At the Deep Learning Summit in Montreal in October 2017, we saw Yoshua Bengio, Yann LeCun and Geoffrey Hinton come together to share their most cutting edge research progressions as well as discussing the landscape of AI and the deep learning ecosystem in Canada. We will be returning to Montreal this October, and Yoshua Bengio is already confirmed to speak at the Summit. We're looking back at the interview to see what we learned, and also what to expect at this year's edition of the summit.
For the first time ever, we brought together the ‘Godfathers of AI’ to appear not only at the same event, but on a joint panel discussion. At the Deep Learning Summit in Montreal, we saw Yoshua Bengio, Yann LeCun and Geoffrey Hinton come together to share their most cutting edge research progressions as well as discussing the landscape of AI and the deep learning ecosystem in Canada.
Joelle Pineau from McGill University who was moderating the discussion began by asking each pioneer to introduce their neighbour, which immediately generated a laugh from the packed auditorium.
Yoshua kicked off by saying ‘here’s Yann, I met him doing my masters and he was doing his post doc with Geoff and later Yann invited me to come and work with him and start working in convolutional neural networks, and it’s still the hot thing today!’
Yann went on to introduce Geoffrey and said ‘I’ll be historical as well, when I was an undergrad I studied neural nets and realised there was no research published in the 70s. I saw a paper entitled Optimal Perceptual Inference and Geoff was one of the three authors. I read the paper and knew I needed to meet Geoffrey.’
We finally heard from Geoff who joked that he might have been the supervisor for Bengio’s thesis, but couldn’t actually remember. He went on to say ‘It was a very nice thesis and it was applying neural networks to speech recognition. Yoshua and I have done well in Canada because Canada supports basic research. Things are happening very fast now and I just can’t keep up with Yoshua now! There’s a couple of archive papers a week and I’ve been particularly impressed by his work on attention and my feeling was that Yoshua was the youngest and he had some catching up to do, but unfortunately I think he’s caught up! He’s now made the impact that Yann made on CNNs in his own field.'

Can you tell me the difference between researching and working in deep learning now and back in the 80s and 90s?
Bengio: Back then, you could focus on research without all the noise. It was a totally different environment. There are some interesting parallels between when we met each other where and neural nets were still marginal and what happened maybe 5-10 years ago. There was a period in early 90s when neural networks were hot and there was a lot of hype with companies really trying to exploit it, so there are some parallels with now, but now it really works.
LeCun: I think it did work then! But people working on perception in the 60s decided that it wasn’t a valuable path so they started changing the names of things and they way they went about things, which had huge practical consequences.
Hinton: They’re too young to remember that!
LeCun: There’s stuff from back then that’s still in AI textbooks from neural nets in the 90s that isn’t so relevant any more, but they’re still used as reference - they’re useful but they weren’t the path to AI. A lot of techniques that we use today will disseminate widely and go underground in the same way unless we find the next step in progress that will keep this technique alive, but if not, it will just die.
Hinton: What he said!
Amongst your papers is there something we should be reading that’s been ignored?
Hinton: Figure the paper that’s one below your H number (laughs). I wrote a paper in 2008/9 that’s using matrices to model relationships and matrices to model concepts. So it’s given triples and you have to make the third from the first two. I did a lot of work on this in the early 2000s, it’s basically early embeddings. I was told to move on as I only had one reference in the whole paper that was a non self citation! The idea was that instead of using vectors for concepts and matrices for objects, you use matrices for both so it can do relations of relations. We taught it that 3 + 2 makes 5, then we taught it 2 and + makes +2, so the output it had produced had never seen this concept before so it had to learn itself. The paper got a 2, 2, and 3 out of 10, I then sent it to cognitive science, and they didn’t like it but they said ‘if I’ve understood the paper right it’s amazing, but I don’t think our readers would be interested!’
Bengio: I didn’t even try to submit mine because I knew it would be rejected! It was about the idea that in order to learn we need guidance from other humans, but that’s another story.
