Daphne Koller is the CEO and Founder of Insitro, a company that aims to rethink drug development using machine learning. We have had the pleasure of hearing the latest progress in drug discovery from Daphne at RE•WORK events, and have re-capped her talk at the last Deep Learning Summit in San Francisco below.
Topics Explored Include:
- The Challenges With the Costs of Getting Drugs Approved
- How to Leverage the Convergence Between the 2 Revolutions of ML and Cell Biology & Bioengineering
- Genome-Wide Association, With Genetical Variants and Clinical Outcomes
- The Process of Semantic Representation Learning
- The History of Science and Where We Stand
- The Two Different Perspectives of Machine Learning in Drug Discovery
- Erooms Law and Moore’s Law
See the full video presentation & complete transcript below.
[00:06]
So I'm very pleased this morning to tell you about my latest adventure, which is how one uses machine learning to help improve the process of drug discovery. In some ways, one can view a machine where drug discovery is today in two perspectives. There is the glass half full and the glass half empty. The glass half full is that in the last 20 years, many diseases that have been thought that had not had any cures and no treatments, except even barely palliative ones, are now seeing meaningful drugs that actually help considerably improve the lives of patients.
[00:44]
And those include different types of cancers, some of which can now be actually cured, including metastatic disease, cystic fibrosis, and many others. So that's the glass half full. The glass half empty is that even with all of these improvements we're in, we have this phenomenon that has come to be known as Eroom's law, Eroom's law is the inverse of Moore's Law, which is a law that we're all familiar with. Eroom's is the inverse of that, which is the exponential decline in the productivity of pharmaceutical R&D consistently over the last 70 years.
[01:20]
Now that's brought us to a point where, despite all of these improvements, the current cost to get a drug from idea to approval is over $2.5 billion and continually rising. And the aggregate success rate for the industry is about five per cent and holding steady. So when you ask yourself why that is, one of the main reasons for this is that we just have no ability in many cases to predict at early stages of the process which of the many paths that are open to us, whether it's a target, which of the many targets, which of the many drugs is actually going to be successful.
[01:55]
And we only find out years later and tens, if not hundreds of millions of dollars. So how do we make better predictions, and can we use machine learning to do that? And in this respect, we're now in the time where we're at a convergence of two revolutions that have been happening in two different communities, and it's time for them to meet. On the one side is the revolution in cell biology and bioengineering, where a range of technologies have emerged separately over the last five to ten years that have been transformative in their own right and when put together, are potentially a perfect storm in data production that can help feed machine learning models.
[02:38]
These include the ability to take a cell from any one of us here and revert it to what's called stem cell status, which allows it to then be differentiated into different normal cells, whether it's a neural cell or a cardiac cell or a liver cell that replicates our genetics. But as of the right lineage and therefore allows us to understand how disease manifests in those different cell types, we can further perturb those cells using genetic engineering techniques like CRISPR. We can assay these cells in many, many hundreds of thousands of different ways, different measurements that allow us to understand cell state and how that relates to disease.
[03:18]
And we can do that at an unprecedented scale using automation microfluidics that on the one side is able to create an explosion, a mountain of data the human will never be able to interpret. But on the other side, we have the machine learning revolution that's been able to come up with a way of deriving insights from such mountains of data. So how can we put those two together? So what we like to think about, as in Chitral, is about how to bring those how to leverage that convergence of revolutions.
[03:50]
So when we think about the pharma R&D pipeline and we like to look for those problems, that if we had the ability to make good predictions at a certain fork in the road, it would actually be transformative to the five percent success rate of R&D, where machine learning is actually the right tool for the job because it's not a silver bullet, it's not necessarily good for everything. And most importantly, perhaps, we have the ability to feed the machine learning with high-quality data because machine learning is only as good as the data that you feed it.
[04:22]
And so we've created a company that brings together bioengineers, machine learning people, and translational scientists to bring to solve all these problems together. So there are many problems that we could tackle, the one that we started out with is, I think at the core of drug discovery, which is what will an intervention do when you administer it to a person because most drugs actually fail because of lack of efficacy. So right now, that question is answered, oftentimes using mouse models of disease that people kind of construct say, hey, look, there's is a mouse that looks like it's feeling depressed.
