When you look at how children learn, they don’t go out there with intentions, they just start exploring and observing. We should look at machines with curiosity driven learning and see how they learn without just trying to solve a task. - Franziska Meier, Research Scientist, Facebook AI Research (FAIR)
Yesterday saw us bringing together some of the brightest minds in AI in research progressions, applications and the possibilities of applying AI for a positive social impact. We heard from Google, FAIR, Uber AI Labs, UC Berkeley, MIT and many more, as well as from our attendees, and here were some of the highlights.
This morning we welcomed back attendees who were ready to learn from some of the brightest emerging startups from across the globe. More of our fantastic speakers joined the RE•WORK team and press for interviews and podcasts, and we continued to explore some of the most cutting edge advancements in AI.
It’s also great to see everyone keeping engaged throughout the summit on Twitter, as well as on the event app:
@AlexJohnLondon: Listening to @CMU_Robotics George Kantor talking about #robotics for #agriculture and #AIforGood in San Francisco #reworkAI
@baxterkb: Poverty mapping in Africa by @atlasai_co at #ReworkAI. Current survey methods are inadequate to accurately measure ground truth. Satellite data too infreq. Their tech is able to fill in the gaps. Explainability important to communicate needs to NGOs.
@e_salvaggio: Hearing #research on political bias from @marvelousai, which is using language processing to discover political narratives on Twitter / social media, at #reworkAI
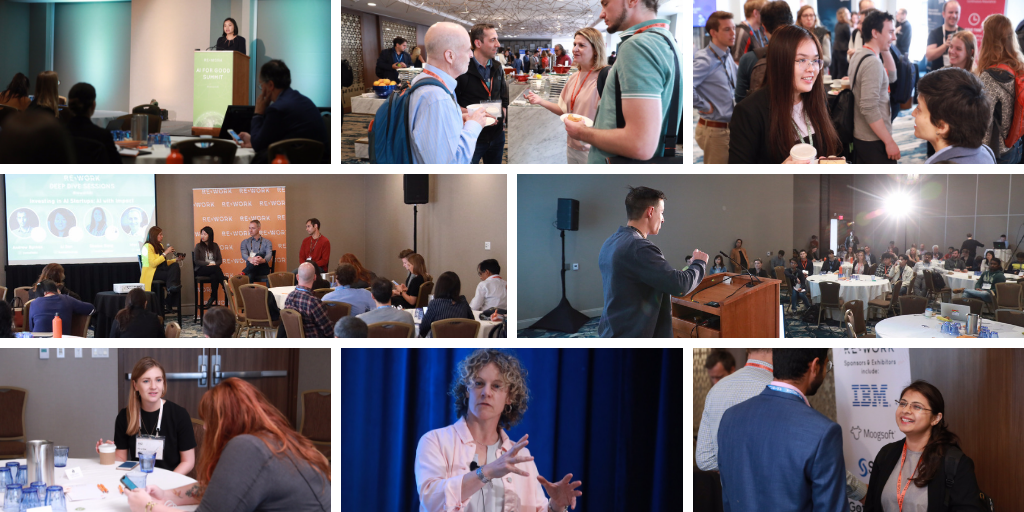
Rising stars
AI is a rapidly expanding field, and more and more, we are starting to see courses in machine learning crop up in high schools across the world. However, it’s still a new ‘subject’ in education, but at the pace jobs requiring computer science and machine learning skills are required, at RE•WORK we are really focused on encouraging the next generation into the space. Today’s Deep Dive Sessions saw us hosting the Rising Stars session where we heard from some of the next generation’s experts.
Eshika Saxena, Harvard University and TakeKnowledGE: The first step to increasing trust in AI is increasing awareness.
Arjun Neevanan, detoxifAI: I’m working to overcome bias in language labelling and I'm developing scalable models to de-bias toxic comment detection and make classification more transparent.
Ananya Karthik, Stanford University and creAIte: AI is an inherently creative and versatile endeavour. I’m excited about sharing my enthusiasm and empowering view with girls in my community in order to promote inclusion in tech.
Welcoming this morning’s sessions on the Applied AI stage, Davis Sawyer, Co-Founder and Product Lead at Deeplite spoke about building compact DNNs with reinforcement learning, and explored how although deep learning algorithms can deliver incredible performance, they require massive amounts of time, compute and expertise to implement. “If you’re starting with a pre-trained model and starting from that network, we explore the design space”. He explained how Deeplite helps humans automatically design deep learning models that are optimized for their task. “The future is small, so smaller, more efficient deep learning will enable new use cases and business value to be created.”
