Not able to join us for the Virtual Applied AI Summit earlier today? Don't worry, we've got you covered.
We've gathered some of the highlights from the summit, including quotes, models and explanations around the latest technical insight and business case applications of AI.
The World Economic Forum Focussing on Ethical AI Practice
Up first, we hosted Kay Firth-Butterfield, Head of AI & ML at the World Economic Forum who discussed the world of ethics in AI and why it is so important that set guidelines are adhered to. The session focussed mainly around trust and the importance of public understanding and acceptance, not only for brand security but for wider perception.
“If you’re not creating trusted AI, then you’re going to have a loss of brand value if something goes wrong"
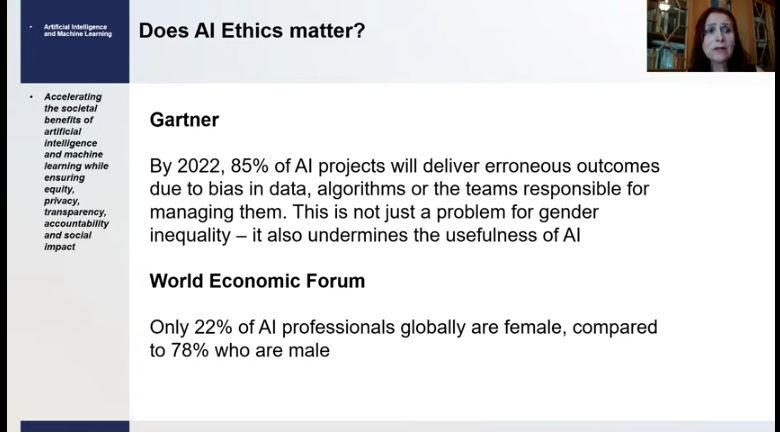
“We found that board members didn’t know very much about AI, so we wanted to make sure they also had a toolkit, so could properly advise to the C Suite”
Kay then went on to explain that ethical AI in itself needs a lot of testing, circling back to the idea of trust. The idea that AI can be used for anything at any time is fast becoming redundant due to the need for many studies, peer reviewed works and more to ensure efficacy.
Kay's talk was later followed by a Q&A with attendees:
Q: "If possible, should companies employ a Chief AI Ethics Officer?"
A: Yes, definitely. AI fits into each of the ESG buckets. As I explained at the beginning of my talk not getting ethics right is very bad for brand but also for use of your algorithms.
Q: Moral philosophy is complex and cultural. Do you think k12 moral philosophy education should be mandatory so developers of AI instinctively have a code of conduct?
A: I agree. I think teaching these concepts to all is important. My own view is that AI ethics needs to run through all university AI courses at all levels. However, consumers also need to understand these concepts so teaching everyone at school would be great, not necessarily philosophy but definitely understanding how the devices used work and why the 10 area I mention in the talk matter.
AI Developments at eBay
On finishing the Q&A, Kay was followed by Tom Virant, Director of Data Science at eBay. Tom opened by asking the question, "How do you deploy and manage not just 1, but 100’s of machine learning models? What changes when you move from batch processing to synchronous calls? When your algorithms become mission critical, what changes?"
What is seemingly easy when working for a small company, with lower volumes or with batch operations becomes exponentially more difficult at scale. Off the shelf development and deployment platforms have existed for years, but use cases are becoming broader and corporations are starting to customize and invest internally. In this presentation, I will discuss the infrastructure and processes necessary to operationalize data science at scale.
Early in Tom's talk he discussed the development strategy at eBay, suggesting that rebuilding models on production data is the way to go if you're not adding new features. Furthermore, eBay build everything in python, to later deploy it in Java. Why? Tom suggested that this was in part to try and minimize developer work when possible from build to deployment, which then leaves the ability to code for performance when necessary. When building and deploying at scale it was stated that eBay has massive internal systems to scale, with their production system running 500 transactions a second, for example. Tom then detailed the four main requirements in building & deploying models at scale, which are Data Management, Tech Functionality, Governance & Monitoring.
