A summary of Day 2 of the hugely successful Deep Learning Summit and Responsible AI Summit in Montreal.
Sportlogiq were up first on the Deep Learning stage with Bahar Pourbabaee, Machine Learning Team Lead, discussing some of the main challenges in developing and deploying deep learning algorithms at scale. The sheer size of this scale was reiterated with Bahar suggesting that they are processing more than 60,000 sports videos from different sources, all of which include many thousands of frames. Bahar's first example of Sportlogiq's latest work depicted that of a fast moving Premier League Soccer game, with examples showing the sheer depth of analysis suggesting that both decisions and non-decisions alongside their consequences can be looked at and scrutinised, whilst individual joints of players and their lateral movement was observed under the microscope.
"Our engine can be applied on any input data. Whilst we mainly focus on visual data, you can also use it for numerical data, be it heart rate monitors, motion trackers or other across a wide variety of different sports and sporting events."
Bahar then continued to detail some of the problems with the visual perception of their learning representation model which included player/object detection, player/team identification, state estimation and data association. Whilst the model is extremely developed, Bahar suggested that the chosen image quality and the level at which a team may invest, will have a direct impact on the quality of data a team receives. If a team wants high-level data, they can invest to a level which would supply them with data far removed from that which it would be possible to collect otherwise.
"Cameras have a big bearing on the quality of our insights. It is difficult to ascertain which players are which at times, and also which teams they are playing for without manual input."
It was then the turn of Jeff Lui, Director of AI at Deloitte to take to the Responsible AI stage to discuss not only the applications of AI at Deloitte but also the ethical considerations needed within business applications in industry.
.@jefflui from @Deloitte talking about ethical deployment of artificial intelligence through an “AI Ethics Funnel”. #reworkai pic.twitter.com/nTIFCiklrE
— Josh Brandoff (@jbrandoff) October 25, 2019
How is it possible that we can use AI to further understand people? Jeff suggested that people are the most under researched asset at the moment. Even listening to Yoshua yesterday, it is clear we are looking more into robotic emotion and ignoring how we can enhance human development with data science. The process at Deloitte for algorithm development follows the thinking pattern of:
"What do we build, should we build it how can we build it and is it safe when creating it using AI. Some things don't need AI, you can use an excel macro and get it done in a few days."
Every decision we make as individuals relies on a flow map of input, prediction, judgement (do I need training), from this there is an action which creates an outcome with a feedback loop then initiated for further training. This thought process is critical to how we deploy AI, with judgement being the key as we are entering a world in which we are moral agents needing to make decisions on a futuristic world which includes self-driving cars and alike.
Following a great talk covering industry wide ethics, the first fireside chat of the day got underway with Frankie Cancico, Senior Engineer and Data Scientist at Target and Valentine Goddard, Founder of the AI Impact Alliance. Frankie went into great detail on Target's ethical understanding and intentions when creating and designing new models, suggesting that it cannot simply be a PR stunt, but that it has had significant backing and an increase in recruitment for this area:
"Responsible AI is about thinking about the end user, but not necessarily in the traditional way. Target has an internal entity which looks at ethics & responsible uses of AI to ensure that we have minimal bias in our models which can happen if a procedure is not developed to work on a wider dat set. Without us having something like this in place, we can't claim to have the accountability we do."
Frankie continued to suggest that companies need to look further outside of their own ethical compass and aim to develop tools which are available and useful to wider society with the possibility of implementing them across different organisations:
"One thing I'd like to see in the future, is companies looking at what is going on outside their organization. The mass are asking for regulation, so organizations need to ask why? You don't have to be a data scientist to start a discussion on how AI & data can be used responsibly. We need different perspectives on this issue to be able to really address the question."
Throughout both days of the summit we have also been undertaking in-depth interviews with some of our speakers, delving a little deeper into not only their current role but also how they found their love for AI and what advice they would give to someone starting out their career in Data Science. Across the two days, representatives from Facebook AI, UBER, Google, MILA and more were put in front of the spotlight. Keep an eye out for these over the coming weeks!