Following on from Davis was Rein Houthooft, Head of AI at Happy Elements who presented on “Generating the Best Game Experience through AI”. Happy Elements is the producer of one of the largest active mobile games worldwide and they’re currently working to optimize the gameplay experience of each player individually at massive scale. “Towards this goal, we research and develop machine learning algorithms and systems for dynamic game adaptation.” Davis and the team have found that optimizing game design through AI can improve user LTV/retention and enhance the player experience. He explained how there is some adaptation to user behaviour which is hard to maintain over time, so their goal is to apply machine learning into something that can be optimized.
After a full day learning about how AI can positively benefit society in areas such as the Government, wildlife conservation, agriculture and many more, we were back this morning to hear from Francesco Paolo Casale, Data Scientist at Insitro, a company that aims to improve the drug discovery pipeline by integrating the production of large-scale high-quality data with advanced machine learning and statistical genetics tool. As an example of how Machine Learning can help gaining new insights into human disease, Paolo presented his postdoctoral work at Microsoft Research on genetic analysis of medical images with deep generative models. Specifically, Paolo introduced a deep generative model which combines CNNs and structured linear mixed models to extract latent imaging features in the context of genetic association studies. He explained that “we optimize an autoencoder that can extract latent phenotypes from high-dimensional imaging data. This works very well when we analyze brain MRI scans, and it enabled us to find regions associated with brain structure and Alzheimer’s disease."
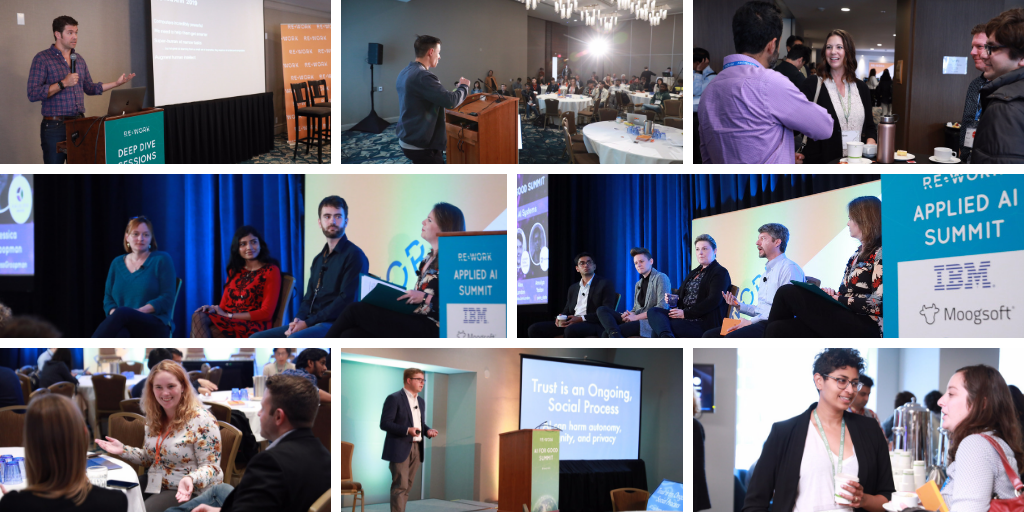
Over on the Deep Reinforcement Learning track, Franziska Meier, Research Scientist at Facebook began by explaining that current robot learning approaches assume that one can prepare a robot for every possible task and environment. She explained that by making this assumption, learning becomes a large data effort. "To be completely autonomous, robots need to be able to incrementally and continually acquire new skills, building on previously learned representations." Franziska explained that where humans are able to make mistakes and improve on this even if it’s a mistake that was made a long time ago, machines aren’t able to retain the information long enough and end up ‘forgetting’ their mistakes. Deep RL can provide a way for the models to keep learning whilst not forgetting what errors were previously forgotten.
Back learning about some of the most cutting edge applications of AI in the real-world, Andrew Zhai, Staff Software Engineer at Pinterest spoke about visual search products and how they’re encouraging users to take action on their inspirations. He took some time looking at what a visual search backend architecture looks like and explained that they’re training embeddings for visual similarity. “We want to make every image on Pinterest shoppable. We can decompose the scene and direct you to where you can buy it. we have hundreds of millions of visual searches each month so we need to ensure it's accurate and quick. Visual search also allows users to search within images. You can search a part of the image - you can press a little button to play around and find different parts of the images. We also have Pinterest lens where you should be able to take a picture of anything in the real world and get recommendations.”
