At the 6th annual Deep Learning Summit in London, attendees congregated to hear from industry leaders, academics, researchers and innovative startups, presenting both the latest cross-industry technological advancements and industry application methods. Running in parallel was the AI Assistant Summit and AI in Retail and Advertising Summit, resulting in some of the world's leading experts from Universities, brands and emerging startups coming together.
We began the day with Huma Lodhi, Data Scientist at BP, discussing some of the tricks and tips she has picked up during her work in Deep Learning, with intelligent methodologies using structured and unstructured data as the focal point. Huma stated that these methodologies whilst beneficial from the standpoint of customer service improvement, increased revenue and improved loss prevention, have been found to hit initial stumbling blocks with industry application:
“We need to find better methods to use this data for our real world applications. Examples of this can be Noisy Data, Missing data, or unstructured data. This gives us the principle problem for data, Quantity vs Quality.”
Huma then went on to suggest, however, that there is no straightforward or quick fix for these issues due to this variation of industry requirements
“It is important for us not to focus on specific algorithms for specific problems. These kinds of algorithms usually fail in real world applications. We should try to take parts of different algorithms and combine them into a real world-applicable solution. This can be in the form of Deep Learning Neural networks.”
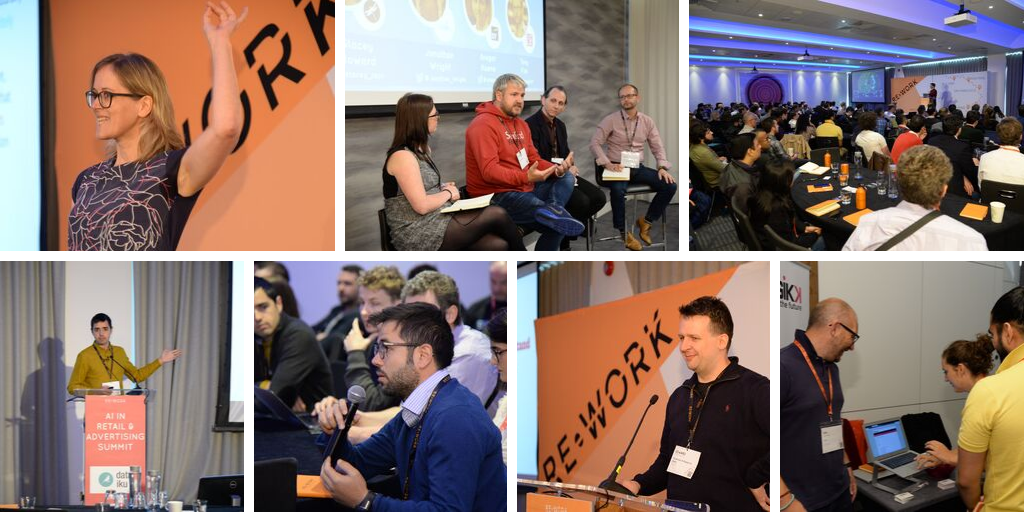
Experimentation with data was also a key topic covered in the first Deep Dive of the day, granting the opportunity for attendees to join a more intimate style of session with regular question intervals. Hado Van Hasselt, Senior Staff Research Scientist at DeepMind opened his discussion with the statement that we are currently at an intersection of two fields, citing that the combination of both DL and Reinforcement Learning has brought an air of excitement to DeepMind. Hado commented that the current standing of processes at DeepMind are rapidly developing. He explained that DeepMind first automated certain physical solutions using GO!, once they had learnt to do this, the efficiency and accuracy of problem solving increased.
Once automation became possible, DeepMind took another step forward with focus on agents learning solutions from experience. That said, the initial data used needed to be of the highest quality due to the sequential nature of learning. Hado stressed that when models are built on data with mistakes, the intended outcome is not always achieved. Goal-optimised reward systems were given as an example of the need for correctly formulated algorithms from the outset. Hado suggested that DeepMind had to find a goal reward which was easy enough to specify and deliver, but which also gave us good results. One test completed saw a car driving around a track with the goal incentive increased through quicker lap times. The agent soon found that this was not through distance travelled but simply speed - this found the car on its roof with wheels spinning and earning high rewards without supplying any usable data for DeepMind.
Single reliance on either human behaviour or model data was also quashed by Hado, who deems a mixture of both to be a determining factor in the quality of results. AlphaGo trained its policy network by first training on human behaviour, which eventually saw it become far more skilful than your average player, however, DeepMind also found that reliance on this model can also see potentially problematic results as the optimal solution for gameplay is rarely that of human decision making.
