‘Data science is as much business as it is science, and data based “decision-ing” is at the core of every step of the customer journey in retail finance.’ - Anurag Setty, Barclays US
“AI is reshaping the future of finance.” It will define and reshape the financial industry through everything from identification, connectivity to credit and risk control. Today at the AI in Finance Summit and AI in Insurance Summit, global experts came together to share and discuss some of the most current progressions in the industry in presentations, panel discussions, round table sessions and deep dive workshops. Throughout the day we heard from some of the leading institutions and companies pushing the boundaries of traditional methods in areas such as underwriting, stock market prediction, algorithmic trading, insurance optimization and more with AI and machine learning.
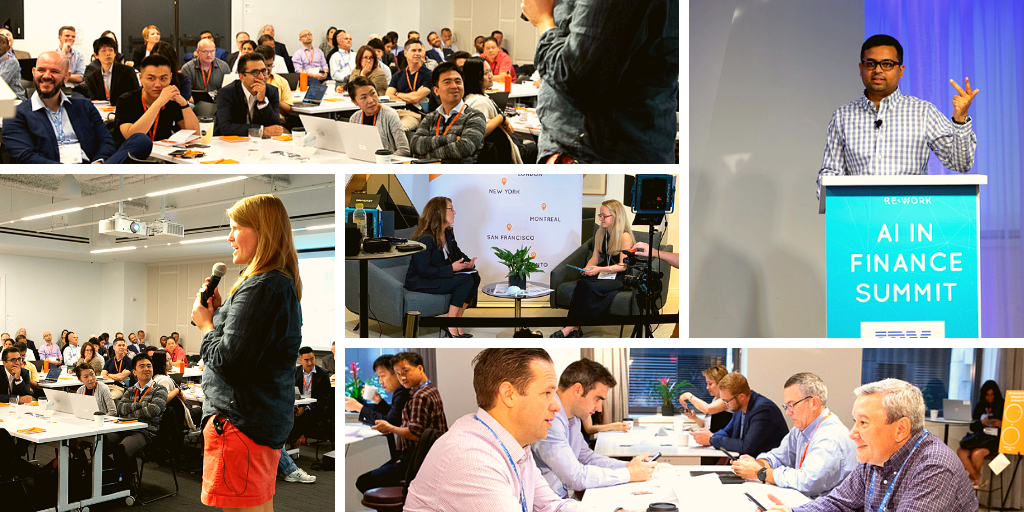
Both Insurance and Finance are long standing, and integral to modern life. This means that lots of the strands of these industries come with historic, well-tested processes, however, as technology has progressed, these processes have become archaic and inefficient. Today’s event brought together some of the leading minds disrupting the traditional models in an effort to break boundaries and revolutionize finance and insurance. Attendees were able to attend sessions from both the AI in Finance Summit and AI in Insurance Summit and Deep Dive Sessions with one ticket, and today’s session started off with the Finance compere for the day, Gene from FINRA introducing our first speaker.
Anurag Setty from Barclays shared his work in “Trends in Algorithmic Marketing and AI in Retail Finance” and explained how “advances in data science and machine learning have redefined marketing by expanding the role of algorithms in key decisions within retail finance.” Algorithmic marketing can be broadly summarized, and Anurag explained that this is through applying programmatic approaches to automate evaluation of target-offer mix and optimization towards business objectives.
Over on the AI in Insurance Summit, Boyi Xie from Swiss Re shared his work in using AI to Advance the Insurance Value Chain. AI is transforming many industries, including insurance. AI, as used here, ‘refers to a collection of modeling methodologies, tools and platforms, applications, big data utilization, and the thought process for problem solving in the field of AI’. As insurance is a highly specialized industry, the AI adoption needs to be customized. However, we have already seen many opportunities and high potential applications. "By accelerating underwriting we can build a better ecosystem to make better decisions. We use a decision tree, we love decision trees! I would say that it’s a community with an open mindset, even though it’s a classic model. It helps us with explainability which is really important in Insurance."