“Data is complex, the whole data management process is difficult focus & simplicity”
Q: How do you manage multiple languages and scalability?
A: Al builds are in python today and we keep a data repository too. eBay has massive internal systems to scale for us, our production system runs 500 transactions a second for example, this scales to multiple systems and is fairly easy at eBay.
Q: What was your strategy to handle data distribution shift due to COVID?
A: We monitor our apex sellers due to COVID. For us, we don't handle distribution shift but we adjusted our models. We saw increased perceived risk due to shipping issues, but this was more of a strategy re-think and not a shift in our models.
DeepBrew - AI at Starbucks
Up until a few years ago, Machine Learning at Starbucks wasn't even considered.. At first, we were drawn in by the hype of ML, Brian suggested, however, the reality of MLs advances were evident. You could get blocked from an ML initiative only 3 years ago for saying that a problem was too complex. In 2016, when AI defeated GO, this argument started to lose it's validity. This accompanied by careful projection of future trends meant eventually establishing ML at Starbucks, so as to not become yet another company that fell behind the times:
“The leadership at StarBucks realized that, if the world is changing, even something as beautiful as StarBucks is at risk, so we decided we would invest in all the elements required to deploy ML”
Brian suggested that through data collected from tens of millions of users who share what coffee they like, when they like it etc, Starbucks are able to take that data away and put it in their EDAP platform, processing it to use it to build solutions in Java or Python to speak to each touch point with highly personalized experiences. Brian and his team are 'hyper focused' on building a “brain” at Starbucks, that will personalize the experience of 10s of millions of customers in under 200ms for each request.
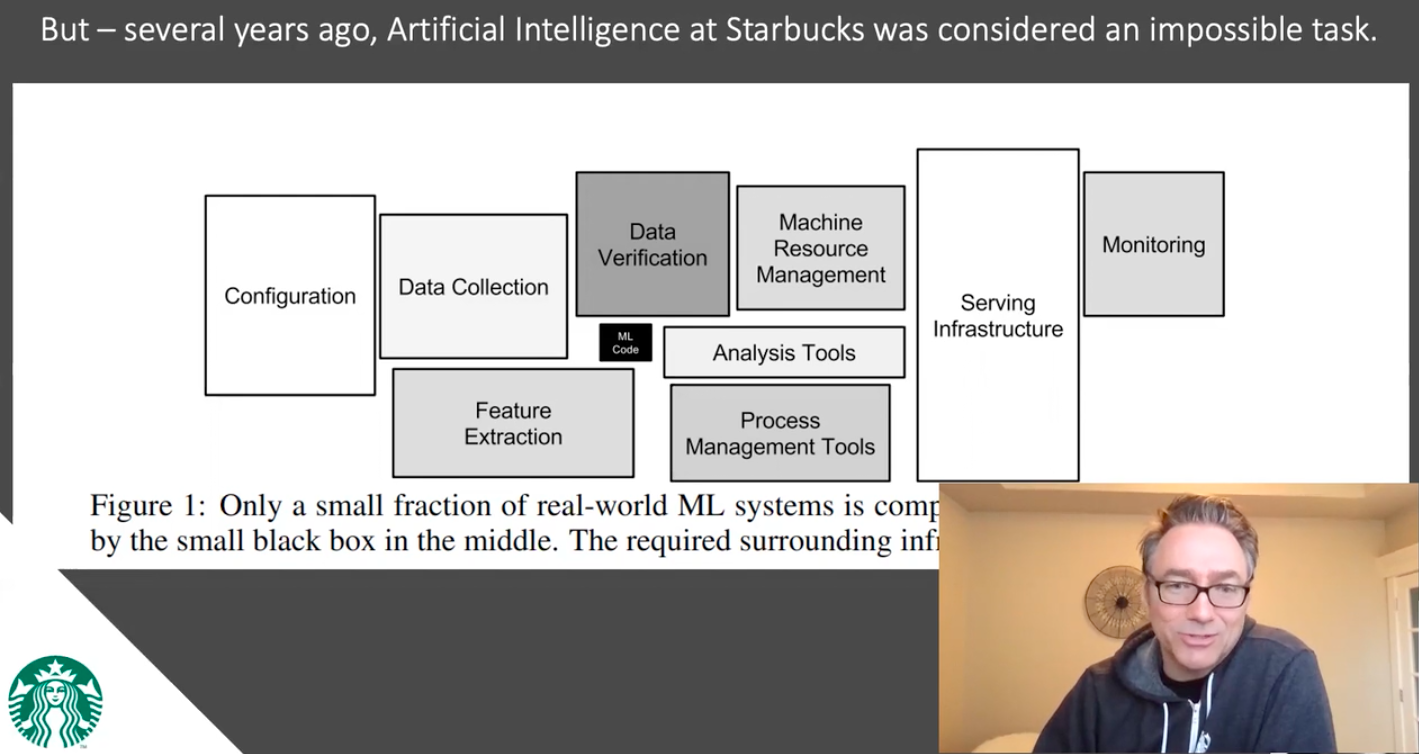
"It’s actually an amazing challenge. I have lots of hopes and dreams outside that, but it is our sole focus here"
Q: What specific problems do you solve with AI at Starbucks?