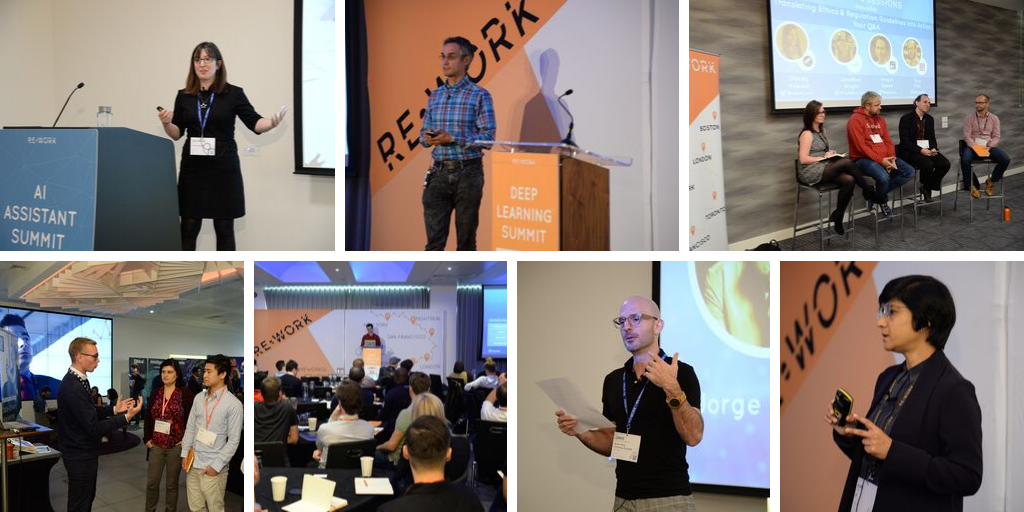
One of the final presentation of the morning saw Richard Turner, University of Cambridge’s Reader in ML, discuss Data-efficiency and continual learning in relation to Deep Learning algorithms. Richard began by explaining both the benefits and restrictions of Deep Learning in his work
“Deep learning has revolutionised many facets of machine learning, however it suffers from a number of crucial limitations that severely limit its applicability to real world problems. For example, deep learning is data hungry requiring large numbers of labelled data points. Deep learning also fails catastrophically in continual learning scenarios, where data and tasks arrive continuously and must be learned from in an incremental way.”
The solution for this? Multi-task Learning! Richard further explained the need for manipulation of model behaviour on an incremental level, involving tonnes of hand labelled data points which is not only time consuming but too restrictive to be scalable:
“An example I can show you of the Deep Learning approaches we are using for our Clarifai database includes this image of the river in Cambridge with people punting. If we run clarifai it comes up with good labels like canal, water, river, watercraft, but not punt. What would we have to do to include missing labels that aren’t present in the training data? We’ll have to do 2 things - take pictures of punts in different environments and add them to the database and retrain from scratch. This process will take weeks. It’s not scalable.”
From unscalable and somewhat painstaking manual algorithm adaptations, we moved to something often associated with a younger generation, building on Minecraft. Just before breaking for lunch, Katja Hofmann, Principal Research Manager at Microsoft, presented both the pros and cons of Minecraft not as a pastime, but as an AI experimentation platform, on which Microsoft developed ‘Project Malmo’.
“Minecraft is an open-ended game, creating biomes through what seems to be an infinite variety of tasks for AI agents. This is seen to be beneficial as most AI models are extremely customised and specifically trained for certain real-world solutions”.
Katja suggested that the open ended nature of the platform allowed for the agent to visualise the digital world of Minecraft, not as we do, but rather as a table of data from which it can develop understanding. That said, this must be carefully scrutinised as Katija explained that the balance between exploration and exploitation is very sensitive, AI agents are often greedy with data which causes a slow learning response through the sheer amount of data processed, of which sixty million frames of recorded human data are available. When used correctly, Minecraft is touted as a platform on which AI research can work toward faster learning, complex decision making and, ultimately, collaboration with its human players!