[04:57]
So let's see if we can use that as a model for depression and develop antidepressant drugs. And honestly, these have very rarely been successful in translating to humans. So, the question is, how can you use humans as a model system for humans? Now, the challenge here, of course, is that it's not easy to create a data set where you actually make interventions in humans and see what the clinical outcomes that are called randomized clinical trials are small and expensive and by and rightly very carefully regulated.
[05:30]
So how does one collect the data set that is relevant to that but is much, much larger, and can feed machine learning? So we're able to bring together two types of data that are complementary to each other to try and solve this problem. The first of those is the growing amount of genomic data that has actually been growing on Moore's Law type scale. So you'll notice that this is a logarithmic scale and this is an exponentially growing graph in terms of the number of human genome sequences, the very first one in 2001, almost 20 years ago.
[06:02]
And not only is this graph growing exponentially, but it's also growing twice as fast as Moore's Law. So if you believe that this trend line will continue, the number of human genome sequences by about twenty twenty-five or twenty twenty-seven is going to be more than a billion, which is pretty amazing. Now, genomes on their own are useful. They're even more useful when you juxtapose them with phenotype data, clinical outcomes. There's less of that to be had today, but it's a growing resource.
[06:28]
One of our favorites in this regard is what's called the UK Biobank, where the UK government created a repository of five hundred thousand people who agreed to not only have their genetics measured but also thousands and thousands of very diverse phenotypes that include both everything from very, very granular biomarkers in blood or urine, whole body and brain imaging and various clinical outcomes that are actually measured over years by a connection to the National Health Service in the UK. So, when you juxtapose that with the genetics, it allows us to start understanding the causal connection between.
[07:06]
Nature's experiment in perturbing a gene and the clinical outcome that you see in a human and indeed when you put those two together, there's been over a decade's worth of work at this point in understanding what's called genome-wide association between genetic variants and clinical outcome, which gives us the starting point for understanding which genes might actually have a relevant clinical outcome if perturbed in a human. So, the problem is that there are literally hundreds of these for most diseases, so Alzheimer's, which is a complex trade, has hundreds of variants, Type two diabetes, almost pretty much every psychiatric ailment.
[07:48]
And it's very challenging to look at those hundreds of genes and say which of those is actually going to be a meaningful intervention in a human. And when you think about the fact that any drug program that you initiate cost you two point five billion dollars, that's a very high stakes experiment. So how does one leverage the other type of data to really refine this? And so, what we're looking at is bringing together that other revolution to understand which of those interventions actually might have a phenotype in human clinical outcome.
[08:21]
And so to give you an understanding of how that might work, I'm going to use this illustration. It's a very beautiful example, but there are hundreds of others that I could have used. This is a case study of a region in chromosome 16 that is called 16 P11 to which, for whatever reason, is subject to numerous copies, no alterations, and different people. Now, wild type is normal, but there is a good fraction of the population in which that region is deleted and another fraction where it's duplicated.
[08:51]
And the patients in which it's deleted have a seventy-five percent penetrance for autism. And those are which is duplicated, had a 40 percent penetrance for schizophrenia. And these are both very high numbers. Now there are 25 genes in the region, and we don't know we still don't know which of those actually or maybe more than one has these phenotypic effects. But what we can do is and what was done in a group at UCSF a little around three years ago is to take it is to take these what's called pluripotent stem cells from wild type controls and from deletion and duplication patients differentiate them into neurons and look at them under a microscope using high content fluorescent imaging.
[09:30]
And you can see even with the naked eye, that there are significant differences in the cellular phenotype across these different populations where on the left side you have a significant excess in synaptic urbanization and axial length. And then the duplication patients, you have a significant depletion of that phenotype. So, this is a very cautious grained analysis that was done by hand on three samples of each type. Even so, it shows statistical significance. But imagine if we could use this high throughput biology combined with machine learning to really dig down into the differences at the cellular level between these different populations and then search for interventions that revert the abnormal to the normal state.
[10:10]
That's basically what we're doing. And what we've built out at in seat row is you can see this in this video is a high-throughput biology lab that conducts that type of experiment that must have taken years and many people do this at scale. So, we have a bank of over a hundred IPS lines and growing, which you can take out of the freezer, prep it for differentiation and then you put it in the Saline management system, where an automated way the cells are differentiated and grow. I wish they grew this quickly, it actually takes weeks but they do grow, and then when you can take them out you put them in this amazing device which is called a lab site echo which perturbs the cells using either drugs or CRISPR reagents to affect individual activities of different genes. And this device manipulates the reagent cells using only acoustic liquid handling, so a pipette doesn't disturb the medium.