In the Deep Dive sessions we were hearing about the Challenges of Accessible AI from Karla Monterroso, CEO of Code2040, Deval Pandya, Data Scientist at Shell, Preetham Vishwanatha, VP of AI at Course Hero, and Nikhila Ravi, Software Engineer at Facebook. They spoke about the definitions of accessible AI and why it’s important, as well as looking at how we as individuals and companies can play our part to increase accessibility.
Continuing the trend, Eddan Katz from the World Economic Forum addressed attendees in the plenary session speaking about his current work in AI & ML Policy and Governance Issues. "We're focused on social and economical issues and how we can address these with AI. We have projects ranging from AI to drones to autonomous vehicles, precision medicine and others. We've launched the Centre for the Fourth Industrial Revolution Network as a space for global cooperation. We want to be a 'do tank' not a 'think tank' on a global scale to champion ethics and values in technology."
Eddan focused on protecting children's rights and explained that “we really want to think through how kids are impacted by AI and the results it has on them in the long run. Our project is split into 4 phases - Opportunity Mapping, Framework Development, Prototyping and Testing, and Scaling and Adoption. We think about it in 'rights' and we want to think about the rights that children should have in automated toys and digital devices, so we're looking at privacy, transparency, optimizing algorithms for children and agency.” Eddan explained that they’re between the scoping stage and the framework development with the goal to scale and roll out this model.
In one of today’s many panel discussions, we heard from Jessica Groopman, Kaleido; Kathy Baxter, Salesforce; Kate McKall-Kiley, XD; Alex London, Carnegie Mellon and Amulya Yadav, Penn State who were focusing on Avoiding Human Bias in AI Systems.
Alex: bias is a term for all the ethical issues we’re worried about in AI. Generally bias is something that’s a deviation from a standard. We need to see relative to what is the deviation. We need to remember not all bias is bad, and it might be important for us to have systems that are biased in order for it to be ethical.
Kathy: it’s important to point out the difference between prejudice and statistical bias. Different groups within companies may have different definitions of what bias actually is. We need to make sure we’re on the same page so we’re not saying the same thing but meaning something different.
The panel went on to discuss the problems of bias in AI and some of the solutions. If you’d like to hear more from the panel, register for post presentation video access here.
The final session of the Deep Reinforcement Learning Summit was from Stephan Zheng from Salesforce Research who spoke about "Learning to Reason, Summarize and Discover with Deep Reinforcement Learning." He explained that recently, deep RL has seen remarkable success in complex simulated environments, such as single and multi-agent video-games. However, applying deep RL to real-life problems remains challenging due to several key obstacles, such as sample inefficiency, lack of strong generalization and inherent task structure complexity. "It works in clean environments, but in the real world this often isn’t the case. We’re looking at the applications such as summerization, translation, reasoning and other practical problems." Stephan walked attendees through multi-hop reasoning in knowledge graphs to find the correct facts. He explained that learning the structures of tasks can improve both speed and generalization. World-graphs have proved to be a powerful way to abstract an agent's environment and accelerate RL.
Wrapping up the Applied AI Summit, AI for Good Summit an Deep Reinforcement Learning Summit were our final mixer drinks where attendees, speakers, press and the RE•WORK team came together one last time to discuss what they’d learned and how they’re going to implement it in their businesses and research.
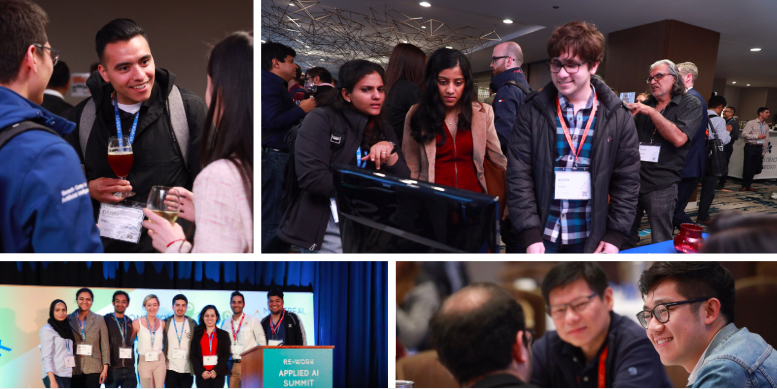
We spoke to some of our guests to hear how they’d enjoyed the two days:
Cassie Lutterman, SAS: It has exceeded my expectations from all facets. The amount of great conversation were having from the booth and the session there are so many people experimenting with AI. I like the community feel here at this event.
Nitin Gupta, Dori: I’ve been following your slack channel for around 6 months and it was great to finally come to one of your events.
