Artificial intelligence (AI) is a major driver of value for the enterprise. According to a recent AI study from IBM, 82 percent of organizations are now at least considering AI adoption, and the number of companies that are beyond the AI implementation stage is 33 percent higher than it was in 2016.
What's more, by pairing AI with other exponential technologies such as automation, blockchain and the Internet of Things (IoT), companies are redefining their business architectures. The IBM “Cognitive Enterprise” report highlights how these technologies represent the next inflection point for the enterprise comparable in scale and scope to the introduction of the Internet and mobile technology. The cognitive enterprise is a framework for companies to define and pursue a bold vision to realize new sources of value and restructure their industries, missions, and business models.
A pioneer in using multiple emerging technologies to reinvent industries — in this case, the global trade market — is Maersk, the world's largest container shipping company. Through a combination of digital, cognitive and blockchain technologies, Maersk is developing a trade digitization solution to help participants across the ecosystem of shippers, ocean carriers, ports and customs save billions when adopted at scale. The network will help Maersk optimize and increase speed, fight shipping fraud and completely disrupt traditional trade. This is just the beginning of what AI can help companies achieve.
AI is the next step change in enterprise productivity across industries and domains. For instance, a prominent mining company that compressed 3D data via deep learning and used Watson AI to transform geological targeting, generating dramatically higher yields with less environmental impact.
How to get there
Enterprise leaders understand the importance of integrating AI into their business models. However, there's a big difference between experimenting with AI and true enterprise-grade integration of AI.
To reimagine customer experience, redesign core processes and revolutionize business architecture, AI integration requires a deliberate and practical phased approach. Consider existing investments and constraints and implement smart compromises to balance value and risk. Other important considerations include impact on the talent, culture and stakeholder ecosystem. At the core of deploying AI at scale is its data foundation.
Every day there are 2.5 quintillion bytes of digital data produced. Yet companies make use of barely a quarter of their own enterprise data. AI includes a non-programmatic set of algorithms that thrive on diversity and quantity of data.
A methodical approach begins with understanding value drivers, business imperatives and target business architecture. Building on that, an organization should identify select data entities as corporate assets for a distinct competitive differentiator, constituting the baseline of an enterprise data strategy, or its “data fabric”. Implementation requires a broad view of data ingested, as well as smart choices on data integration, governance, privacy and security.
Design your business architecture to ensure four outcomes:
- A resilient data architecture
- High-quality data management
- Strong data stewardship
- Change management practices with executive leadership support
Build an AI-ready data architecture
An enterprise data architecture is “a set of rules, policies, standards and models that govern and define the type of data collected and how it is used, stored, managed and integrated within an organization and its database systems. It provides a formal approach to creating and managing the flow of data and how it is processed across an organization’s IT systems and applications.” Essentially, it is the data DNA of an organization.
A well-designed data architecture can help enterprises become AI ready via simplification, standardization and cost control. It replaces the redundancy of multiple tools and processes with a designated catalog of services. It also allows "one fact in one place" storage, ensuring data integrity and creating an authoritative record of truth.
With the right framework in place, each data element created is automatically cleansed, rationalized and consolidated into the foundation. This approach makes the data immediately available for real-time analytics rather than waiting for it to be prepared in a batch fashion. This is the approved provisioning point for consolidating point-to-point interfaces while reducing complexity and enterprise risk.
As an organization identifies the right combination of repetitive decision points for AI, it's critical to determine whether the data that's needed to define those business rules is available in an AI-useful format. If so, measure the confidence in the data quality to determine whether automation is viable. If there are unwritten rules or poor-quality data, reinforce the data foundation to improve results from AI.
By evolving data architecture through ties to your business process model, you can create points of integration across all enterprise applications. That framework becomes the bridge that facilitates effective interchange of information, simplifying how the organization uses data and making it easier to integrate AI.
It sounds daunting, yet a multi-brand retailer made it happen for its customers. By integrating online and offline events streams, it took a mere six months to open the door to over $200 million in cross selling annually.
Start at the foundation
Every competitive enterprise should make mapping out the data architecture step one in its foray into AI integration. AI thrives on data. By deploying a pragmatic approach, organizations can realize the value of AI today and maximize returns on existing investments.
To learn more about our IBM Services capabilities, visit our Big Data Services and AI Services web pages.
Join IBM Services at the Applied AI Summit next month to discuss how to reinvent your company with AI and become a Cognitive Enterprise. Get your tickets here now as they are running out.
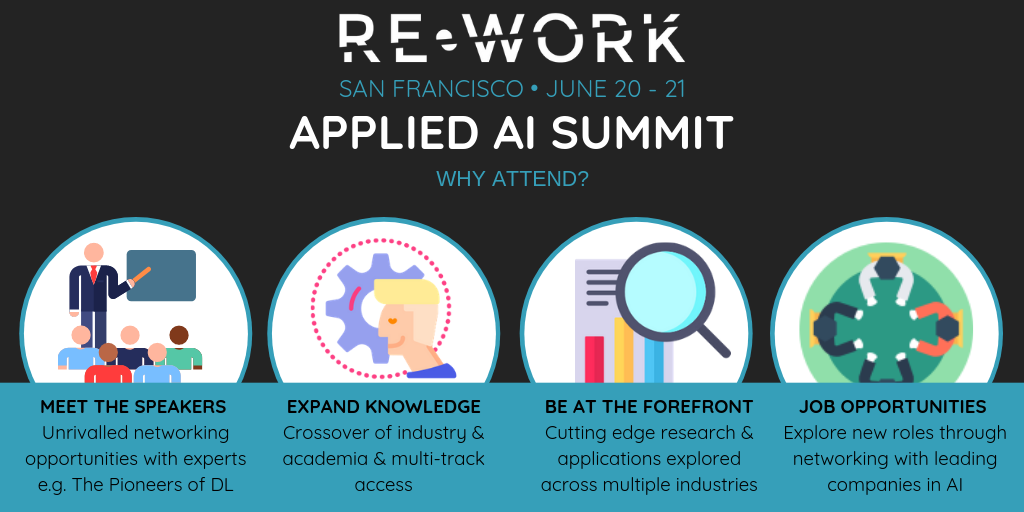