[11:15]
And it's incredibly reproducible and high quality at which point you can take the perturbed cells and measure their effect before and after using devices like this high content imaging system. Which is an automated microscope and see beautiful images like these ones on the next slide. So maybe we can just go to the next slide. And what you see on the next slide are many images that look like this and we have literally at this point 120 terabytes of images that look like this. And you can imagine that this is a hard thing for people to interpret because there is really a tremendous amount of data that people are not used to looking at and so that's where machine learning comes in.
[12:03]
Now in many audiences, we actually have to go and explain this notion of deep learning and end to end training and in this audience, I'm sure I don't need to do that but I will point out one aspect that we will use in our subsequent analysis. Which is the in addition to making good predictions and end to end training system like this also produces an alternative representation of the underlying data. So if you start out for instance just hypothetically with an image that's a thousand by a thousand that lives in a million-dimensional space. At the end of the neural network just before the classification is made, the factor is about a hundred dimensions. So, what effect that has done is it reduced a million-dimensional space into a hundred-dimensional space so from the perspective of a mathematical what that does is it actually defines a hundred-dimensional manifold in a million-dimensional space.
[12:52]
So this is just a visual depiction of a two dimensional manifold in a three-dimensional space because that's' as much as one can draw on a slide. And what's important to understand that the manifold that the machine learning selected is one that's designed to optimize predictive accuracy. And so, for instance, these trucks that these two images of trucks that are quite different from each other distance from each other in the original million-dimensional space, are actually by design need to be close to each other to the manifold. And other classes that share features even if they're labelled differently like the cars and the tractors will also be in the same region as space, whereas cats and dogs will be in a very different part in the space.
[13:31]
So what effectively this does is it learns a semantic representation that corresponds to some functional differences. So we're going to do that exact same thing with cells where the cells come from people with different levels of disease burden, sick people, healthy people, people with or without genetic penetrant genetically penetrant mutations and that's going to give us a manifold of cell state from which several things will emerge.
[14:00]
First of all, we're going to be able to find clusters of cells that might be identical at the clinical manifestation level but might quite different from each other at the cellular molecular level, and thereby indicating what subtypes of disease that we have not up until now been able to identify. That's actually been critical in areas like precision oncology, where we now know that breast cancer is not one thing and molecular subtypes of breast cancer are treated in very different ways. We've not been able to do that for most other diseases but perhaps now we'll be able to do that and then search for interventions in the system that for each of those clusters revert the unhealthy cellular state to a healthy cellular state, hopefully with the same effect on clinical outcome.
[14:49]
So this is the vision and this is how you know this is a long term project, we've only been operating for less than 18 months but even so in the 18 months, we've actually been able to put some of that into play. So, this is basically some experiments that show you could actually look at cells and figure out the phenotype that is relevant. So, this is taking actually cancer cells and identifying that were perturbed using different chemical reagents and asking can you look at the cells and figure out what was done to them.
[15:23]
This was a very significant amount of work because machine learning is not designed for images that are 20,000 by 80,000. It's designed for images that are 256 by 256, so we had to rebuild the firmware on the microscope, we had to rebuild the machine learning pipelines. But overall at the end of the day, the performance of this is much better than the state-of-the-art. We were able to do the same thing for genetic perturbation, this is a perturbation called CRISPR. It's a very subtle perturbation because it only decreases the expression of one gene by about 20-40 percent. And yet we're able to apply the same pipeline and get a significant improvement in accuracy and what's important is that this only took two days because we had already put in all the heavy lifting on the pipeline's here.
[16:12]
And then finally to show that this is not just for cancer cells we've also done this for differentiated neurons, differentiated from IPS cells and again you can see that we're able to distinguish different treatments to the cells as much better accuracy than the stay of the state-of-art. So just to wrap up, what we're really building is this unique closed feedback group between what you think of as a biological data factory, that is producing massive amounts of high-quality data using robotics and automation at scale with machine learning and genetics analysis on the other side with a very tight feedback loop that goes from what the machine learning has to do the experiment and back again, in a very tight integration.
