We had the pleasure of speaking with Emily Bailey, Data Science Manager at Uber, for an episode of our Women in AI Podcast Series. Emily, who is now leading data science for Uber's loyalty and subscription product, discusses the glass ceiling in the working world for women and shares her advice and personal experience on the steps taken in her journey into data science.
Read the full podcast transcript below.
Topics explored include:
* COVID Recovery Strategy
* Large Scale Business Forecasting
* Successes and Failures within the Business
* Model Modification
* Female Role Models
* Management Strategies
* Women in Data Group at Uber
Charlotte RE•WORK [0:46]
Hi Emily, thanks for joining us today. I'm really excited to speak with you about your role at Uber, diversity and inclusion and also a little bit about Uber's COVID recovery strategy which would be really interesting to hear about. So, to start us off, can you give us a little bit of background about yourself and your journey into data science?
Emily [1:09]
Sure, and I'm really happy to be joining you. Thanks for having me. So I got into data science about five years ago now, technically, but I would say, I have sort of been building along that path unknowingly since college. So in undergrad, I studied environmental science and economics, which is not the most traditional way to get into the field. But I ended up writing a senior thesis about energy efficiency that leveraged a lot of different econometrics and statistical techniques that then helped me find a job after graduation, that was working on forecasting and experimental design and measurements for a small tech start-up. And so that led me into learning a lot more about programming and other modelling techniques.
Emily [2:01]
I found it so interesting. I really love making decisions with data and being able to discover insights. After working at that company for several years, I thought it would be areally great to work somewhere with, really quite a big name in data science so that I could grow my technical skills and explore more of the field. And so Uber has a pretty great reputation when it comes to being a data driven company.
Emily [2:27]
I was fortunate enough to end up here and so I started on a very small team with a very small number of people in data roles, I got to do a little bit of everything for my first year at Uber and then migrated into an even more technical role after about a year and since then I have been working on a bunch of really interesting problems and moved into management in the last couple of years . I am enjoying the organisational problems almost as much as the technical ones these days.
Charlotte RE•WORK [2:59]
Fantastic. What are you currently working on and what excites you about the role at the minute?
Emily [3:06]
Well, I actually spent the last three and a half years working with our finance data science team, and then just six weeks ago I moved to work on our customer engagement products, which are the subscription passes. So being able to buy a monthly pass that gives you specific benefits when using any of our products and also our loyalty programme, so the programme that will reward you for taking trips and ordering with each app. That's been really exciting for me, because I'm at the same company, and I have a lot of the support that comes with, you know, being in the same job, but I'm actually doing a completely different job. So that's been really fun learning a new part of the business. And then as you've alluded to earlier, this is very relevant to our COVID retail recovery strategies.
Emily [3:56]
So that's been very exciting and thinking about how we can re-engage customers when they have not had the habit of necessarily taking Ubers anywhere when we've all been staying home, rightfully so. But we will start going places again, hopefully soon. And we want to do so safely. But its figuring out how to do that.
Charlotte RE•WORK [4:16]
Yeah, that's really interesting work. And you've previously done some work on large scale business forecasting at Uber, and the success and failures in adapting to a changing business. So can you tell us more about this and what the successes and failures are?
Emily [4:35]
Sure. So this was in my time on the finance data science team, where we worked really closely with financial analysts around the globe, and Uber grew very quickly over the last, well actually since it started, really, it hasn't stopped growing fast. And so forecasting how quickly our footprint in every individual city will grow is really critical to ensure that we can create scale effects and that we have enough supply to meet demand and that demand to meet supply enough customer support agents, enough HQ staff to keep it all running smoothly.
Emily [5:11]
And so we are forecasting how many trips will occur as the number one metric for us, but a lot of other metrics as well to help us keep things, keep things running. And so that we did at a city level, which you can imagine you've probably taken Ubers in many different cities, we operate in hundreds. This was a really fun, challenging problem because no two cities really look alike. So we could share a lot of insights across geographies, but we couldn't necessarily use exactly the same model everywhere. And then we couldn't even use the same input features really, you know, certain cities have different products launched or they have different regulatory conditions.
Emily [5:55]
It was really interesting working closely with our local teams to understand what we needed to take into account and understand what their tolerance for error is, and whether we should be maybe calibrating toward over forecasting or under forecasting when we know that there's going to be bias in one direction. So it was very challenging, and then when you ask about what are the successes and failures. I think I'm speaking now from a place of having worked on those problems for almost four years, but in the early days, we thought that maybe things would look a lot more similar to one another.
