Training data and the lack thereof is often one of the most commonly cited roadblocks in AI, both in regard to scaling & testing. Whilst many are aiming to address this, AI.Reverie are a standout organisation due to their horizontal focus across industries and obsession with proceduralism, or designing everything so it can be scaled automatically. We caught up with AI.Reverie co-founder and CEO ahead of his presentation on 'Solving Hard Problems in Computer Vision With Synthetic Data' at the Applied AI Virtual Summit.
Interview below with Daeil Kim, co-founder and CEO of AI.Reverie.
For some time now you have either been working or studying in the field of AI & Computer Science. What was it that initially sparked your interest in these areas?
I’ve studied a variety of subjects throughout my life, so I might not be a typical technology founder. In fact, during my undergraduate years I was primarily focused on the humanities, especially literature. My interest in literature led me to study psychology and volunteer at a few cognitive neuroscience labs, which then led me to a research job studying schizophrenia from fMRI data. Following this, I really became interested in understanding the nature of intelligence. I applied for an NSF grant that allowed me to enroll in a doctoral program in computer science, studying under my advisor Erik Sudderth, who was a computer vision professor at Brown at the time. At the end of the day, the nature of intelligence - human or artificial - still fascinates me, and that seed grew into AI.Reverie.
Prior to becoming founder of AI.Reverie, you had utilised ML ops, full-stack ops and APIs. Do you think that this practical implementation of algorithms, alongside your already extensive theoretical knowledge, created a solid foundation which allowed you to build AI Reverie?
After my PhD, I spent a few years trying to build innovative machine-learning based products at The New York Times. One product I worked on, first known as “Readerscope” but now called “TAFI,” has helped The Times continue performance marketing without relying on Facebook and Twitter pixels, which supports an organizational push towards greater data privacy for readers. It was a great experience in regards to getting familiar with the various technologies that are required to build a product driven by ML, and it’s certainly helped me in shaping the way I architect things.
In general though, I personally believe there is great value in building useful tools that can last. It’s worth considering how certain inventions can become a powerful catalyst for new science. Think about what the microscope did for biology or the invention of MRI (magnetic resonance imaging) for neuroscience. If you’re driven to build such tools, you’ll often figure out on your own the best way to create them. Though that experience of working with dev tools was valuable, I really wonder if AI.Reverie would have happened if the drive to create meaningful tools were never there.
Before we start talking about the product, can you tell me a little more on AI.Reverie, where the concept came from and when you decided to take the leap into creating a startup?
During my PhD, I was exposed to a lot of problems that typical practitioners face in this field and one that primarily stood out to me was the general lack of training data. The idea that we were still using this old way of collecting images and labeling them seemed very inefficient to me. Another thing you realize about academia, which I have a lot of love for, is that the papers you publish are often drowned out by the hundreds of other papers that are out there. I knew early on that I could probably publish work in this area or make a great github repository for something useful, but what you really need to prove that synthetic data works is to create something that could be commercialized and used at scale. What led to the possibility of this becoming a startup was meeting my co-founder Paul Walborsky at The New York Times and having him seed those ideas of entrepreneurship in my mind, being a former entrepreneur himself. In regards to the concept, it’s a sentimental one. The word ‘reverie’ is defined as a short sweet daydream, and I became enamored with a future where intelligent systems are first trained through the daydream of a simulation before they are released into the world to do our bidding.
AI.Reverie was founded back in 2017 - what did you find to be both the initial roadblocks and early successes when building the brand?
The initial roadblocks were definitely convincing investors of a technology that was quite foreign to them at the time without any precedent for a business model that worked in this field. We were sometimes successful at convincing them that the technology made sense and that the paradigm of the old ways of training computer vision needed to be fixed, but the hardest part was finding investors who believed we could commercialize it to make it a strong business model. I also have my co-founder Paul Walborsky to thank for believing in such an ambitious dream and helping make this a business reality as he’s been essential in making us the viable business that we are today.
In regards to early successes, a significant advantage we had was working with the government, who were often the first customers that understood the need for this technology. We also have them to thank for helping us stay afloat when commercial enterprises were still struggling to understand the benefits of synthetic data.
Your product is said to cut the cost of training while improving the quality, diversity, and accuracy of metadata, do you believe you have found not only one of the first products in this area, but also one which could solve one of the largest issues we have seen in recent years for AI (lack of training data)?
I would say what really distinguishes us from other products that try to do synthetic data is our horizontal focus across industries and our obsession with proceduralism, or designing everything so it can be scaled automatically. We knew early on that if we solved the content generation problem at scale, then we could solve many computer vision problems across a vast range of applications. In other words, if you build an amazing city that is rich with metadata, you could use it for self-driving cars, delivery robots, and a myriad of other important urban challenges. We took an approach towards proceduralism and content generation that was flexible enough for many verticals, so our product is unique amongst others in servicing a wide variety of computer vision challenges.
With the brand already in-demand, can you touch a little on the scaling potential of the product?
I would say scaling is inherent in the products we build, because it’s simply a matter of running the GPU to create more annotated data. Perhaps the interesting part here is really in scaling to still more verticals, and that’s something we’re getting better at every day as we learn how to generalize different parts of our procedural tools for use cases we had never imagined before partnering with some of our clients. Of course, more important than that is ensuring that the quality of data is high enough to be useful for training vision algorithms in the first place. So first, increasing the general fidelity of our synthetic data and second, adapting our tools towards a horizontal focus has helped shape our product in ways that are advantageous in a market that is still maturing.
You currently have projects in Defense, Retail, Smart Cities, Industrials and Agriculture including airport simulation, weapons detection, cashier-less shopping and delivery bots. Are there any other areas you are hoping to break into this year or in the near future?
