After a hugely successful day at the Deep Learning Summit, AI Assistant Summit and AI in Retail and Advertising Summit in London, we were back for a second day in London. Find out what you missed yesterday here.
Attendees were continuing to network and discuss the previous days presentations over breakfast before making their way to the session rooms for a morning of talks ranging from personalised depression care through robotics to the steps being made to better interpret Neural Networks.
We kicked off the morning in the AI Assistant Summit with Martin Goodson, Chief Scientist and CEO of Evolution AI proclaiming that the days of time consuming and mundane back-office tasks are soon to be over due to breakthroughs in computer vision and machine learning.
“Some of our customers get up to five million documents in a year, the human outlay for which is incredibly high and the chance for mistakes is increased with the volume of documents.”
Martin went on to explain the level to which these new tasks are accurately performed, with some organisations already automating their financial departments, citing that through training on active learning techniques, their recognition software can adapt to human deviation of norms, which is imperative due to the varying nature of some accountants and workplaces for procedure. Why Finance? Martin explained that this industry, once algorithms have been built, provides an environment in which may tasks are standardised with incremental changes made on repetitive tasks. This was said to give the platform a good base for learning. The next steps? Potentially handwriting! Whilst this would be an extremely complex step to take from block-print text, there are steps which can be taken to incrementally move toward text recognition in this format, with languages using symbols a logical next step in development.
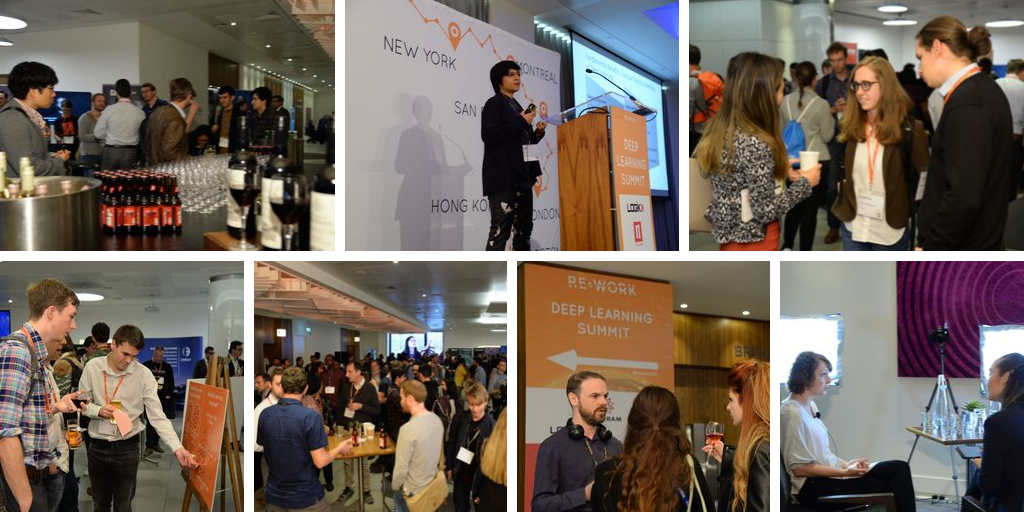
Next up we were back in the Deep Dive room to discuss the step toward better interpretability of Deep Neural Networks at HSBC. Roshini Johri, Senior Data Scientist broke down some of the major challenges faced in industries which rely on strict data accuracy, including finance and healthcare, stating that “We need explanations that not only data scientists understand, but what our customers understand too.” Roshini also mentioned that the idea of a ‘computer said so’ culture must be avoided. To gain trust, companies must be held accountable for their decisions and be able to explain this to users “You wouldn’t say to someone that you need to do a medical procedure and not tell them why”.
After the initial points including reliability, explainability and trust were covered, Roshini discussed LIME, a recent model developed at HSBC which provides local model interpretability and how it can be implemented at scale through the use of Deep Neural Networks.
“We’re moving more and more towards an era where we work with DNN’s. It’s impossible for our brains to comprehend the amount of data the model is processing and what it’s doing.” That said, Roshini was also conscious of the ethical nature of her work: “It’s our responsibility as data scientists to make sure we’re doing ethical things. I need to be aware that my data is representative of the demographic I’m working with”. The steer toward an ethical standpoint could not come at a better time due to the current media spotlight of AI development, especially as Roshini was quick to note the agnostic nature of LIME, only caring about input and output and, therefore, not customer reaction.
Whilst models are increasingly being used in Finance for automation and definitely seem as though they are the ‘future’, Roshini suggested that there has been some hesitancy from industry to adapt “Trying to explain an ML model to people in business in finance is way harder than my whole masters in AI. So I need to come up with visualisations and features that explain it well. We want to be able to deploy the right models and justify them correctly.”
The mixture of startups, academics and industry professionals was evident in the midday session, with our panel of investment experts gathering to share industry insights and tips for entrepreneurs at the summit. The main talking points of the session covered the short, medium and long-term challenges in investing in AI to solve important problems in society as well as the main success factors for AI startups and the challenges faced from the viewpoint of VCs. The session included an in-depth insight into the current workings of startups, with points made on the importance of building a competent and skilled team and also futuristic thinkers. The final thought of the session? Where our investors see their investments going in the next five years:
Steve Collins - I love AR (augmented reality), we are close to a tipping point where devices are not obstructive. We haven’t yet had a device that has connected us to the information that the internet has which is exciting
Uzma - Technologies that are augmenting humans in any forms to drive efficiency are interesting and also gene editing and reprogramming biology. It’s exciting what you can design with life!