A: We have RL based recommendation systems running in the mobile app, inventory prediction, IoT for predictive maintenance, supply chain & many more in the works.
"We see ML as a team trying to make it easier for customers to have a great experience & personal interactions"
Q: What are the key platforms/tools( R, Python, etc) and environments (AWS, GCP etc..) used in your team to build the end to end AI solutions?
A: When asked the tools that they are using at Starbucks, Brian replied: GitHub, DataBricks, Azure SQL, Splunk, New Relic, PagerDuty, PowerBi and more.
Lack of Training Data? No Problem!
CEO of AI Reverie, Daeil Kim, then gave a talk on solving hard problems in Computer Vision with Synthetic Data. We had a chance to discuss Daeil's product at AI.Reverie, which is said to cut the cost of training while improving the quality, diversity, and accuracy of metadata. Daeil suggested that what really distinguishes them from other products is that AI Reverie makes synthetic data the horizontal focus across industries and their obsession with proceduralism, or designing everything so it can be scaled automatically.
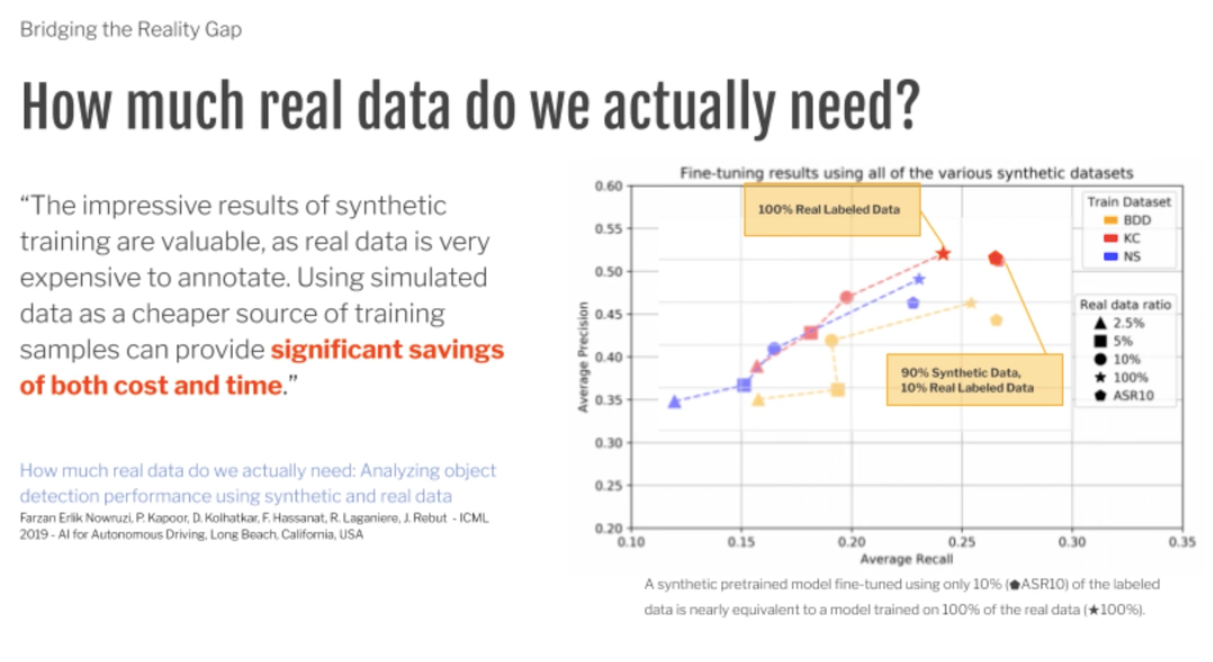
Daeil suggested that they knew early on that solving the content generation problem at scale, then we could solve many computer vision problems across a vast range of applications.