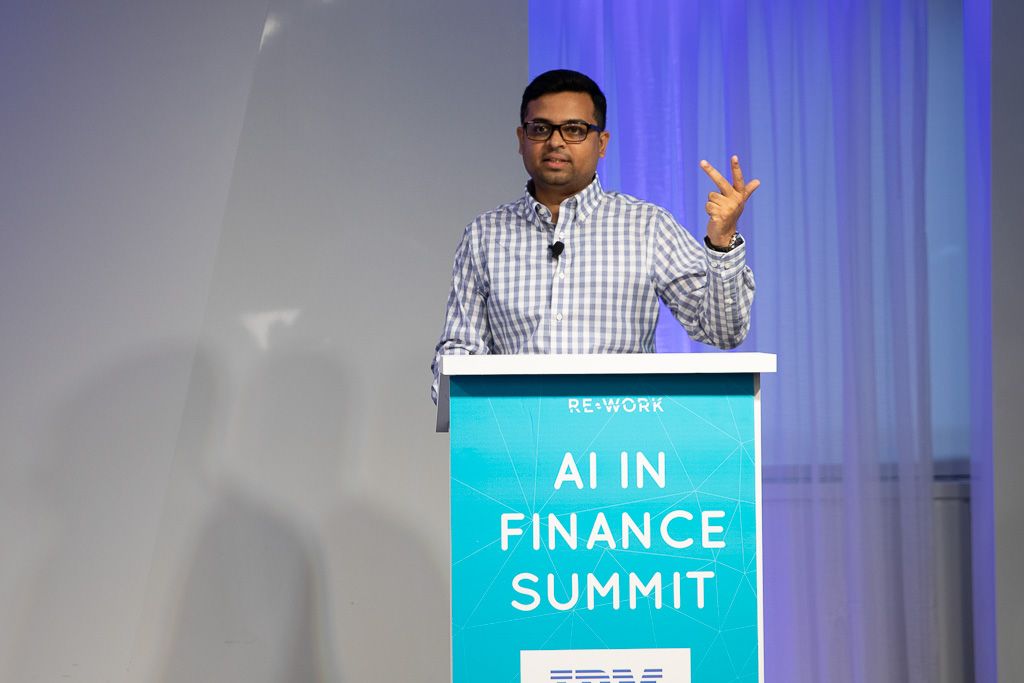
During the morning’s coffee break, experts from Square, Prudential, Swiss Re and Barclays hosted a roundtable discussion for people new to the field. They covered questions such as ‘how do I apply AI in my business?’, ‘how do I develop ML for customer facing products?’ ‘how can I use AI to enhance the value chain’ and more.
What else did we learn this morning?:
Alan King, IBM, AI in Finance Summit:
“Fusion AI is an idea that started because we deal with international banks and often you can’t move data across an international boundary. We want to use this fusion idea so that when an entity in a bank allows the parameters to be outside of government entities. We are looking at a lot of opportunities to use reinforcement learning at IBM for financial applications. The challenge is getting enough data. At the moment, these applications are primarily trading."
Julia Romero, Haven Life, AI in Insurance Summit:
“In product development we make a lot of assumptions. The way we make assumptions is interesting. Product design and assumptions drive premium rates. Better assumptions can give you an advantage in pricing. Machine learning AI is good at complicated relationships and making better assumptions”
Michael Natusch, Prudential, AI in Insurance Summit:
“We are trying to build Self-Learning Systems. Without data we have nothing. We need data, models and user interface.”
After a busy morning hearing from both industry and academia about the breakthroughs in the industry, it was time for lunch and for attendees to discuss the opening sessions.
