It is key to ensure that, as an AI leader, your investments lead to success. Ahead of our RE•WORK London AI Summit, we caught up with some of our expert speakers and asked them ‘What are the highest priority areas for AI leaders to invest in for continued success?’. 14 AI experts have shared their thoughts. We’d love to hear your top tips for continued success in AI too!
Stephen O'Farrell, Machine Learning Scientist, Integrity & Safety at Bumble
Transparency, especially in terms of privacy in data. It feels like online privacy is quickly becoming a bigger concern for the general public. To an uninformed public, more scandals in AI will lead to a complete lack of trust in the field - which can eventually lead to overly heavy regulations. GDPR is a step in the right direction but I feel like leaders should still be doing more to let the general public know exactly how and why their data is used, opening discussions with policy makers and end users to show the benefits of ethical data usage and why access to certain forms of data are necessary.
Emmanuel Ferreyra Olivares, Principal Researcher – AI & Data Security at Fujitsu Research
AI technologies need to be trusted by people, embracing fairness, accountability, and transparency. Similarly, data are the core of machine learning algorithms. I think that for continued success, the high-priority areas for AI/Deep Learning leaders to invest in are:
- Trusted AI technology, including Explainable AI and Ethical AI
- Discovery AI to find causal inferences of large-scale data
- Automation of machine learning
- Transparent trust transfer technology to ensure data authenticity
- AI Cybersecurity
Huma Lodi, Senior Machine Learning Engineer at Sky
Casual AI is one of the high priority areas which now is becoming a focus of AI leaders. There are many crucial tasks in areas ranging from health care to global warming, where predictions from AI techniques are not enough to make an effective decision. Casual AI approaches enable automated complex decisions by identifying cause and effect. These techniques may also improve out-of-distribution generalisation and may enhance AI expressiveness.
Hastagiri Vanchinathan, Senior Director, AI at ShareChat
Apart from AI talent (which continues to be the no:1 high priority area), I look to invest in building the right frameworks, tooling, monitoring and alerting mechanisms.
Michael McTear, Emeritus Professor at Ulster University
Systems that are based on deep learning are essentially a black box where often not even the developer knows why a particular system behaviour has occurred. With commercial systems developers, providers, and customers want more control over the system’s behaviours and the ability to obtain explanations. Thus an important area for future development will be to explore methods for providing greater transparency. This is likely to involve investment in research into hybrid systems where the benefits of deep learning from large language models are enhanced with methods developed in symbolic AI to represent reasoning and provide explanations.
Florin Coman, Conversational AI Architect at Bosch Service Solutions
The top priority should be on proving that all the research and development in NLP/ASR/Computer Vision etc. have tangible application in real use cases to solve business problems. Everything has become very complex, but we have not yet seen the magic in AI that everybody is expecting.
Mike Hales, Digital Product Owner – Conversational AI & Contact Capability at BT
Machine learning, deep personas and style guides, and investing for the long term i.e. continuous development
Ross Parkes, Product Owner – Automation at HomeServe
I would see a high priority area should be to provide anyone looking to deploy AI into their organisation is to also invest in analytical tools alongside it, if you invest in an AI tool for your organisation it is imperative to invest in MI alongside it to help measure success.
Marion Riffaud, Data Scientist at Specsavers
There is a lot of potential in democratising data across a business to allow the wider audience to be data-led. This demystifies data and makes it more approachable for users that would not have had access to it previously. Of course, the data team should lead the way and help all the actors to ensure best practices, this can be by organising webinars, creating documentation, giving access to learning platforms to upskill the users interested in coding and offering an alternative drag and drop system for those that do not feel confident enough to code themselves. It is about empowering the users with data to make better decisions.
Rob McKendrick, Head of Data at Co-op
Investing in data frameworks that allow multi-vendor / multi model AI solutions to be applied to their business problems.
Stephen Jordan, Senior Data Scientist at Shopify
Solid infrastructure and tooling is critical. Your data scientists are expected to do a lot (moving data, deploy models, launch A/B experiments, etc) and providing the right capabilities to help them do their job will reduce friction and frustration.
