Trying to predict what people are going to be buying is a tricky thing for retailers.
With there being numerous variables to take into account, understanding how customer spending differs across factors such as price, location, seasonality, and product type can be incredibly difficult. This is an area in which the capabilities of retailers can be greatly enhanced by the assistance of machine learning.
Two of our incredible speakers at the recent RE•WORK AI in Retail Summit, Sharon Gieske (Data Science Tech Lead – Picnic Technologies) & Rick Rijkse (Principal Data Scientist - Ahold Delhaize), delivered talks on their experience using this application of ML to the shared use-case of groceries. They both spoke on the individual solutions their businesses used for improving demand forecasting.
As Sharon succinctly put it, “demand forecasts are critical for retail”, but this is even more imperative when considering the unique challenges of grocery shopping, where Rick identified issues such as the fact that products are perishable, huge outliers like the Covid pandemic exist, and demand does not necessarily equate to sales due to the presence of out-of-stock items.
Sharon, being the Data Science Tech Lead at Picnic Technologies, an online-only grocery business, spoke about their employment of Temporal Fusion Transformers (TFTs) as a solution to predicting demand. Not only are these adaptable to different feature types, but they’re also capable of multi-horizon forecasts. They also have explainability ‘out of the box’ which solves the common issue of ML algorithms being black boxes that lack in explanation for their outcome. The image attached below shows what these models look like on paper.
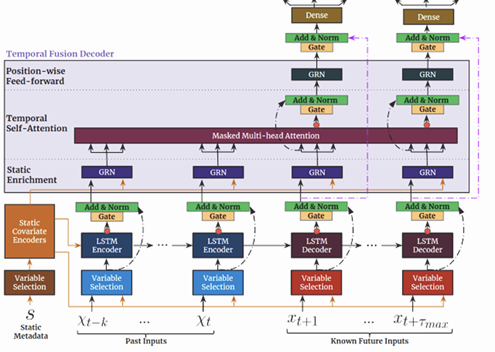
Using the prior situation of Covid, Sharon compared how other forms of demand prediction might be less effective for their business. For example, other forms of demand prediction could struggle in extrapolating the unique data which arose from the pandemic, such as the sudden bulk-buying of toilet paper, as these were values they hadn’t seen before in training. This is a gap covered by Picnic’s usage of TFTs.
Building on from the points made by Sharon, Rick’s own talk on demand forecasting at Ahold Delhaize , where he is a Principal Data Scientist, related also to the challenge of having physical stores in comparison to Picnic’s online-only presence. Just as with Sharon, Rick was quick to establish that “demand forecasting is at the core of our business”, showing how their unique solutions stem from the same critical business problem. For a company as large as Ahold Delhaize, this is clear in the approximately 1 billion predictions they make on a daily basis .
To extract key information about demand from this huge volume of data, Rick mentioned his company’s usage of tree-based models, which are fast, have good performance, and are non-linear. Thus, they capture interaction effects very well. With these models at their disposal, Ahold Delhaize have already made great strides in reducing their food wastage, and they are aspiring to cut down their overall food waste by 50 percent between the periods of 2016-2030. Rick also spoke about how his company handled the Covid pandemic, with his team identifying anomalous periods of demand and cutting them from their model predictions so they weren’t skewed.
Further information can be found from the paper Sharon recommended, from which Picnic’s TFT models were used: Temporal Fusion Transformers for interpretable multi-horizon time series forecasting. If you want to develop your knowledge of ML applications in retail even more, feel free to look at RE-WORK's AI in Retail Youtube playlist.
To watch Sharon and Rick's full presentations on Predicting article demand with Temporal Fusion Transformers and Reducing Food Waste by Demand Forecasting at Scale, as well as all the sessions from the RE•WORK London AI Summit across our Deep Learning, Conversational AI and AI in Retail tracks. All you need to do is purchase the On-Demand Access, where you will be provided with the recordings and slides of all of the sessions to watch back in your own time. Get you On-Demand Pass here.