Emily [6:31]
So we were trying to use one model to fit the whole world and that left us with big problems in places. For instance, when we launch in a brand new city, it's really hard to forecast a new city and you might think you know what it's going to look like based on similar cities near it. But if you're not thinking closely about the specific factors that separate one city from another, you can do a really bad job.
Emily [7:00]
We had some cities where our forecasts were so spot on, and others where we were wildly off and causing huge problems for local teams. So I definitely learnt a lot over the years.
Charlotte RE•WORK [7:12]
Great. What do you need to think about when getting internal buy in on data science projects? And what does this involve?
Emily [7:21]
It really takes a lot. So, and this is coming from Uber, where data science is really, really integral to so many things about the business. I mean, the core thing that we do is match riders who need to go somewhere or people who are looking for food to a courier driver, who will make the trip, which in itself is a data science problem, it’s a matching problem. And so we're very much a business that's comfortable with data science having a leading role, but it's still because the business is so interconnected, and it's a big marketplace. So any one thing you do has ripple effects, on the whole company wide.
Emily [8:02]
There's a lot of communication and coordination that goes into making any decisions, about especially automated decision making that we might do. For instance, on the forecasting example, and those forecasts are also used in determining how many servers should be online at a given time, they're used in like I mentioned, customer support staffing, and so when we would go about making modifications to those models, we would work with business partners from all over the organisation to ask, how should we optimise this will? Will this outcome fit your needs?
Emily [8:36]
So it does take a lot of communication here and there, maybe more familiar to the industry at large. I'd say it really comes down to identifying what the overall business objective is that you're solving for and not getting lost in the technical details. There are a lot of times when there's a really lightweight solution that gets you most of the way there, and maybe speed is more important at that moment than perfection. Those are challenging decisions for data scientists to make. We really love building fun, complex things, so that might be the hardest part.
Charlotte RE•WORK [9:18]
Great, and thanks so much. That's really interesting to hear about. We're going to move a little bit more on to diversity and inclusion, so as a female data science manager, from your own experience, what would you say were the steps you took to get to this point?
Emily [9:35]
I've been extremely fortunate to work with people who are willing to answer my questions all the time. I think that is something that is valuable for anybody. But I think as a female in the field, it can be hard to ask those questions, and so just getting comfortable with asking questions that you might worry that other people know it all already. And you worry that you're the only one who doesn't know it.
Emily [10:06]
I just had to get really comfortable asking those questions, and even now, as a data science manager for several years, I just started leading this new team. There's so much I still don't know, I'm asking my team questions all the time, and making sure that I really deeply understand the problems and the solutions that we're working on. It's still uncomfortable today, but pushing through that is something that I've been able to do. Then I think something else that has been important for me, or I perceive it as important, is I think there's a glass ceiling that we talk about across all of the working world for women.
Emily [10:43]
In technical roles, I think we all have seen it's as if there are even fewer women in leadership roles. And so I had, like I mentioned, an undergraduate degree that wasn't extremely relevant. I started taking classes on the side just to make sure that I actually had the fundamental side needed. So I started taking classes at Berkeley and classes at Stanford and other schools.
Emily [11:09]
I was racking up so many credits, I figured I may as well turn it into a master's degree because those qualifications really do end up being important as you're looking at higher and higher level roles. So even while working full time at Uber, I was doing a part time Master's in Computer Science. I definitely know men who are doing the same, but I don't know if their reason for doing so is identical. I think as a woman, it feels even more critical for me to have have those letters after my name at some point.
Charlotte RE•WORK [11:40]
Great, that's really cool. Thank you. Do you have any role models in the field and what did they teach you?
Emily [11:47]
I definitely do. I'm, as I mentioned, super, super fortunate to have worked with people who are willing to invest in me, and my previous director was a woman named Fran Bell. She was at Uber for a number of years and just left earlier this year to go work in an exciting division for Toyota.
Emily [12:11]
She taught me so much, especially about leading data science teams, and being very rigorous, being very organised, and being very inclusive. We were talking about getting buy in earlier, getting your buy in stepwise. So starting with who's closest, and who needs to be 100% bought in and then expanding sort of in concentric circles. She's had some amazing advice for me about leadership and keeping your team motivated and engaged. Actually, even as I was considering taking this last role, she's somebody who I called for advice and just watching her career is very exciting and I'm really grateful that she's willing to take the time to talk about mine.