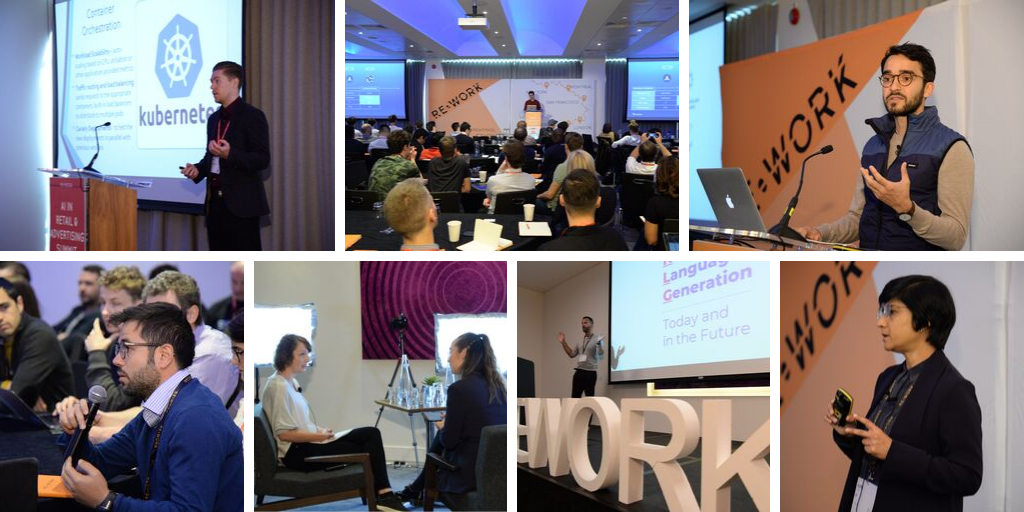
Post lunch saw one of the most rewarding sessions of our summit, with the up and coming ‘Rising Stars’ in the AI world coming together to present their latest work. Speakers from the London College of Fashion and the ‘Teens in AI’ group presented on subjects ranging from technologies being used as fashion communication tools to cancerous cell detection through AI and everything in-between. Opening the session were Hydroponics.AI, a startup focussed on optimising conditions in a hydroponic system so that plants can grow more efficiently using less space by using data from sensors to detect and change the conditions under which the plants are growing. The message? That we are sleepwalking into a global crisis and they are working diligently to find the solution! Other presentations included GreenFeast and EarlyCatch, focussing on carbon efficient food recognition and cancer cell detection respectively.
One of the final talks of the day saw Kallirroi Dogani, Machine Learning Engineer at ASOS discuss the Neural Collaborative Filtering model for product size recommendations currently being tested. Kallirroi began her talk with reference to the current problems faced in retail, providing customers with accurate size guidance. Recent research at ASOS has been focussed on size predictions for consumers, which in turn has aided in the availability of products on a wider scale, credited to the training of neural networks on previous purchasing history.
Kallirroi further suggested that this model needed to initially be trained on a brand level, later transferring to products, slightly customising each model for each gender and product category. It sounds like it won’t be long before we always find that bargain in our size when we head to the shops!
As the day drew to a close, attendees gathered to share their experiences and learnings from the day in the networking drink period. Here’s what our attendees has to say about the first day of the summit:
“Really nice spread of talks, great mix of theoretical and applicable across the different tracks.” - Tom Bowling, MMR Research Worldwide
"I'm just getting into the field of AI whilst studying for my PhD in Biology - the event has been really eye opening and is helping me consider working in data science." - Gabriel Harrington, Keele University
“Great audience interaction, really tackling the issues which people tend to dance around” - Ansgar Koene, EY
"I work at a hedge fund and was looking for something in my field for relatable talks and it's been great, there have been a few clashes but I want to see everything so that will always happen! Great talk from DeepMind too!" Chris Nicholls, Quadrature Capital
Start a free membership of our extensive Video Library here.
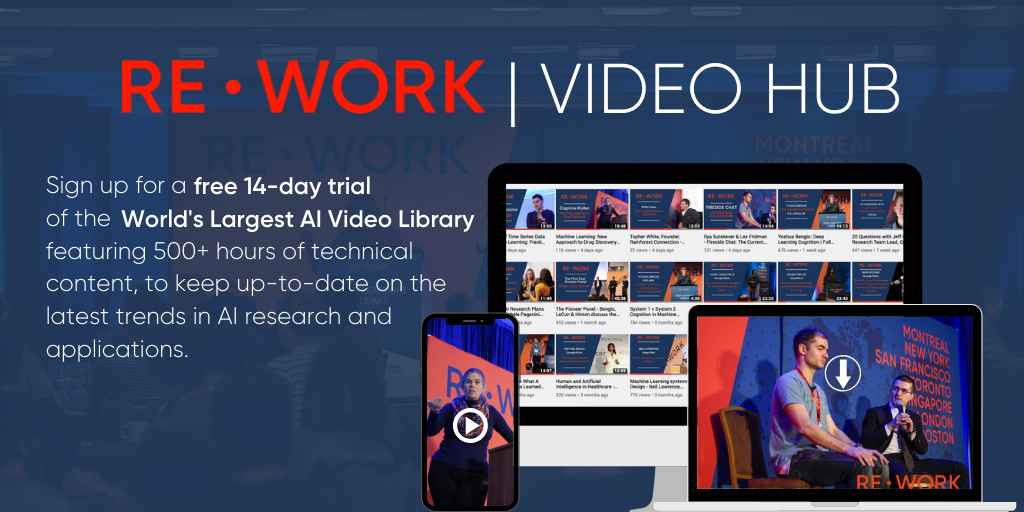