According to Gartner, Inc the number of companies implementing AI has increased by more than 270% in recent years. With this increased adoption organizations will need to build their own AI teams to overcome the challenges and drive business growth.
Ahead of the New York AI Summit in April 2022, we interviewed our panel of expert speakers about their approach to building out AI teams, learning what traits they prioritize, how effective teams are built, and any secrets they have for accomplishing both. Included are contributions from Octane, Mutual of America, and Finicity, by Mastercard.
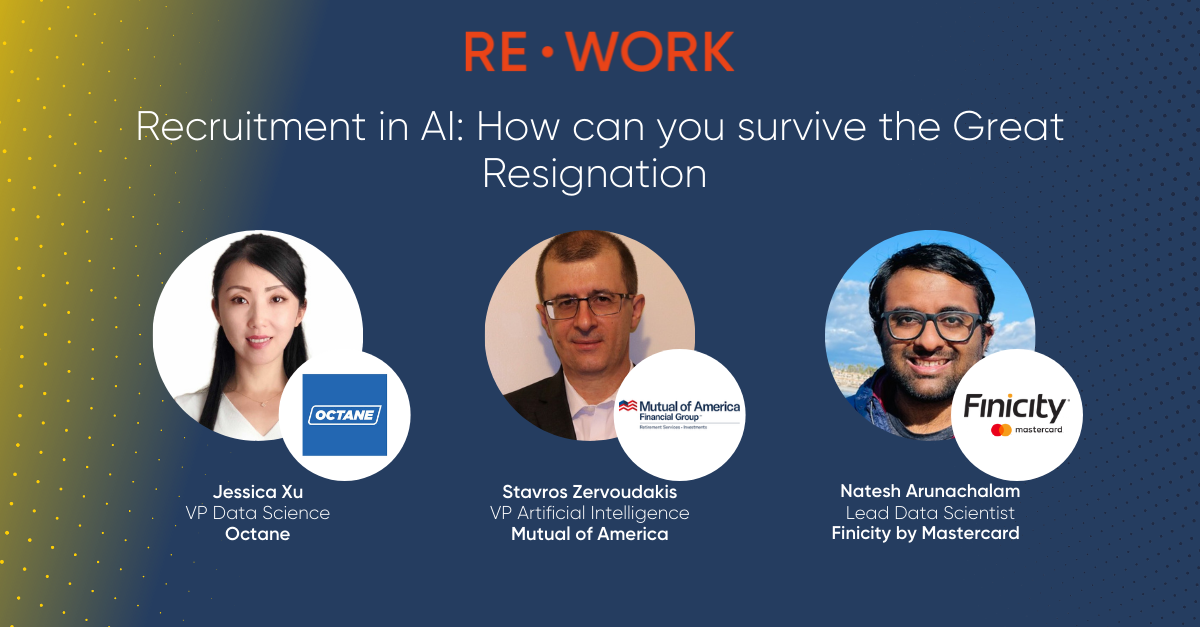
Experience vs. Agility: Which do you prioritize and why?
Jessica Xu, VP Data Science, Octane
As we grew our AI team by 300% over the last six months, I prioritized candidates who were versatile and agile. At a high-growth company like Octane, the AI team needs to quickly run experiments, test different hypotheses, and select and implement the best solution. Since business challenges and priorities are dynamic, the AI team needs to be agile enough to adapt and respond effectively to changes.
Stavros Zervoudakis, VP Artificial Intelligence, Mutual of America
Both are important but experience in leading projects and programs, in AI and outside the realm of AI, is proven to be a key differentiator. I believe agility needs to be a given, along with a continuous-learning, constructive, team-player attitude. Without agility and attitude, it is a no-starter for candidates and for hiring managers who wish to succeed. However, leadership experience and getting-it-done attitude, brings the team over the tipping point for success.
Natesh Arunachalam, Lead Data Scientist, Finicity by Mastercard
This is an interesting tradeoff that needs to be optimized based on the size and maturity of a data science organization. At early stages of a product or company, agility would be more important as rapid experimentation will be necessary.
What’s the secret to building an effective AI/ML Science team?
Jessica Xu, Octane
Building an effective AI/ML Science team requires three key things. First, build a diverse team. When your team has members of different backgrounds, perspectives, and experience, you will be more likely to approach problems in innovative and unique ways. Second, encourage both individual ownership and cross-team collaboration. Make collaboration essential but in the same time enable each member to develop individual areas of expertise and accountability. Third, listen. Lead by example and encourage team members to share ideas, offer feedback, and ask questions, and ensure team members feel heard.