Emily [12:59]
So when we talk about increasing the number of women in the field, what's interesting I've noticed is, though the number of women in tech is much smaller proportionately than the number of men, data science, I've observed more equality, which is really neat. So what's interesting to me about that is data science as a field is newer. I think there simply wasn't as much of an entrenched masculine dominance as there is, for instance, in engineering.
Emily [13:32]
I think that's given an opportunity for equality to just exist or at least exist more from the get-go. And I think a big part of that is there weren’t established data science leaders who all looked like one certain blueprint. There is still in balance, but I think, so what that makes me think is the leadership roles matter a lot.
Emily [14:04]
For recruiting teams to focus on getting women into data science leadership roles sets the tone, it shows people that they should stay in this career and they can continue growing and get there. Like I mentioned, I worked for a female director and that was extremely motivating for me. I've actually had the privilege of working for two extremely high-level women at Uber, and that's extremely motivating to have them on our data science team.
Charlotte RE•WORK [14:30]
Fantastic. Can you tell me a little bit more about your Women in Data group at Uber?
Emily [14:35]
Sure, so a few years ago, the number of women who worked in Data Science at Uber realised that though we had a really fantastic lady data group, among other groups for minority individuals and another social and employee resource group at Uber, we didn't have one specifically for Women in Data Science, and so they founded that.
Emily [15:01]
I joined the leadership team for that group a couple years ago, and have really enjoyed this, not only as a way to make a change and increase the number of women at the company, but especially in the data science field. Working on various recruitment initiatives and partnering with organisations. Honestly, around the world to do events and help women at Uber in the technical field, or especially in data science, share their work and have a platform to speak.
Emily [15:36]
It's also been really wonderful just to build a community. Like I mentioned earlier, you can feel a little out of place and having questions, or just feeling like you're one of few in in your demographic and a job. We have a really active Slack channel, we have community events frequently and just building that group has been such a wonderful part of the last couple of years for me.
Charlotte RE•WORK [16:02]
Great, that's a really nice project. Then I guess where can our listeners keep up with you? And do you use social media?
Emily [16:11]
I do. I'm pretty active on Twitter, and you can find me there @EmilyWBailey. And that's about it really, um, my dog has an Instagram.
Charlotte RE•WORK [16:27]
We can put the link to that in the description box.
Emily [16:31]
And he doesn't talk about data science.
Charlotte RE•WORK [16:34]
Great. It's been so lovely to speak with you today, Emily. I think that was a really good conversation. Thank you so much for joining us.
Emily [16:43]
Thank you so much for having me.
Charlotte RE•WORK [16:51]
As Emily said, you can follow her on Twitter @EmilyWBailey and keep up with her work. We hope that you enjoyed the episode and if you have any questions, know someone who would be great to get involved, or have some things that you'd like to ask our upcoming guests get in touch at [email protected]
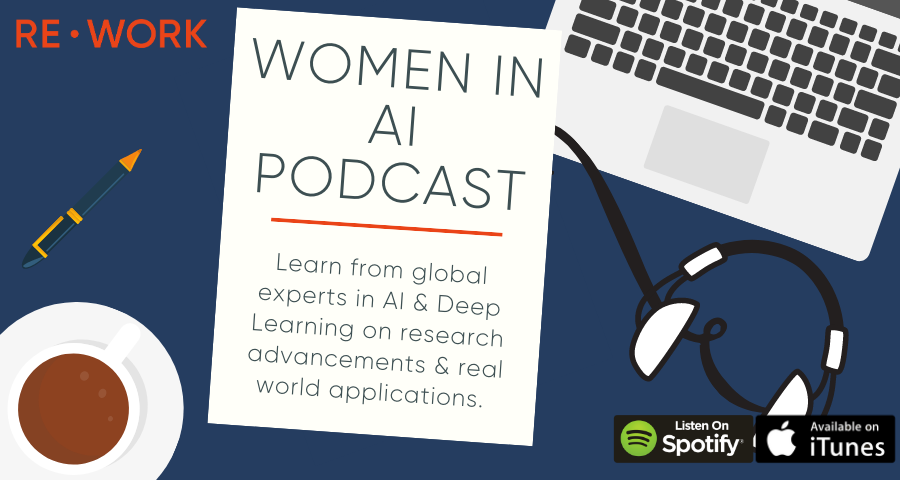