2022 was a massive year for the advancements in AI, particularly within the financial industry. But what are the challenges for AI in Finance this year? We caught up with experts from JP Morgan & Chase, UBS, University of Greenwich, Cornell University, and Fidelity Investments to find out about the top challenges that AI in Finance will face in 2023.
Adam McMurchie - Lead Data, DevOps & Cloud Engineer, Contractor
"Finance is the global hotspot when it comes to the world of AI, which has brought intensive scrutiny, some of the common core challenges are:
1. Regulation: The financial services industry is heavily regulated, and there are concerns about how the use of AI and machine learning will comply with existing regulations. Loopholes and exploits are a risk as well as grey areas where policy doesn’t yet exist.
2. Trust and transparency: There are concerns about the potential for bias and unfairness in AI and machine learning algorithms, and about the lack of transparency in their decision-making processes. This can make it difficult for customers and regulators to trust the decisions made by these systems.
3. Data quality and availability: AI and machine learning systems require large amounts of high-quality data to function effectively. However, the financial services industry often has challenges with data quality and availability, which can limit the effectiveness of these technologies."
Luke Vilain - Director of AI Ethics, UBS
"Increasing regulation - this will put pressure on firms to ensure processes and policies are in place, which may take time to develop and embed.
Exciting firms about data quality and data management - these topics don't always initially capture the attention of the c-suite, but we need investment and focus to ensure the data we're basing our algorithms on is of appropriate quality to be able to trust the resulting recommendations/predictions / decisionsML / AI engineering - developing capability surrounding our data scientists and data engineers to be able to improve the quality and speed of the build of algorithmic systems."
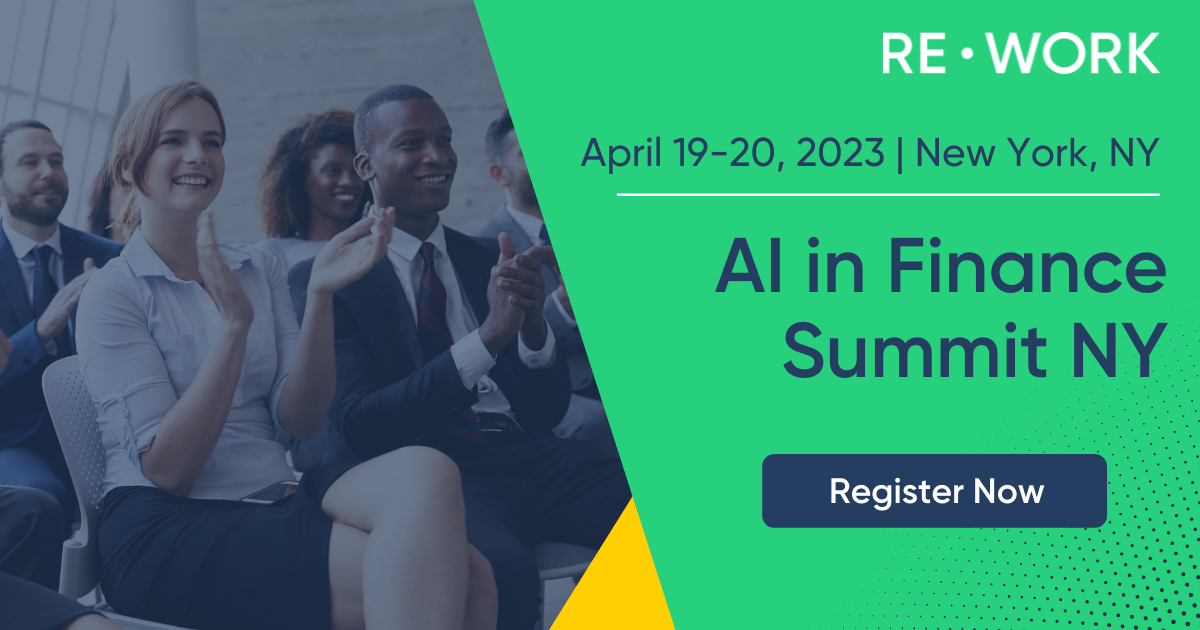
Georgios Samakovitis - Professor of Fintech, University of Greenwich
"Data sharing will continue dominating, with regulators’ attention still focused on data privacy in light of increased demand for consumer protection from fraud. Advances in privacy-enhancing technologies (PETs) point to the right direction, but the industry is nowhere near uniform or even reliable solutions.
The second challenge I see is in AI regulation and particularly explainable AI: especially where generative models become more integrated in the decision-making loops in Financial Services, more clear-cut approaches to interpretation for regulators and consumers will be required.