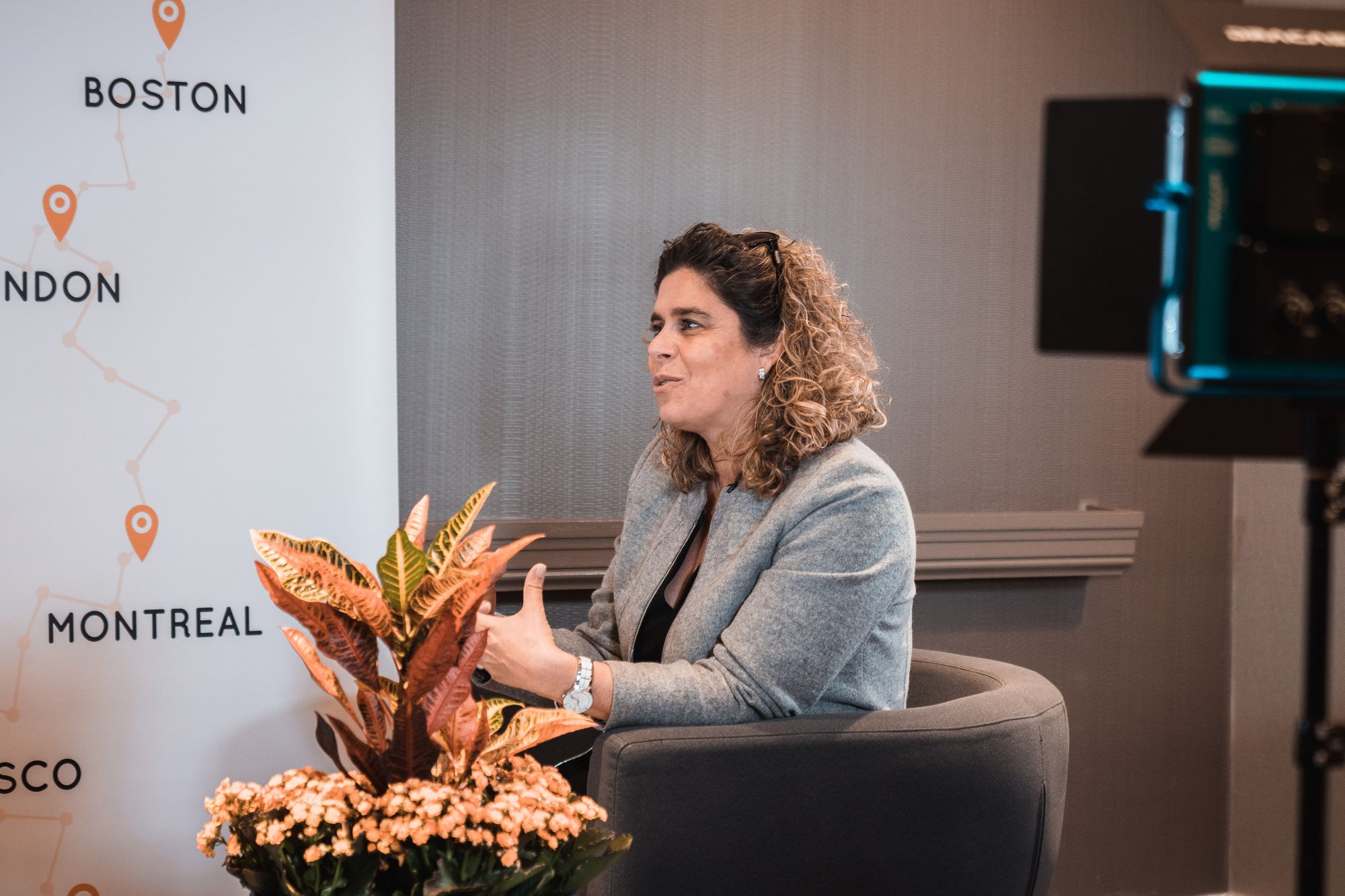
The Deep Dive sessions then started, allowing for a more intimate setting of discussion and collaboration between attendees. Topics ranged from Deep Learning in the 3D world to Deep Learning in Transport & Cities: The Human Urban Mobility, whilst also covering trends on investment and AI startups. Here are some of our favourite quotes from these sessions:
"As we use DeepWait for wait time analysis, we replace the linear log risk function with a neural network and use feature selection to enhance performance.” Arash Kalatian, Ryerson University
“Lots of companies find a problem they want to solve, but don’t have the solution down yet. Often they want investment to hire someone who has a phd etc to solve the problem and build the AI, but that is a big gamble and is a second step, not a first step.” Sergio Escobar, BCF Ventures
Governance in banking was then the subject of focus in the Responsible AI summit, with the opening remarks suggesting that, historically, the banking sector has always been a large ship that is hard to steer into new directions. In terms of innovation and technology, large banks have been less agile than Fintech startups. Now that banks are beginning to catch up, alongside technological challenges, there come structural and cultural shifts that bring about a different type of challenges. Manuel Morales, Chief AI Scientist; and Dominique Payette, Legal Affairs Lawyer both from the National Bank of Canada scratched beneath the surface of the challenges currently faced in the financial sector during their talk which mapped out their current breakdown of data use at NBC. The cross collaboration between data science and the legal department was unique and gave a different insight into the work happening behind the scenes in NBC:
"We have all heard about predicting financial habits, here we face the 'cool or creepy' dilemma. If we knew you were at the airport, we could send you an advert for travel insurance, would this be cool or creepy? (Majority of audience raises their hands for creepy). This is good to know from a legal standpoint, we can see that whilst this could work from a data science viewpoint, we can look at the ethical and potential legal viewpoints of this".
“It’s not every day that lawyers get to work along scientists!” - the #ReworkAI summit is really championing cross-collaboration between STEM & social sciences / humanities 🙏 #kids2030 pic.twitter.com/LMnWJUJMI6