Dino Bernicchi, Head of ML at HomeChoice International PLC
Data. People. Technology. While that may sound simple – it is deceptively complex. Leaders need to invest in their data strategy – a plan to create value from data. Actively investigate and test AI opportunities – collect, clean, and enrich data sources which support those opportunities. Invest in great people and be deliberate when attracting and retaining talent. Foster an inclusive culture that appreciates data. Leverage consultants to kick-start or accelerate your strategy. Invest in technologies like AI Platforms and cloud infrastructure – they increase worker efficiency and standardise the AI lifecycle meaning quicker prototyping and deployments. My answer here is a small summary of the 2-day data science executive leadership course which I lecture at the University of Cape Town’s Graduate School of Business. For more info, please visit 10Xdatascience.com.
Calvin Seward, Research Scientist at Zalando
The AI value creation chain is only as strong as it's weakest link, be it disorganized data, poor algorithms, bad monitoring or an uncomfortable UX. The highest priority is always the weakest link.
Yariv Adan, Director of Product Management at Google
Creating a quick path from ground-breaking research ideas, to useful, affordable, scalable solutions in productions.
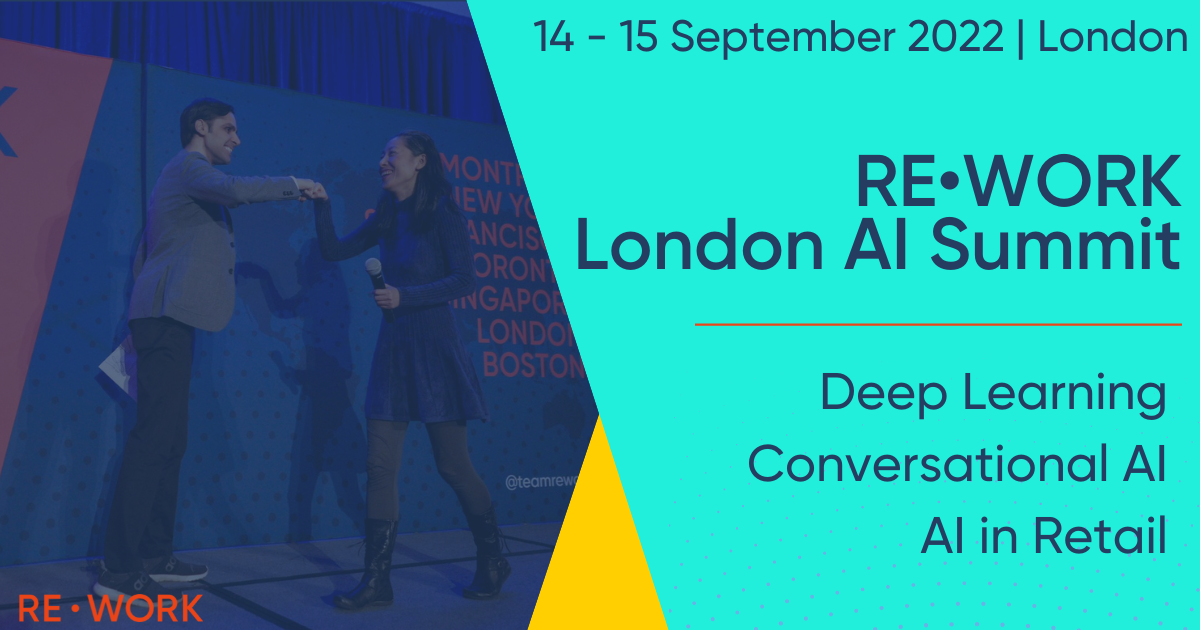
If you enjoyed this blog, check out the on-demand sessions from the RE•WORK London AI Summit that took place on 14-15 September, and hear from AI experts, including, Toju Duke at Google, Detlef Nauck at BT, Xin Wang at Shell, Claire Lebarz at AirBnb, Sunando Das at Unilever, Florin Coman at Bosch, Asmita Poddar at Amazon Alexa and many more! Get access to sessions from three unique tracks – Deep Learning, AI in Retail and Conversational AI. Get your on-demand pass today.