“I really enjoyed Leman from Carnegie Mellon University giving an update on her Anomaly Mining and Detection research.” Robert Zembowicz, Vanguard
“The value for me is in the conversations. I’ve connected with plenty of people on LinkedIn so I think we’re all set!” Michael Richardson, Nationwide Insurance
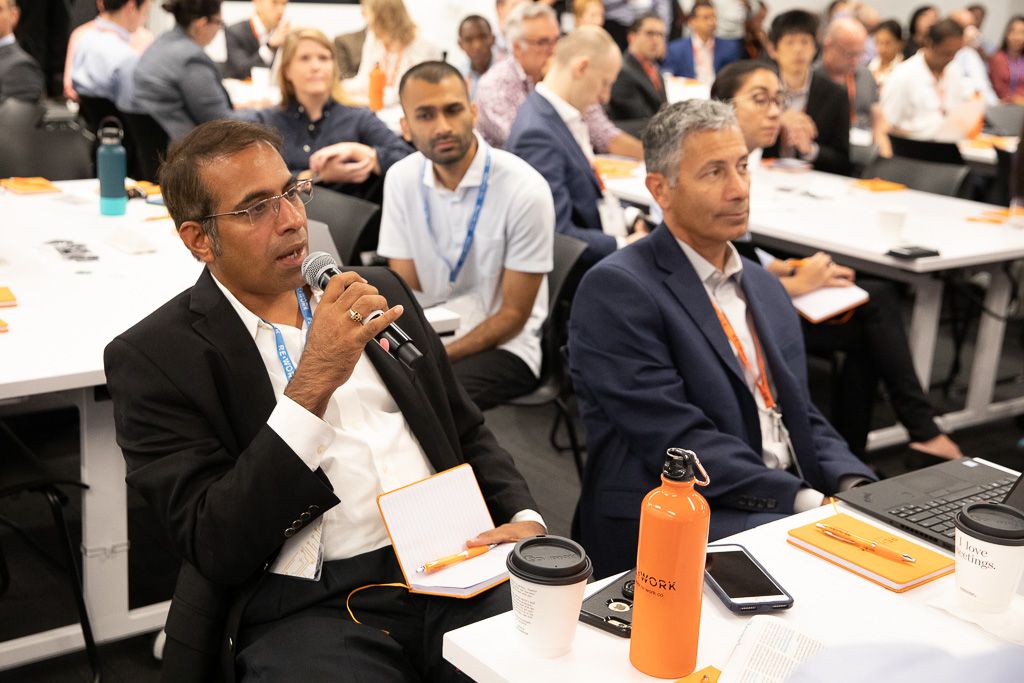
After everyone had refuelled, it was back into sessions where Baiju Devani, SVP, Chief Data Officer from AVIVA welcomed everyone back, getting stuck into algorithmic and data-driven business growth in AVIVA’s Applied AI Journey in the AI in Insurance room. Highlighting the importance of cross-industry collaboration, he explained that "the best place to look for the lead in change is outside this industry. The algorithmic revolution is happening everywhere. We need to be thinking about algorithms first, not just AI first.”
On the AI in Finance track, Frederic Godin, Assistant Professor at Concordia University explained how traditional hedging procedures are based on local sensitives of risk factors called Greeks, but these approaches don’t consider the interaction of hedging factors over time. He explained how hedging outperforms traditional methods by improving risk optimization which is especially true for long dated options. It’s really flexible as you incorporate many features into the hedging scheme which works in a high dimensional framework when it’s combined with machine learning.
Following on the trend for the importance of AI in modern banking, Rohit from MasterCard explained that although “MasterCard started as a payment company, it has changed to an information technology company by facilitating payments and utilizing technology. Now it’s moving from information technology company to an AI company. Every transaction in and out is digitally merited.” He went on to explain that their financial model at MasterCard is "based on the flow of data ingestion and sanitization auto algorithm selection and prediction, error analysis and parallel computation.”
