Adopting AI into your organisation is a challenging process and can require a clear strategy for successful implementation. That’s why we caught up with AI experts in our community and asked them the all-important question of ‘What advice would you give AI leaders who are looking to implement company-wide adoption of AI?’. Five AI experts, who will be presenting at the RE•WORK Berlin AI Summit on 4-5 October, have provided the best advice for adopting AI into your organisation.
Ertugrul Dalboy, Manager Machine Learning Engineering at ING
AI adoption is not any different than digital transformation projects. It needs to be part of the strategy and there needs to be a structured approach with a clear communication. Train employees to reskill or upskill them and train the business units to understand what they can do with AI. It needs to be blended in the business and not seen as a cost centre.
Sammer Puran, Data Scientist at Swiss Post
Make sure to have a wide understanding of AI in the company --> the change management from digitalisation into an ai data-driven mindset. Make sure to have a team of different capabilities to bring projects into products delivery and sales pipeline. Bored data scientists don't stay, but also overwhelmed data scientists quit.
Sarah Haq, Senior Machine Learning Engineer at Artsy
It really depends what stage of AI adoption a company is at, but I personally am very frustrated to watch talented Research/Data Scientists and MLEs spend months on projects that fail for various reasons.
Companies need to adopt agile data science methodologies to ship, learn and work iteratively to ensure the long term success of AI projects. For companies who have mastered AI adoption, they should be focusing on a robust MLOps platform to boost earnings from AI, as highlighted in this paper.
Eric Liese, Lead Architect and Advisor for AI & Analytics Infrastructure at BSH Home Appliances Group
1. Assess the maturity level of your company:
Identify the changes that need to happen to be ready to create, deploy and run productive AI use cases, i.e. company culture, organisational setup, people, processes, technology stack.
2. Find potential use cases with high impact and low effort
Aleksandra Kovachev, Data Science Manager at Delivery Hero
1. Invest in training people that are already in the company: Data Analysts, Junior DS, in addition to hiring talent.
2. Organize guilds, knowledge transfer sessions, give space and time for learning and experimentation.
4.Educate your Product people and C-Levels: AI and ML in real setting is more often failure than success.
5. And last but not least, start with simple models always, even heuristics and statistics is a great solution in most cases. Look-up to the Occam's Razor.
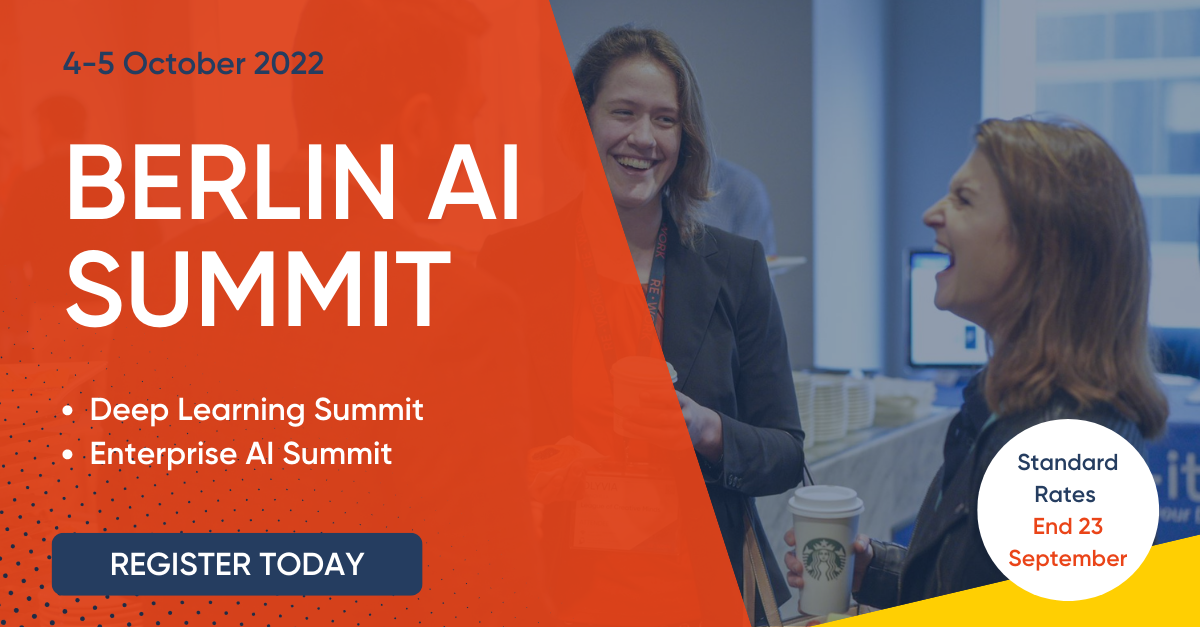
Did you enjoy this blog? You can join us at the RE•WORK Berlin AI Summit on 4-5 October to hear from these experts plus many more, where you can discuss the latest trends in AI. The Summit will see a number of topics covered including Deep Learning, Leveraging AI, AI Implementation, Cloud Computing, Meta-Learning and many more! Get your tickets.