John Spindler - What really excites me is the founders I work with. Working with academics who have become entrepreneurs is much more intellectually stimulating.
The penultimate presentation of the morning saw tech giant Google take to the stage on the AI in Retail and Advertising Summit. Darragh Kelly, Data Scientist at Google discussed at length the way in which Google are able to decipher customer reviews through the use of transfer learning. Darragh explained that customer reviews are an incredibly overlooked resource for business which can be neglected due to the mammoth task of sifting through large volumes of data, a huge waste of actionable insights from millions of customers.
Darragh was quick to present the shortcomings of Google’s algorithms which utilise customer data, suggesting that not all data collected is usable at this time:
“Whilst we have the universal decoder which is multilingual, it doesn't work in every language yet. Our current variety of translation API's need to first start off with one single language. Currently we can give them a sentence and it's able to understand and which words are dependent on others. This is useful for customer reviews as they explicitly tell you what they think about your business or experience. This will help you focus on the nouns and then try to represent an embedding for the entire review. Understand which reviews are similar and which can be easily clustered into a particular topic.”
Neil Lawrence was up next to present his work on ML system design, giving an overview of where we are now with machine learning solutions, and what challenges we face both in the near and far future. Examples given included practical application of existing algorithms in the face of the need to explain decision-making, mechanisms for improving the quality and availability of data, dealing with large unstructured datasets. Neil suggested that the problem we are currently facing is that automation forces humans to adapt as it adapts to us. Through this adaptation, however, the systems we work with in AI remain increasingly fragile. Neil continued to suggest that a good way of testing algorithms is to use the younger generations, with children testing capabilities in ways in which we do not think to!
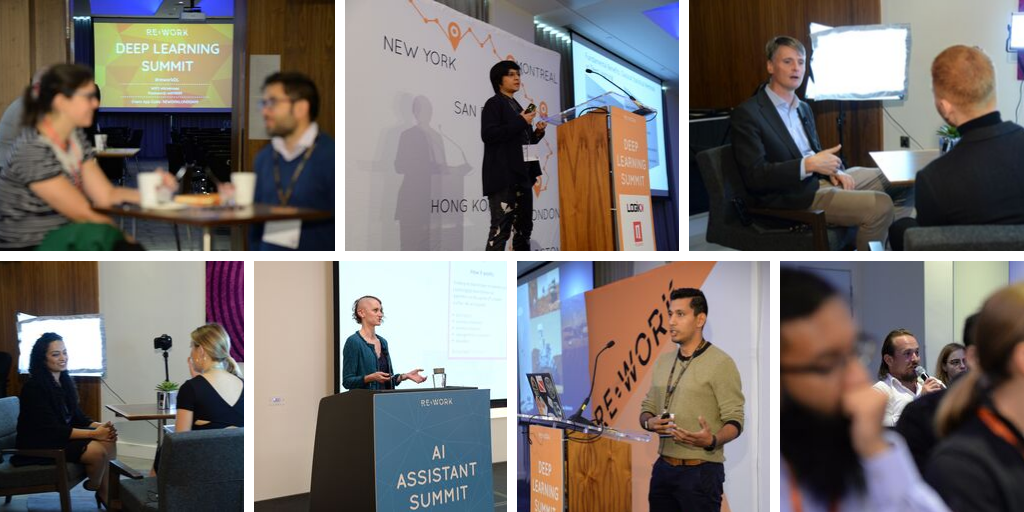
One of the most engaging talks of the afternoon came from Shreyansh Daftry, Research Scientist at NASA JPL, who is currently working at the intersection of Artificial Intelligence and Space Technology to help develop the next generation of robots for Earth, Mars and beyond. Shreyansh began his talk by recognising, as many had before today, the difficulty of finding both usable and well labelled data for algorithms baselines
“Getting good labelled data is hard. It doesn’t help to put lots of people in this because only a handful of geologists in the world actually understand this. So how do we train the DL model without much data? We need to develop continual learning.”
The need for further training data was also referenced in a funny anecdote:
“The need for situational awareness is one of our biggest challenges. There was a situation where a robot looked at a surface that appeared to be rock but was actually sand. It approached the terrain as if it was rock and it got stuck. We need machine intelligence to classify these kinds of terrains and make an informed decision on how to behave and move second by second as the terrain changes”
That said, the development at NASA is continuous and fast paced. “We started as a rocket making company to a satellite making company and we’re now the center making robotic spacecraft to support and improve exploration in space. We are now looking at creating intelligent machines that can operate without human input. However, the further you go, the harder it gets!”
As the talks came to an end, attendees once again gathered for networking drinks to close the summit discussing some of the subject matters covered over both days, whilst also voicing their thoughts on the summit as a whole
Elizabeth Traiger, DNV - “Thank you for a really well run conference, it was my first time here and I really enjoyed it. I’ll keep an eye out and see when the next one is because I’ll definitely be back.”
Neil Lawrence, Sheffield University - “It was great to reflect on my first talk here 3 years ago and how the technology and themes have grown over time. An excellent mix of research, startups and corporations.”
Laura Thompson, Phenomenal Training - "Congrats on a truly brilliant event. What struck me was the amazing mix of people- from the retail audience with Prada handbags alongside the techies with their black rucksacks. I’ve never seen those two sides mingle quite so much and it is a testament to your breadth and depth of audience education opportunities to have appealed to so many types of people."
Adam McMurchie, Barclays - "As always it's been great guys, I will be back again! It's great to see how it has grown so much over the last few years, there were hardly any techies like this five years ago!”