It would finally be impossible to ignore the challenges that DeFi brings, and, from among a long list, I’d pick as the top challenge to be the impact on risk modeling that comes from mainstreaming crypto assets with existing traditional asset classes. Requiring different infrastructure capabilities and introducing new affordances as programmable arbitrage vehicles, crypto assets should potentially be viewed as a transformative force in how the industry perceives investment risk-return relationships in the future."
Darian Nwankwo - Ph.D. Candidate, Cornell University
"I suspect the top 3 challenges with AI that the finance industry will face in 2023 is recruiting AI experts to finance, trying to address any moderately challenging problem with AI (deep learning in particular), and relying on the predictive power of these models without further investigation.
The predictive power of these models is fascinating, but our economy is maintained by the financial sector and we shouldn’t rely on these models as being the oracle of truth. We’ll have to ensure we keep humans in the loop, but the cost savings will be worthwhile as we develop diverse regimes of AI models working with humans."
Upal Sen - VP - Squad Lead/Product Owner AI, Fidelity Investments
"1. Scalability of AI solutions: As an industry, we need to make sure we do not create overfitted solutions to unique use cases. Instead, we need to stay focused on creating scalable AI services/ platforms that can help drive solutions across a wide range of use cases.
2. Working in the customer’s best interest: It is not just enough to create AI solutions that maximize profitability and revenue. We need to find a way to create solutions that drive the best outcome for our customers while building lifetime relationships with them. This is as much a business strategy problem as an AI/ML application problem. We also need to be cautious of inherent biases in our data and be able to correct for them. Balancing bias while driving business performance and acting in the customer’s best interest is going to be a tricky tightrope walk.
3. AI ML Ops: Rapid experimentation and fast time to market will continue to be a key differentiator for AI applications in our industry. Our AI solutions may often have complex optimization functions with a long-tailed feedback cycle. Making improvements in time to market in these complex applications will continue to be pivotal."
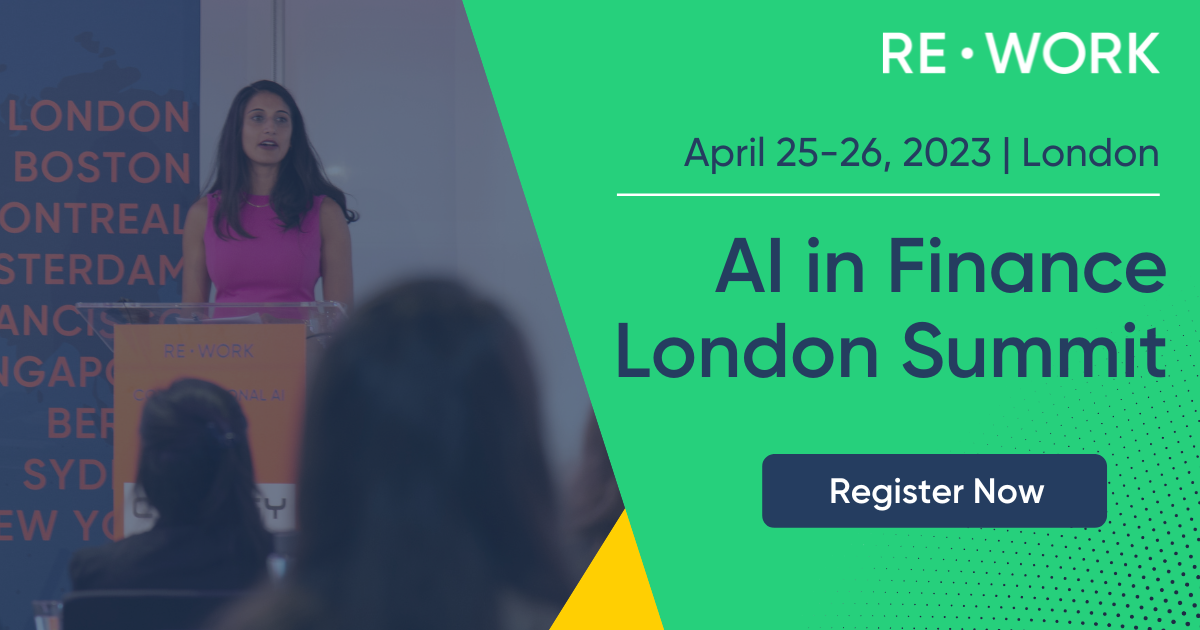
These leading experts will be joining us at the AI in Finance Summit New York on April 19-20, 2023, and AI in Finance Summit London on April 25-26, 2023, where they will be discussing the challenges of AI in Finance in more detail and how to overcome them.
Download the brochures for more information:
AI in Finance Summit New York Brochure
AI in Finance Summit London Brochure
Early Bird ticket sale for AI in Finance Summit New York ends on Friday, February 24, so secure your place today to save $500.
Early Bird ticket sale for AI in Finance Summit London ends on Friday, March 3, so secure your place today to save £500.