Stavros Zervoudakis, Mutual of America
A problem solving, solution-oriented leader with both technical know-how and mid/senior level multidisciplinary management experience, along with the ability to identify business needs that AI/ML can address. Also support from the top in building the vision and during implementation of the strategy for all business verticals, involving end users at all levels from the start of each project and learning from them. You will also need a good-sized team with members who can wear multiple hats (each member being a 1-person AI/ML technology unicorn), metrics to determine returns to business, MLOps and SolutionOps pipeline automation and monitoring, selecting a set of projects that can have high visibility and potential for good returns, a budget to pay higher than for typical leadership and development work, positive and appreciative feedback to team members, and a bit of patience, to name a few.
Natesh Arunachalam, Lead Data Scientist, Finicity by Mastercard
Data scientists are a very curious bunch. It is crucial to ensure that each data scientist is continuously learning and expanding their own intellectual boundaries.
Is self-service AI changing how you recruit?
Stavros Zervoudakis, Mutual of America
Not today. It is a useful skill set to be familiar with auto-ML, which I include in my course at New York university, but relying on self-service AI only can be a costly approach with low to no returns. Candidates need to have a good understanding of what is under the hood, how to work with data and models, how to experiment and how to create an AI-based business solution, how to drive adoption and teach others. In Finance, compliance and other constraints make self-service even more challenging, as it requires post-processing and specific guardrails. Having said that, taking candidates through painstaking data science and ML tests and using (recently popular) laborious technical interview processes is not recommended at all, since it creates a negative taste for your new hires, before they even get to go through the door.
Want to learn more from AI experts? Check out the RE•WORK London AI Summit, 14-15 September 2022.
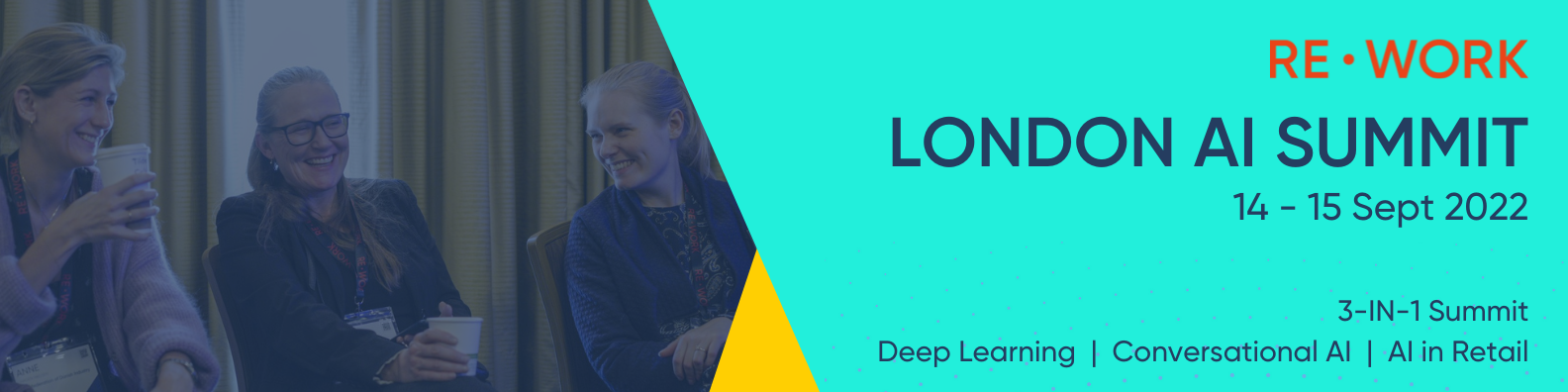
Join us to hear the secrets to successful AI implementation and gain practical insights from the world's leading AI innovators, from companies such as Google, Amazon, BT, Expedia, BP, Airbnb, Snap Inc. and many more.
The RE•WORK London AI Summit consists of 3 unique tracks; Deep Learning Summit, Conversational AI Summit and AI in Retail Summit Summits. Attendees can join sessions across all 3 tracks with just one ticket. There are now a limited number of tickets available so book your ticket here to secure your place.
For more information contact [email protected].