Inioluwa Deborah Raji has been in the news quite a lot recently, mainly due to her inclusion in the Forbes 30 Under 30 list for Technology influence. Deborah started on her path to Forbes recognition back in 2014, completing her Engineering Science degree at the University of Toronto. Upon completion of her degree, Deborah worked at Clarifai as an ML intern, MIT as a student researcher and the Partnership on AI as a research fellow. During her time at the University, Deborah founded Project Include, a non-profit initiative to increase access to Engineering Education in low income and immigrant communities ('Needs Improvement Area' designated neighbourhoods) in the Greater Toronto Area.
We had the chance to put some questions to Deborah prior to her talk at our recent Deep Learning Summit on the AI Ethics stage. Below are a few quick questions which Deborah was kind enough to answer for us.
Back in 2015, you founded Project Include. What was the main driving force for you to create this incredible concept?
"As an undergraduate, I had actually worked at a very fancy robotics camp at my university for a summer job. It was an incredible experience and a good job but definitely made it clear to me the divide between those that have access to such camps and learning opportunities and those who don’t. The camp was expensive, and hosted at the university, far from any neighbourhoods of people that looked like me or shared my background.
Unsurprisingly, I myself had only learnt to code in university, being completely shut out from opportunities to learn earlier. I understood that I’d have to take action to make this knowledge accessible. This is what led me and a couple incredible friends to start Project Include, an initiative where we could raise money to offer the camps for free, go into low-income and immigrant neighbourhoods (rather than have them come all the way to the university), and center the curriculum around problems that the community cared about."
You have worked as a mentee at Google and at the Partnership on AI institute. Do you see mentorship and teaching as something that will be prevalent throughout your career? How important do you think this structure is in developing future AI minds?
"I think it’s incredibly important to get mentorship - I’ve personally greatly benefitted from incredible mentors and hope to one day pay forward even a fraction of the opportunity their support and guidance has provided me. Mentors don’t need to look like their mentees - my first and most important mentor, Karl Martin, was so very different from me in every visible way but ended up being a key contributor to my success by believing in my ability, cheering me on, and doing all he could to support me regardless. I admired his character as well as his success and this is why I had initially reached out to him, because I knew he would have my back."
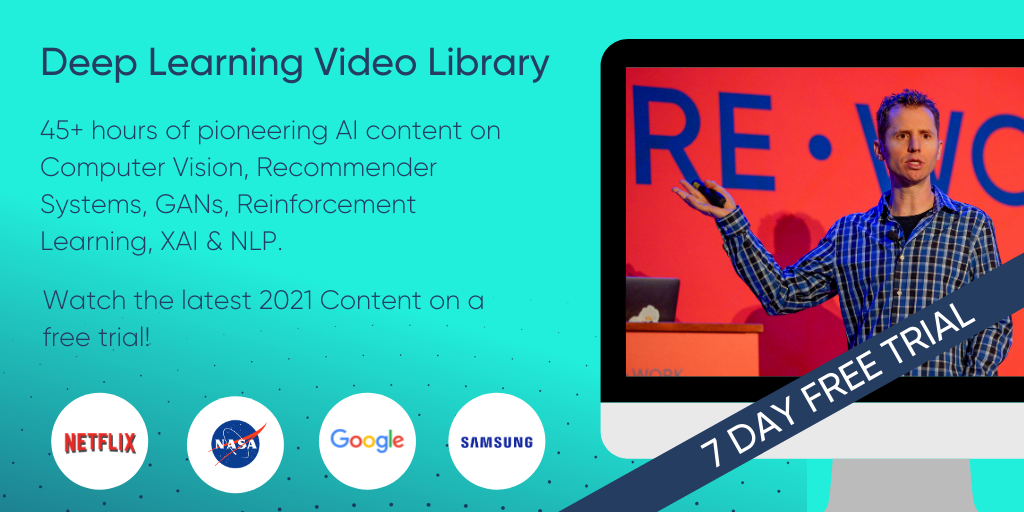
How important do you think this structure is in developing future AI minds?
"Having that support really gave me the courage to take the career risks I would later on, and other mentors have been integral to empowering me to stay in the field and persevere even when things got difficult. I encourage anyone given the opportunity to play this role in someone else’s life. It makes an enormous difference."
Earlier this year you were voted as one of Forbes 30 under 30, one of the youngest on the list. Your work on facial recognition bias was brilliant, when did you first realize this was such a prevalent issue in AI?
"I realized it was an issue when I was working as an intern at a machine learning company, and sifting through the data for a model I was developing at the time. It was actually a facial recognition model, and I noticed that none of the faces I was scanning through in the dataset looked like me. That was really alarming at the time, but when I tried to notify peers, the finding was met with indifference. I ended up getting very frustrated that no one around me seemed to be as concerned as I was about these issues - and that’s actually how I ended up reaching out to Joy and working with her. Right now, my thinking has evolved a bit on the issue."
What are the biggest changes in terms of addressing bias in AI that we will see in the near (and far) future?
"I’m more aware now of how facial recognition can be harmful, even when it does work and do think there’s been some great advancement with this work in conjunction to developing privacy policy. Also, I’ve since done work on internal auditing in addition to this external audit work, so I have a better sense of what’s going inside of these companies, and the blindspots in the engineering process that reveal themselves. I think we’ll see a lot more talk about these blindspots and engineering challenges and hopefully a shift towards pressuring the field as a whole to be more responsible about what they create."
Can you tell us a little more about what you will be working on at Mozilla?
"I’ll be thinking more about algorithmic accountability and particularly reflecting on this internal vs. external audit dynamic, who each have different situations and circumstances. I’m trying to understand how policy influences the reality of the situation of both internal and external auditors hoping to hold the companies accountable, and exactly what kind of challenges each group is battling in their attempt to do so."
If you could give us one thing to take away from your talk today, what would it be?
"I hope people take away that “AI doesn’t work unless it works for everyone.” The idea that a technology that’s biased and dangerous can still be deployed on millions is unbelievable to me. I really hope the field does better moving forward."
Top 5 Read Blogs of January '21
AI Experts Predict 2021 Trends
AI Experts Suggest 13 Roadblocks & Limitations to AI Progression in 2021
30 Influential Women Advancing AI in 2020
13 ‘Must-Read’ Papers from AI Experts
How Netflix uses AI to Predict Your Next Series Binge - 2020