[16:59]
So taking an even bigger step back just to wrap it up, I like to think of this work that we're doing in a historical context. Where if you look back at the history of science there have been certain periods of time where one discipline suddenly took off and made a tremendous amount of progress in a short amount of time, because of the new way of looking at things or a new discovery, or new technology. So, in the late 1800s that discipline was chemistry, where we discovered the periodic table and understood that you couldn't turn lead into gold. In the 1900s that discipline was physics, where we started to piece together the connection between matter and energy in-between space and time. In the 1950s that discipline was digital computing, where the invention of silicon microchips I was able to enable the computer to perform calculations that up until that point only people or sometimes not even people have been able to do.
[18:02]
And then in the 1990s, there was an interesting bifurcation where two fields took off at the same time. One of those fields was the field that I would call data, to feel that many of us are part of it emerged from computing but it's different from it because it also involves elements of statistics and optimization and other neural science that fed into that field. And then the other field was the field of what you might call quantitative biology that moved biology from an observational science that just catalogues plants with different shapes of leaves and such, into something that actually measured biology in robust and quantitative ways using increasingly high-throughput assays.
[18:42]
And these fields proceeded in parallel without a lot of overlap between them and I think the next big field that we're going to see emerging with a tremendous amount of progress is what I like to call the gentle biology. It's the ability to measure biology at an unprecedented scale, an unprecedented quality, uses the types of machine learning models as well as new ones to interpret what is it that we measure and then bring that back in right biology using technologies like CRISPR and others to get it to do things that it wouldn't normally otherwise do. And I think this will have significant implications across numerous fields that range from biomaterial engineering, environmental science, and importantly human health. And I think this is going to be a great field to be a part of in the upcoming decades so thank you very much.
You can watch all the videos here.
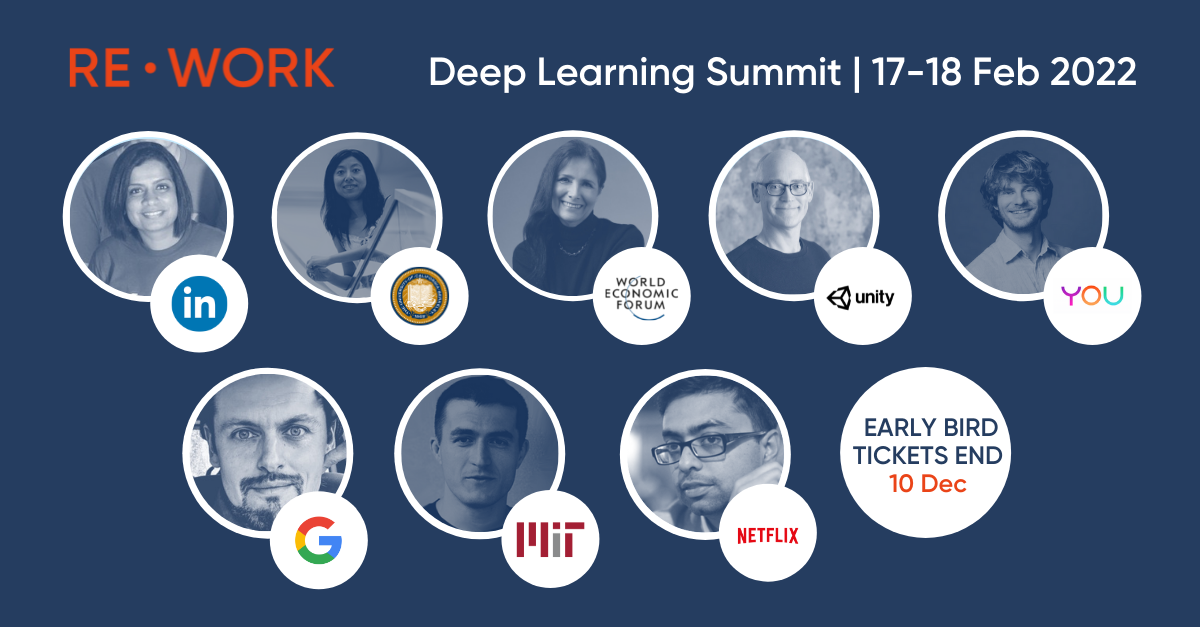
Join the Deep Learning Summit in San Francisco on 17-18 February 2022 to hear from 90 experts on Deep Learning, Reinforcement Learning, AI Ethics and Enterprise AI.