At the Deep Learning Summit in Montreal, RE•WORK brought together the ‘Godfathers of AI’ to appear not only at the same event, but also for their first-ever joint panel discussion. We saw Yoshua Bengio, Yann LeCun and Geoffrey Hinton come together to share their cutting edge research progressions, first memories of each other and AI as well as discussing their papers and predictions for the future of AI. This panel discussion is moderated by Joelle Pineau, who at the time was Associate Professor of Computer Science at McGill University and has since also become the lead of Facebook's Artificial Intelligence Research lab in Montreal, Canada.
The candid-style 45-minute panel opened with Joelle asking each to introduce the other, explaining how they first met & their first memories of each other, which turned out to not only be quite humorous, but incredibly detailed given the length of their friendship and working relationships. Post-introduction, we were interested to hear the challenges faced by the three panelists in their work, which many would believe to be limited due to their presence in the field. LeCun emphasised that, when compared to a decade or so prior, the speed at which AI is developing has caused roadblocks, with the the previous trial and error method no longer widely accepted.
Following this, Joelle asked each member to highlight a paper of theirs which they believed didn't get enough credit, either causing issues with their H number as Geoff joked, or due to a lower number of citations regardless of content. The must-read papers, considered seminal contributions from each, are highlighted below:
Geoffrey Hinton & Ilya Sutskever, (2009) - Using matrices to model symbolic relationship
Yoshua Bengio, (2014) - Deep learning and cultural evolution
Yann LeCun & Tom Schaul, (2013) - Adaptive learning rates and parallelization for stochastic, sparse, non-smooth gradients
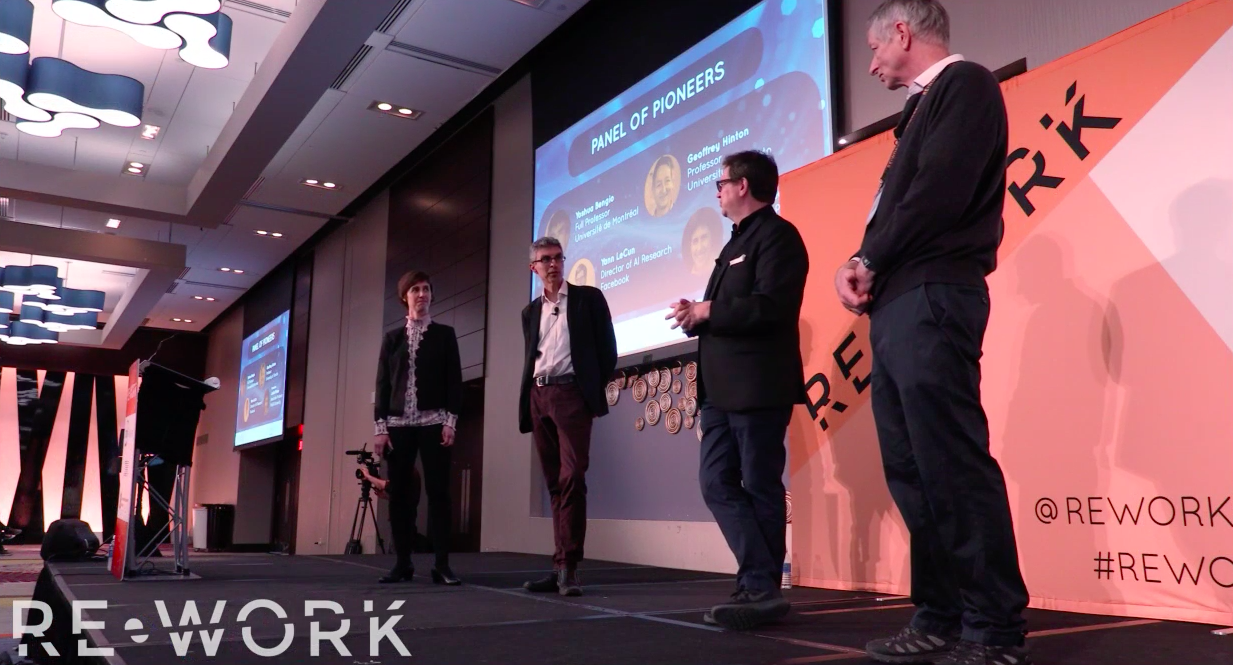
Joelle later quipped that they could now be considered the three amigos, having previously disagreed on different subject matters in their early careers, that said, we wanted to know what they still don't agree on! Yann broke the silence by suggesting that the disagreements are more so becoming different approaches to the same subject matter, something which can only be beneficial to the field!
"At a certain time Yann wanted nothing to do with probability, he called us the probability police, he would calculate probabilities any way without calling them partition functions" - Geoff Hinton & Yann LeCun
Are we in a phase where Deep Learning will always be equated with progress in AI?
"I think we need new ideas to build on top of those which have been successful in AI, maybe some of those ideas will be inspired by those done before, but we have to be inventive" - Yoshua Bengio
"Deep Learning is not going away, we will still be using some of the practices used now in 20 years, however, it is not completely sufficient for progress so we need to think about new architectures. A lot of people are excited about dynamic architectures and that is very interesting from an NLP standpoint. Perhaps going back to the mid-2000's with sparsity may be beneficial" - Yann LeCun
"One of the things I think we all believe is that the biggest obstacle is not having an objective function for unsupervised learning that doesn't require us to re-construct pixels. But the question is what is the objective functions" - Geoffrey Hinton
The Chinese game of GO was then referenced by Joelle, which we know has since seen players of the highest-level retire due to being outsmarted by AI. Joelle asked the next grand challenge which the pioneers thought would be next after GO. Geoff joked that getting below 1.5% error on smallnorb should be next on the hit-list. Starcraft was also mentioned from Yann, something referenced to be considerably more difficult than GO, on which we have seen some great work from DeepMind since this panel.
Yann went on to suggest that we have seen very little use of ML in Starcraft which of course we would see develop over the coming years, once again proving that the pioneers foresight of industry trends and challenges shows why they are so very coveted in the field. Yoshua then referenced an initiative from his lab called the 'Baby-AI' game, the preface being that the a human has to teach a (virtual) baby AI using natural language and pointing, similar to a parent. Happening in a virtual environment, the game was to be used to examine the rate at which learning can be affected using AI.
Anyway, enough from me, enjoy the full forty-five minute panel video here.