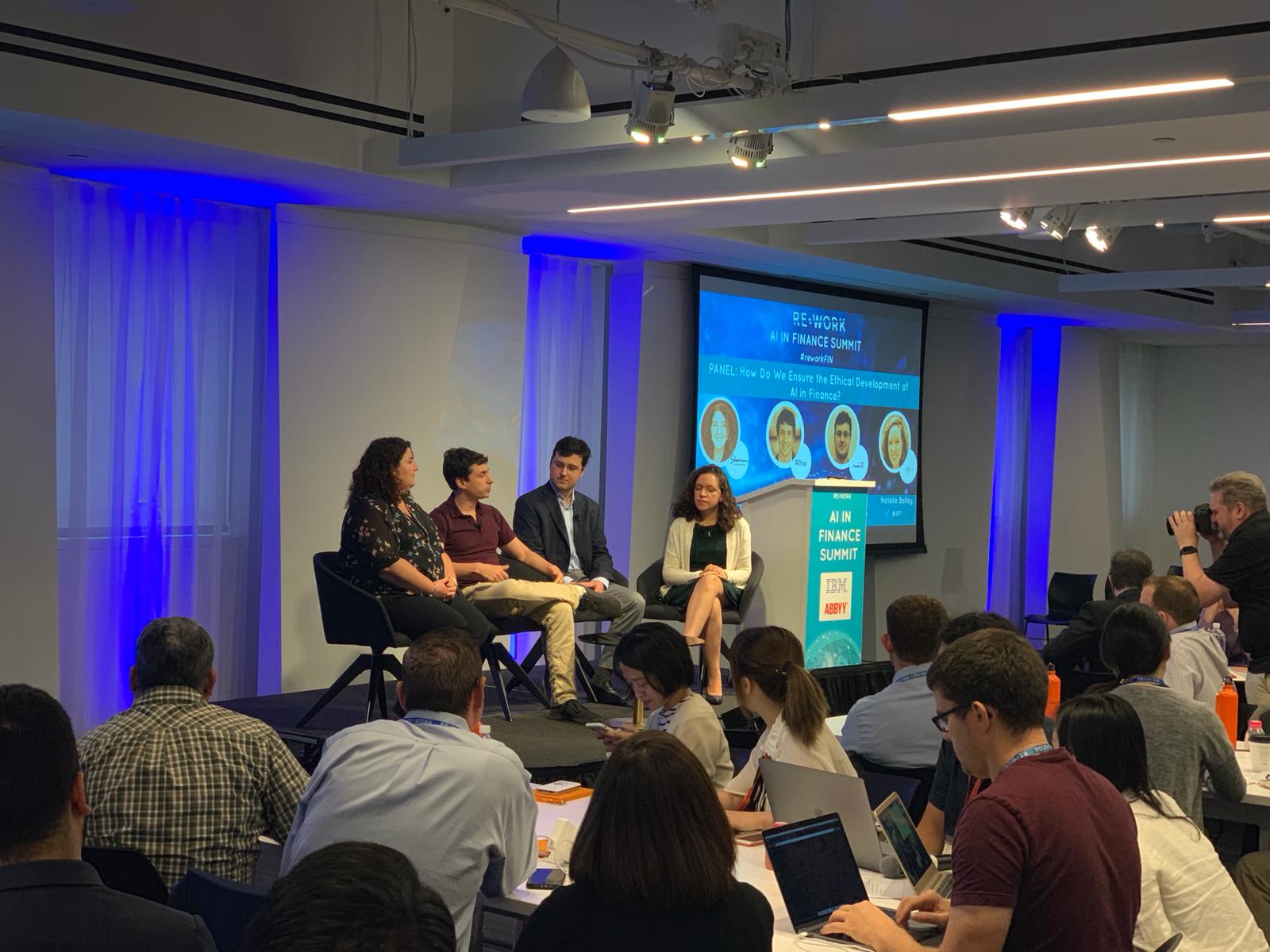
One of the topics that arose time and time again on both the finance and insurance tracks was ethics, bias and accountability. In this afternoon’s sessions we heard from Emma Maconick from Shearman Law, Aaron Roth from Penn State University and Zachary Hanif from Capital One on the panel ‘How do we ensure the ethical development of AI in Finance?’, which was moderated by IIF’s Natalia Bailey. To kick off the session Natalia asked what the most prominent risks in this space are:
Emma Maconick: I come at this from the perspective of 'how do we hold each other accountable?' in this space, the law is actually a solved problem, there's still a lot of work to be done from a regulatory perspective, but we're trying to look at the limitations of the algorithm and then look at responsibility and help you navigate the landscape.
Aaron Roth: There are various parts of decision making pipelines where we expect decisions to be made. As we're increasingly putting algorithms in here we should reasonably expect the algorithms to respect the ethical norms. Algorithms that are the outcome of ML pipelines are separated from the human doing the programming by several degrees, so when something becomes biased or unethical, who do we blame?
Zachary Handi: The most obvious risk is the lack of visibility. We increasingly become concerned if our models are accurately modelling what we 'think' they are modelling. We start seeing signals in areas where we didn't expect to see signals, and we now have to ask the question of why, this is concerning.