As time goes on, it seems you agree on more and more. You’re the ‘three amigos of deep learning’, but are there areas where you still strongly disagree?
Bengio: Is this a trick question?
Hinton: Politics! But we agree on American politics
LeCun: we disagree perhaps on approaches to problem solving rather than the actual problems. There was a time when Geoff was using probability…
Bengio: Yann wanted to know nothing about probability, he called Geoff the probability police
There are many people have been active in DL and Neural Networks, will DL always be in AI or are there other areas that will have as huge impact?
Bengio: We definitely need new ideas to build on what we have now. These ideas will be inspired by what we have now, and will be built on to create something new.
LeCun: the concepts will be parameterised and grown - they’re not going away, but what we have now is of course not sufficient so we will think about new architectures - lots of people are excited about dynamic architectures, and there are interesting things happening in natural language processing. What we need also is more ways of training very large learning systems - it might not be the ultimate answer, there could be ideas that come back. There will be some way of connecting DL with more discrete things like inference.
Bengio: We need to find ways with ML and DL to bring back objectives and train and teach them with our new methods so they’re a crucial contribution to AI.
Hinton: Yann an Yoshua believe this as well - the biggest obstacle is not having an objective function of unsupervised learning. I published a paper in ‘92 about the idea of spatial coherence being an objective function. When we have this, we’ll be able to learn more layers and learn more stuff on top of that. We’ll also train autoencoders.
Talking of objective functions, with the results we found the goal - the system that was able to beat people at Go. We didn’t know when we’d solve it, but what do you think is the next challenge and next thing we’ll solve?
LeCun: At Facebook, there’s a team working on Starcraft. This game is way more difficult than Go as it uses strategy, multiple layers, skill - you don’t know what your partner’s doing, it’s actually a form of serious professional gaming in Korea, it’s very challenging. There are a few bots that play it but not nearly at human level. The Facebook team and also a team at DeepMind are using machine learning, and I think we’ll see some progress there, but really how to train a car to drive is the next life changing problem we can solve - is there a way to do this completely and safely?
Bengio: I’ve actually been working on a recent project. An AI game with a human and a baby AI. The human teaches the baby AI using natural language, pointing, and all the things you’d expect from a parent. This happens in a virtual environment. It’s a game is for the human to indignity the best and quickest way to do train the baby without having to ask too many questions. It’s great, because the game could be fun for the human and also help to collect huge amounts of data to learn how using reinforcement learning we can identify the correlation between natural language and environment.
Do you feel like there are problems in AI that if we were to solve you would retire?
Bengio: There are some really hard problems that remain to be solved, and it’s fun! I want to know how machines can discover high representations to explain the world. There are general assumptions to explain the world and interims to be statistically efficient - this is fairly simple, but not so simple to solve.
Hinton: One particular thing that would have an impact for me is in natural language processing and language understanding. There are sentences like ‘the trophy wouldn’t fit in the suitcase because it was too small’ and the trophy wouldn’t fit in the suitcase because it was too big’ - in the first one it’s the suitcase that’s too small, in the second it’s the trophy that’s too big. We infer this and assume this because of the structure of the language, but if you translate these sentences into French, there are other factors to consider - does the machine get the genders right? It’s the context that you have to understand. If a machine could do a whole bunch of those translations successfully it would demonstrate that they really understand what’s going on, but I think the sets need to be about 1000 times bigger than they are now in machine translation for it to work perfectly. If we can do that and they get all that common sense it’ll be huge. It’ll convince people in old fashioned AI that it’s not right by luck, but they really understand what’s going on. I think it may well be 10 years before we can do this
Bengio: I think we should get machine learning to explain what sleep is for and what it’s about. I think that it’s odd that people don’t question why we spend more than a third of our lives sleeping, but if you deprive people of it they go crazy! We like 8 hours of sleep and we don’t know why. I strongly believe it’s telling us something about how we do learning.