Some key quotes from the presentation included:
“For us we’ve taken the approach of coupling our procedural tools with real world data to help offset bias that occur in simulated environments”
"In the future we think that 90% of the data you need can be replaced with synthetic data”
“In general, we feel there are 3 major bottlenecks associated with getting good data, the 1st is getting the data itself the 2nd issue is annotations being mislabelled the 3rd & most critical is the overall labor costs”
ML Solution Development at Walmart
A good ML solution often takes a year to develop and can sometimes take several years according to Tian Su, Director of Machine Learning at Walmart. That said, Tian was also conscious that in the current climate, especially in retail, six months can seem a long time for development to those not fully involved.
"As leaders in this field, we want to try and strike a balance between speed of rollout and acceleration and quality"
“One way I found useful to accelerate ML solution development was to find a commonality across the portfolio. When I joined Walmart, I found that this was anomaly detection”
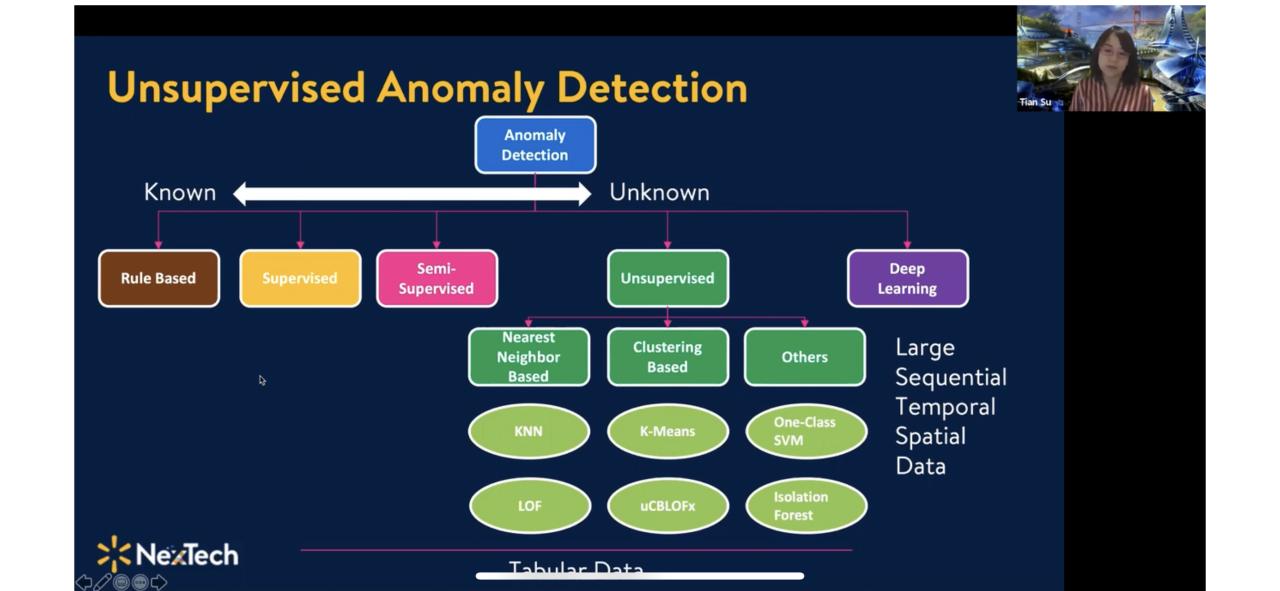
Whilst recognizing that many algorithms can be scaled with minimal disturbance, fraud detection was noted as one of the very clear use cases where you see the shifting of anomalies due to their development of new ways to cheat the system, meaning that you need to continually update your model.
