As humans, it is our responsibility to take care of the planet. It is our home, and the way we treat the environment has everlasting impacts on future generations. Over the centuries as we have moulded and manipulated natural resources, we have created some life-changing technologies, but have also created environments that have negative ramifications to our planet. We have the opportunity to act now for a positive change and transform the land, seas and air by acting responsibly with social and environmental growth at the core. This World Environment Day, we’re taking a look at how some global AI experts are leveraging technology for positive social impact to benefit the environment and the world.
The predictions of the future of our planet are bleak unless we make drastic changes. Both the UN and other bodies are aware that the protection and improvement of the environment is an issue deserving of much attention, so the celebration of today encourages all generations to do their bit for the planet and support initiatives going on across the globe. This June 20 - 21 in San Francisco, we will be hosting the AI for Good Summit where researchers, government bodies and business-people will come together to share their work in the space. Here are some of the experts who will be sharing their work at the summit:
Carlos Felipe Gaitan Ospina, Chief Climate Scientist & Head of AI, Climate AI
Presentation: Climate Zones Classification for Agriculture Applications using Machine Learning
Modern climate classification techniques typically employ intra-annual variability of climate data--e.g. temperature and precipitation--in order to segment geographical regions into meaningful zones with similar physical characteristics. For instance, common classification schemes like the Koppen-Geiger rely on human-defined clusters. Carlos is working on a generalizable method for creating meaningful climatically-relevant zones tailored to each user’s needs. To accomplish this, he first explored several clustering methods by comparing performances and weighing potential advantages and/or disadvantages to each technique. Carlos and his team found out that their streamlined clustering procedure can benefit the real state and agricultural sectors especially, by taking into consideration climate change conditions.
Carlos did his doctoral studies at the University of British Columbia (Vancouver, Canada) working with William Hsieh in machine learning applications in the environmental sciences. He also holds a Bachelor degree in Civil Engineering and a Master degree in Hydrosystems from the Pontificia Universidad Javeriana (Bogota, Colombia). He is a member of the American Meteorological Society’s (AMS) Artificial Intelligence Committee. He previously worked as a VP of Weather Forecasting at Arable Labs and as Research Scientist for the South Central Climate Science Center at the Geophysical Fluid Dynamics Laboratory (GFDL) in Princeton, New Jersey.
Pushpendra Rana, Postdoctoral Researcher, University of Illinois
Presentation: Machine Learning for Environmental Sustainability
The advances in machine learning are revolutionizing how we understand and design effective strategies to deal with forest and other natural resource sustainability challenges. Pushpendra is working on state-of-the art machine learning algorithms advance analysis of the social and ecological impacts of natural resource policies and generate evidence to prioritize governance actions to further environmental sustainability goals. He is also exploring reasons for slow adoption of automated decision making in natural resource management and conclude with possible directions for mainstreaming artificial intelligence and machine learning in forest conservation and management.
Pushpendra is an interdisciplinary environmental social scientist interested in evaluating and predicting the impacts of forest conservation policies at local and global scales to build knowledge on sustainable Social-Ecological Systems (SESs). He is particularly interested in exploring the use of AI and ML approaches in environmental sciences especially in the fields of policy impact evaluation, forest conservation and management. He also has experience in using advanced spatial analytical techniques, machine learning and causal inference based statistical approaches to explore research objectives in the field of environmental and forest governance.
Prabhat, Group Lead, Data & Analytics, Lawrence Berkeley National Lab
Presentation: Deep Learning for Climate Science
Climate change poses a major challenge for humanity in the 21st century. Characterizing the impact of climate change requires sophisticated analysis of complex datasets produced by climate simulations. In recent years, we have shown that Deep Learning based techniques are capable of classifying, detecting and segmenting extreme weather patterns in O(10) TB sized datasets. We have successfully scaled Deep Learning architectures to the largest CPU- and GPU-based HPC systems in the world. This talk Prabhat will review his latest results, and conclude with efforts to create ClimateNet - an open labeled dataset and reference architecture for collaboration with the broader community.
Key Takeaways:
- Deep Learning can be applied to climate science problems
- Analyzing large datasets requires HPC systems
- Access to labeled data is a key challenge
Prabhat’s group is responsible for supporting over 7000 scientific users on NERSC’s HPC systems. His current research interests include Deep Learning, Machine Learning, Applied Statistics and High Performance Computing. Prabhat has co-authored over 150 papers spanning several domain sciences and topics in computer science. He has won 5 Best Paper Awards, 3 Industry Innovation Awards, and he was a part of the team that won the 2018 Gordon Bell Prize for their work on ‘Exascale Deep Learning’.
Interested to learn from more experts working to leverage AI for Social Good? Join us at the summit in San Francisco this June 20 - 21 and hear from Google, UBM, Farmview, U.S. Dept of Homeland Security, World Economic Forum and many more.
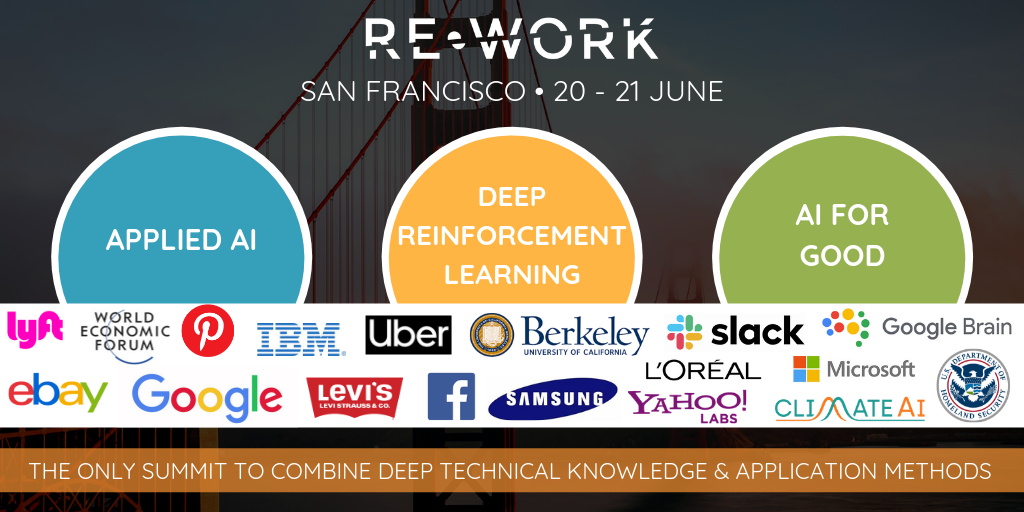