LeCun: How do we get machines to achieve common sense? Unsupervised learning, objective functions in representation space, it might 10 20 years we don’t know
Hinton: Or it might take a week!
So moving away from the technicalities and towards the ethical implications - which ethical aspects are most likely to keep you up at night?
Bengio: Well for me it’s the miss-use of AI and products we’re making. For example I’m particularly sensitive to the use of AI in intelligent arms weapons - this could be really dangerous, and I think governments should sign treaties. Also, AI in advertising in a manipulative manner can be really dangerous for democracy. Eventually the question of AI falling in the wrong hands are really troublesome.
LeCun: If it’s used by people with a malicious intent this could be really bad. The fact that machine learning methods can be used badly in situations that cause harm. For example when you train a machine with biased data the machine will be biased, and when you train a system it replicates the behaviour of the humans who train it. It’s technical question as well as ethical - how do we remove bias? The image of AI in the public could become tarnished so we have to be proactive about this. I’m working with ‘The Partnership in AI’ who come up with guidelines to deploy tests and so forth to keep it safe.
What advice you’d share with young people working in AI now?
Hinton: If you have a strong intuition that something’s a good idea and everyone else says no, take the hint that it’s not a bad idea but it’s actually an original idea. Then think, should you work on your ‘good idea’ or not? If you have good intuitions work on what you think, if you don’t have good intuitions it doesn’t matter what you do!!
Bengio: Listen to Geoff
LeCun: The three of us are very intuitive, we come up with concepts and idea by intuition and sometimes other people tell us no, so some of the most interesting ideas aren’t the most complex, but the way you implement it might be, it’s surprising it takes so long for people to realise some things are good ideas!

After the panel, Geoffrey had to dash off, but we were fortunate enough to have Adelyn Zhou from Forbes and TopBots conduct an interview with Yann and Yoshua which we will be sharing on our video hub in the next couple of weeks. You can subscribe to the video hub here to watch presentations and panel discussions from the Deep Learning Summit in Montreal.
To join us on the next stage on the Deep Learning Summit Series register for Early Bird discounted passes for this year's edition of the Deep Learning Summit in Montreal. Discounted passes are available until August 30.
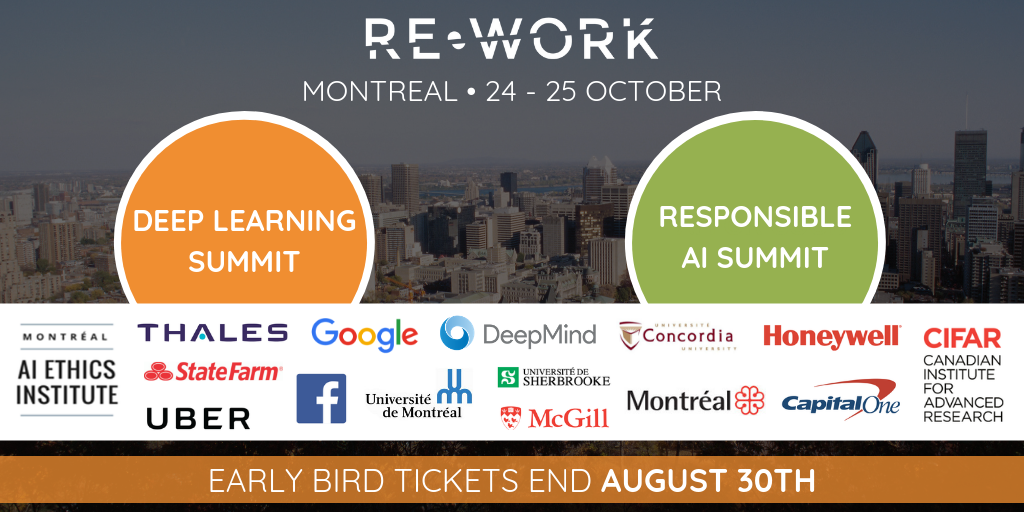