Notable quotes from throughout the day:
Whilst we could cover every discussion of the day, we realize that may make this a little long so we decided to collate some of our favourite quotes from the presentations today.
"Complimentary recommendations are products that go together & have a similar form and function as the anchor product If someone buys a bathroom mirror, we’ll recommend a toilet, basin & cabinet that have a similar form. If two items are in a collection together they will get a positive label of 1 in the training data, and we randomly collect items that are not in the collection & label them with a negative label” - Simon Hughes, Senior Data Scientist - The Home Depot
“Computing power is basically the most important thing when it comes to building AI solutions because the reason those models become so big & grow really fast is the computing power. We can assume that every cloud user has a dedicated chip you want to be able to orchestrate workloads on the right type of accelerator on different processors — the PPU can be beneficial in certain scenarios, but not for every scenario. You really need a system that knows how to orchestrate the workloads on a GPU or PPU" - Omri Geller, Co-Founder & CEO, Run.ai
“Gartner suggested that ‘by 2023, over 30% of operational warehouse workers will be supplemented by robots’, which i am not surprised by. The challenge faced by Sam's Club is monitoring the inventory sitting in each 'bin'. If we could have some type of bot/AGB or a drone which can move down these isles in off peak hours & create that work-list for you..that is the problem we are trying to solve” - Ravi Dalal, Senior Computer Vision Engineer, Sam's Club
"We built an infrastructure which allowed us to record sound, process it in real-time and send alerts to partners on the ground. Detection post-analysis, in a nutshell can automate this process, recognizing chainsaws for de-forestation for example" - Bourhan Yassin, COO, Rainforest Connection
"You might think it's for healthcare, but surprise, it's not. The most common use of robotics during this lockdown and quarantine is crowd control and enforcement! The second most common use for robotics at this time is clinical care, covering disinfecting, patient intake and more!" - Robin Murphy, Director, Texas A&M University
“The typical development cycle of an ML Engineer has a lot or repetitive steps. Ideally the process would be much simpler which is exactly the goal of automated model exploration; To automate standard automation practices, to democratize AI & speed it up to make an impact” Sandeep Jha, Staff Technical Program Manager, Linkedin
With that, we'd like to extend a huge thank you to all of our attendees and speakers, as well as our sponsors Run.ai, AI.Reverie, RapidMiner, Diffgram & cord.
Interested in reading more leading AI content from RE•WORK and our community of AI experts? See our most-read blogs below:
Top AI Resources - Directory for Remote Learning
10 Must-Read AI Books in 2020
13 ‘Must-Read’ Papers from AI Experts
Top AI & Data Science Podcasts
30 Influential Women Advancing AI in 2019
‘Must-Read’ AI Papers Suggested by Experts - Pt 2
30 Influential AI Presentations from 2019
AI Across the World: Top 10 Cities in AI 2020
Female Pioneers in Computer Science You May Not Know
10 Must-Read AI Books in 2020 - Part 2
Top Women in AI 2020 - Texas Edition
2020 University/College Rankings - Computer Science, Engineering & Technology
How Netflix uses AI to Predict Your Next Series Binge - 2020
Top 5 Technical AI Presentation Videos from January 2020
20 Free AI Courses & eBooks
5 Applications of GANs - Video Presentations You Need To See
250+ Directory of Influential Women Advancing AI in 2020
The Isolation Insight - Top 50 AI Articles, Papers & Videos from Q1
Reinforcement Learning 101 - Experts Explain
The 5 Most in Demand Programming Languages in 2020