use by use case. He explained how they are training LSTMs to predict trips by combining past engagement data of a particular driver with incentive budgets and use a custom loss function (i.e. zero inflated poisson) to come up with accurate trip predictions using LSTMs. Predicting rider/driver level behaviours can help Uber find cohorts of high-performance drivers, run personalized offers to retain users, and deep dive into understanding of deviations from trip forecasts.
What else did we learn this afternoon?
Sherif Goma, IBM: Reinventing your company with AI and becoming a Cognitive Enterprise
Sherif explained how there is now an 'outside-in' digital transformation which is giving way to the 'inside-out' potential of reshaped standard business architectures and intelligent workflows. This has given birth to Cognitive Enterprises who are defining and pursuing a bold vision to realize new sources of value and restructure their industries, missions and business models.
Ashley Edwards, Uber AI Labs: Learning Values and Policies from State Observations
Ashley used an example in her presentation to communicate the DRL she’s working on. “If we’re building an IKEA table, it’s more valuable to have the pieces outside the box than inside the box, but then it’s more valuable to have the table built than to have the pieces on the floor. So we give these states values. We then apply these values in observation and use them in Deep Reinforcement Learning.”
Wrapping up the presentations of today was the panel discussion “What is Responsible AI and Where Do I start?”. As mentioned previously, ensuring that entire companies have ethical AI at the centre of their mission is integral, yet there are many areas where people feel that the research and applications take center focus, and there’s not enough time to focus on the social implications. The panellists spoke about creating transparent frameworks and common standards, as well as the positive impact of economic growth.
- Anna Bethke, Head of AI for Social Good at Intel - "we have a lot of projects ranging from earth conservation to social impact. We’re working a lot at online harassment at the moment and using NLP algorithms to figure out if we can deter and defuse the conversations with automatic replies."
- Tulsee Doshi, Product Lead for ML Fairness at Google - "I lead product for ML fairness. I get to work across products and have to learn how this is different across all our Google products. I’m looking at how developers can ask questions about their own products to see how we can ensure everyone is responsible."
- Devin Krotman, Director at IBM Watson AI XPRIZE - "we’re asking teens and startups around the world to pick global challenges and apply AI or DL to it".
- Adam Murray, Diplomat at U.S. Department of State - "we’re interested in looking in the international frameworks on AI and we’re looking at things related to the digital economy. It’s really important to foster trust in AI because it will boost our economy, boost innovation, but to do that we need trust. AI should be human-centred and fair, trustworthy, robust and ethical."
Some of the best networking happens outside of the presentations and sessions, to wrap up today we rounded off with networking drinks where we brought together all four streams.
We’ll be back tomorrow with more presentations, deep dive session panel discussions as well as a VIP breakfast and mixer drinks to see off the summit. If you missed out on joining us this time, make sure you keep up with tomorrow’s sessions by following us on Twitter at @reworkDL and @reworkAI #reworkDL #reworkAI.
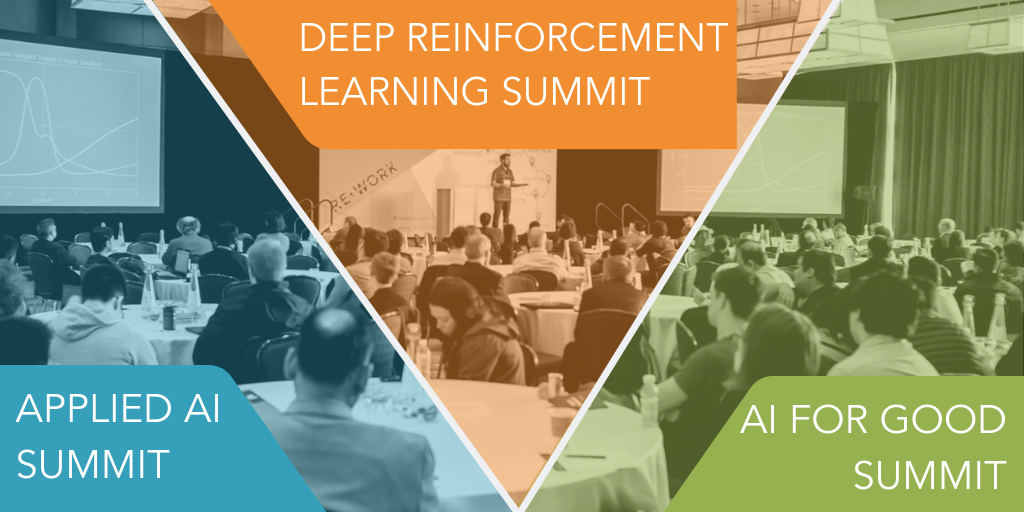