— Kids Code Jeunesse (@KidsCoding) October 25, 2019
In one of the more technical talks of the afternoon, Subhodeep Moitra, Research Software Engineer at Google, discussed Deep Learning for programme repair. To begin his talk, Subhodeep discussed the need for both Machine Learning and ML for Software Engineering, suggesting that through measuring against an X and Y axis of Data Quality v Amount of Data, it is possible to create the opportunity for advancing ML algorithms and data harvesting processes. That said, Subhodeep was quick to suggest that to advance even slightly in the ability to repair programme faults, we must understand the developer process, how they work, their skill level and how they look for bugs. Through this, we can forecast potential problems which may arise. Without this, the mass amounts of data are, well, just data.
"The software development process can be frustrating, painful and costly; rife with bugs, project delays and unexpected outages. If machine learning were to help with software engineering it would make for the stuff of dreams." - Subhodeep Moitra, Research Software Engineer at Google
As the talks came to an end, attendees once again gathered for networking drinks to close the summit discussing some of the subject matters covered over both days, whilst also voicing their thoughts on the summit as a whole:
"I truly enjoyed every minute of day 1 yesterday. I'm on my way to day 2 now, with a great schedule. Thank you RE•WORK for making it a day to remember!" - Vincent Boucher, MontrealAI
"It's been very informative and worthwhile. I've learned a lot to take back to my colleagues" - Gary Choy, Research Engineer, Communications Research Centre Canada (CRC)
“Hearing talks from visionaries like Yoshua Bengio really broadened my horizons and makes this summit very valuable. I can learn not only about the technical uses of AI, but also broader questions around AGI.”- Alaa Khamis, General Motors of Canada
“Top notch speakers, especially Hugo Larochelle, as his talk has really given me some strong takeaways that I can use in my startup which has limited data” - Filippos Konidaris, C4P
If you haven't yet, make sure you catch up on yesterday's content with blogs from Hugo Larochelle, Doina Precup and Yoshua Bengio. Sad that it's over? Fear not, we're back in the US in May next year, bringing the Applied AI Summit and AI for CPG to Austin! Register your pass before the turn of the year to save over $600!