We’re quite happy with the number of verticals that we’re tackling, but we are getting all sorts of new projects every month that go beyond the use cases we might have initially imagined. Funnily enough, when we started we wanted to stay away from self-driving cars since most competitors were focused on creating simulations there, and we wanted to show the world what you could do in other areas that have often been ignored. Now that we’ve created several rich city environments for some of our clients, we can easily leverage that to create training data for self-driving cars as well. So the way we tackle new verticals is really more about using we’ve already built and our capabilities at the moment and determining how easy it would be to shift that into a new application without incurring a huge development cost.
Ethics in AI and Machine Learning has become very prevalent and somewhat of a buzzword - how have you addressed potential ethical hurdles when growing as a business?
It certainly is quite the topic, and there are many great conversations going on in that space, such as the ethics around the design of products built with peoples’ data as well as concerns around privacy and civil liberties.
I try to be transparent around my own personal ethics and what I ultimately care about. It helps shape what we’re willing to work on and not. I can spend hours conversing about it, but in general, I make it clear to folks that I believe the great promise of AI is tied to figuring out ways we can guarantee the basic needs of human society. I believe that a lot of suffering in the world is tied to the inability to secure the basic physiological needs of ourselves and our loved ones, essentially our inability to satisfy the base of Maslow’s hierarchy of needs. When we don’t feel secure around those things, human beings have a tendency to take desperate measures to achieve them and there needs to be some compassion for that. Policy is certainly an important way to mitigate these issues, but so is making essentials such as food and water cheaper to acquire through technology. It can make otherwise infeasible policies feasible and there is value in building things that can help shape this reality in the future.
I think there is also an interesting discussion to be had on claims around the neutrality of technology, but it seems that’s often brought up to bypass the need to discuss the ethics in the first place. I believe it behooves technology leaders to read and understand the history of our world beyond a technological lens. Reading someone like Paul Farmer who talks about structural violence in Haitian societies and the ways they reinforce cycles of poverty can help shape what technology should ultimately be used for. As much as there is a race to create a general artificial intelligence and self-driving cars, the greatest AI systems may address more basic problems, such as an unlucky mosquito bite or a lack of access to basic sanitation.
This is another reason why I wanted to create a technology that could scale across several verticals. In light of that, I’m personally excited about our work with Blue River technologies in helping them solve problems in agriculture through computer vision. It aligns closely with my drive to build a more utopian future where robots help provide for our basic needs.
Just last month it was announced that you had secured funding led by Vulcan Capital. Can you tell us a little more about this process that led to securing funding?
Vulcan was actually an early investor for us and we have loved working with Yongbai from Vulcan over the years. It was a wonderful opportunity for us when he was willing to lead our most recent round since he was someone we trusted. As you know, it’s very important to have the right members who believe in your vision when it comes to bringing folks onto the almighty board.
Are there any projects or releases you have coming up that you can tell us about?
We’re getting pretty close this year to generating within days large open world environments that reflect real world locations at a 1:1 scale. There is an awesome engineering challenge involved in taking all that real world data and making it into a fully parameterized simulation environment that can span hundreds of kilometers. A lot of the procedural tools that we’re building are getting to a point where it can be used to recreate a lot of the world around us and we hope to showcase some of the speed with which we can accomplish that within the next few months.
What are you looking forward to in 2020 for AI.Reverie?
We want synthetic data to become the default input when it comes to training vision algorithms. It feels like this year, based on our results to date, the conversations are finally turning this way.I’m excited to see the amount of interest in this area from customers and other researchers as well. I think this will be the year for synthetic data and it feels wonderful to be part of the journey to help evangelize its use.
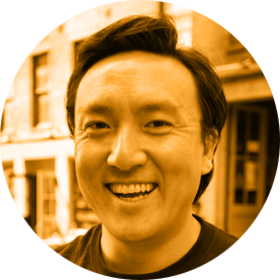
Daeil Kim is co-founder and CEO of AI.Reverie, a startup specializing in creating high quality synthetic data to train computer vision algorithms. Daeil received his Ph.D in Computer Science from Brown University focusing on scalable machine learning algorithms. He is excited about building tools that will help advance machine learning progress and considers synthetic data to be a core element towards advancing that field.
About AI.Reverie
Training computer-vision AI to be accurate and nuanced requires diverse, complex annotations — a lot of them. To solve that problem, AI.Reverie creates a virtually endless supply of data through a unique synthetic data platform for computer vision and machine learning applications, in order to lower the cost of training all while improving the quality, diversity, and accuracy of metadata. Learn more at aireverie.com.
Interested in reading more leading AI content from RE•WORK and our community of AI experts? See our most-read blogs below:
Top AI Resources - Directory for Remote Learning
10 Must-Read AI Books in 2020
13 ‘Must-Read’ Papers from AI Experts
Top AI & Data Science Podcasts
30 Influential Women Advancing AI in 2019
‘Must-Read’ AI Papers Suggested by Experts - Pt 2
30 Influential AI Presentations from 2019
AI Across the World: Top 10 Cities in AI 2020
Female Pioneers in Computer Science You May Not Know
10 Must-Read AI Books in 2020 - Part 2
Top Women in AI 2020 - Texas Edition
2020 University/College Rankings - Computer Science, Engineering & Technology
How Netflix uses AI to Predict Your Next Series Binge - 2020
Top 5 Technical AI Presentation Videos from January 2020
20 Free AI Courses & eBooks
5 Applications of GANs - Video Presentations You Need To See
250+ Directory of Influential Women Advancing AI in 2020
The Isolation Insight - Top 50 AI Articles, Papers & Videos from Q1
Reinforcement Learning 101 - Experts Explain
The 5 Most in Demand Programming Languages in 2020