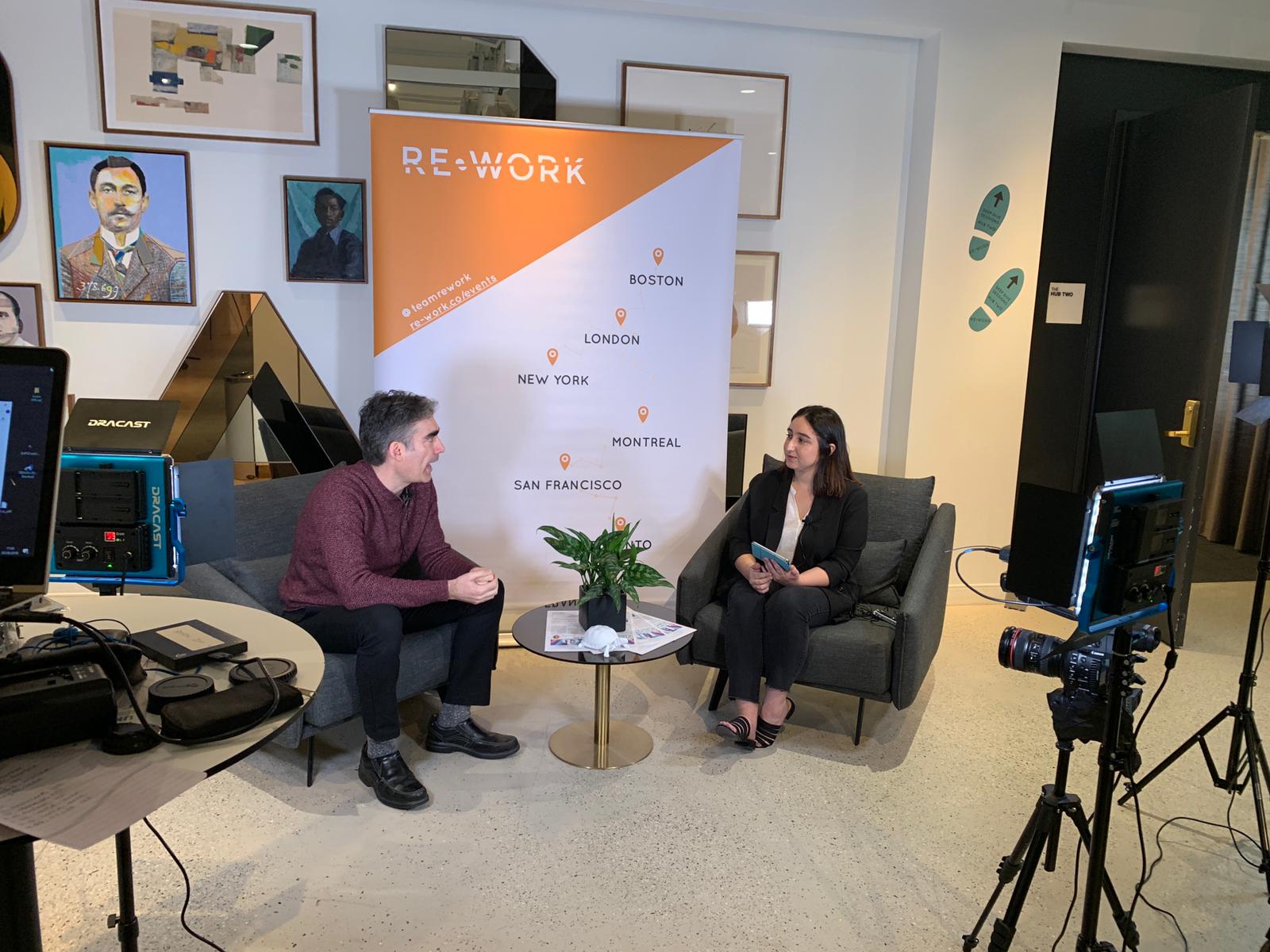
As well as presentations, workshops and panel discussions, several of our speakers joined RE•WORK in interviews, speaking in more depth about their experience. We were fortunate enough to speak to Marsal Gavalda from Square who delved into the ethics of AI in prediction systems often used in insurance policies: “At Square we train sophisticated machine learning models that attempt to map the name of a business (e.g., "Rolling Scones") into a business type (e.g., "bakery"). Interestingly, we found that in some cases the model would make different predictions based on the apparent gender of a person's name, e.g., "Eric's Shop" might generate a suggestion of business type "automotive services", whereas "Erica's Shop" might generate "jewelry and watches". Does this mean the model is "sexist"? Well, it does mean that in the training data, that is, in the actual population of sellers, there's some correlation between (gendered) personal names and business types, but is this "historical sexism" something we want to carry over to new predictions? Better not, so we decided to replace all personal names with a special token ("<PN>") during training and at prediction time, so that specific names no longer have any bearing on the business type. (And to be clear, if another word in the business name is characteristic enough of a business type it already negated the "sexist" effect, e.g., both "Eric's Bakery" and "Erica's Bakery" obtained "bakery").”
As the day drew to a close, everyone came together to share their experiences and learnings from the day in the networking drinks session. Here’s what our attendees had to say at the end of today:
“You thought AI was cool, this is AI IN INSURANCE, just wait!” Newcombe Clark, AIG
“For me, Marsal from Square talking about NLP was great. It’s really relevant in Insurance but it’s good to know the crossover to finance in more detail.”Mo Yad, DEEPQASH LLC