Nina D’Amato, San Francisco Department of Technology: This is the most future leaning group I’ve seen and I love the skill sets that compliment each other. I’ve not seen it at any other summits. The panel in ethics, which is super charged in AI at the moment, was great and I’ll be checking out all the others later today.
Are you keen to join us at an upcoming RE•WORK Summit? We’ll be in New York for the AI in Finance Summit and the AI in Insurance Summit in September, followed by the Deep Learning Summit and the Responsible AI Summit in Montreal this October. If you’d like to learn more, get in touch with John at [email protected].
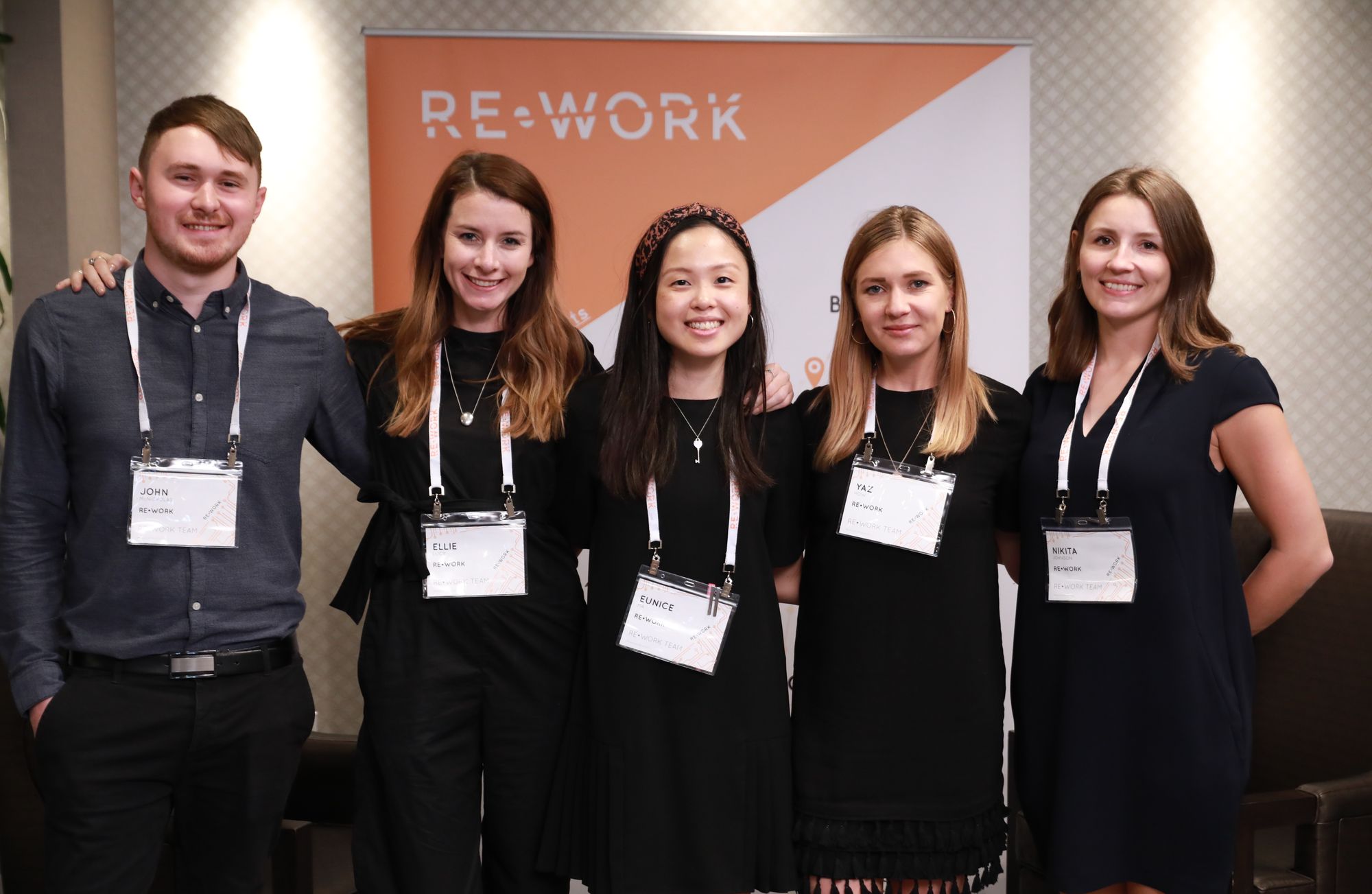