We were delighted to be joined by Doina Precup, Research Team Lead at DeepMind in Montreal, at the Deep Learning Summit. Doina took to the stage to discuss the latest developments in Reinforcement Learning and how it can be used as a tool for building knowledge bases for AI Agents. Doina began her talk by first giving an overview of Reinforcement Learning (RL) and detailed the contents of her presentation to come.
“Reinforcement learning allows autonomous agents to learn how to act in a stochastic, unknown environment, with which they can interact.”
Doina continued to suggest that Deep Reinforcement Learning, in particular, has achieved great success in well-defined application domains, such as Go or chess, in which an agent has to learn how to act and there is a clear success criterion. The role of RL lies in being a tool for building knowledge representations in AI agents whose goal is to perform continual learning. That said, Doina discussed Reinforcement Learning as something which is inspired by animal learning, that is, an agent which is interacting with an environment with reward functions made available to those who succeed and negative reward for those which are not successful, akin to laboratory mice used in early scientific experimentation. Doina joked that whilst the development of capability shown in Reinforcement Learning was scary to some, she finds it to be extremely exciting.
“Whilst it is restrictive at this time, and does not have the messiness of life to contend with, I, one day dream, that this capability can be powerful enough to power Poutine robots.”
Whilst the audience laughed, there was some logic behind this statement, with Doina suggesting that from the viewpoint of advancing AI, this would be a great task. How you ask? Well, whilst we do not see cooking as a benchmark for intelligence, it would require regular troubleshooting, an advancement of knowledge and the ability to interact with an environment which is far less structural than those shown in video games. The underlying point? That we can use RL not just to learn simple problem solving skills, but to build knowledge which can be applied to wider societal tasks. Further still, Doina suggested that the procedural knowledge capability of alphaGO can only complete an action against a current state and value function which shows estimation of expectation against long time return. This is something we want to overcome when looking forward. The next step, of course, is one of much greater difficulties, as we face the problematic creation of opinion and allowing an agent to make its own choice in tasks of greater complexity.
The decision making function of agents is only really called into practice when a point of impasse is reached, be it an enemy in a game or an impassable object in the virtual world. A system that can be programmed to achieve a goal following set instructions is one thing, however, the idea that we can encourage random exploration from an agent and expect great results is not one which is realistic at this time. The next step in this development, Doina suggested, could take great influence from actor-critic architecture, however, it was suggested that this would require an increase in signals from the value functions available due to the increasing complexity of the tasks. The example of option critic success was given with reference to Atari. Through Atari, DeepMind have seen option critics perform at the same level as DQN. Why is this important? It can be used cross task and it is leveraging new knowledge acquired which can then be used outside of Atari and video game scenarios.
Doina suggested that we should not expect this development in too much haste, mainly because we have spent that last thirty years helping agents build predictive knowledge. In the latter part of her talk, Doina also talked generalisation with regards to DRL algorithms, touching on the ability to generalize in two ways, through both general cumulant functions and continuation functions, the latter of which aids considerably in mapping model states. That said, we must recognise that even with generalisation possible, we must focus more so on the incremental changes possible, referenced to by Doina as lego bricks, which can see smaller value functions added together over a period of time. This should be seen as a way forward which can aid in the development of groundbreaking algorithms as there is seemingly no longer just a ‘single task’. The idea that a ‘single task’ can be the base layer for development on which building blocks are placed is shortsighted, with suggestions made that the whole Data Science field need to agree to a re-think in regard to empirical evaluations of models. There is, in fact, a need to formulate a hypothesis about what the agent should know or how it should behave, given certain knowledge, as whilst the returns are important, they are too simplistic at this stage.
Doina brought her talk to a close with two points which gave food for thought surrounding the current Deep Learning landscape. The first was patience, many are too quick to try and advance Reinforcement Learning through tinkering to develop new algorithmic possibilities without allowing RL the time to learn for itself. The second is somewhat of an open question right now. We have reached a stage at which we are weighing the value functions and understanding what the agent would like to do. How can we aggregate an agents actions? That, we’re not quite sure on yet!
Speaker Profile
Doina Precup holds a Canada Research Chair, Tier I in Machine Learning at McGill University, Montreal, Canada, and she currently co-directs the Reasoning and Learning Lab in the School of Computer Science. Prof. Precup also serves as Associate Dean, Research, Faculty of Science and Associate Scientific Director of the Healthy Brains for Healthy Lives CFREF-funded research program at McGill. Prof. Precup’s research interests are in the area of artificial intelligence and machine learning, with emphasis on reinforcement learning, deep learning, time series analysis, and various applications of these methods. She is a Senior Member of the American Association for Artificial Intelligence.
Join us at the next edition of the Deep Learning Summit Series or start a free trial for our Video Library. Hear from the likes of OpenAI, UC Berkeley, Uber AI Labs and Google Brain.
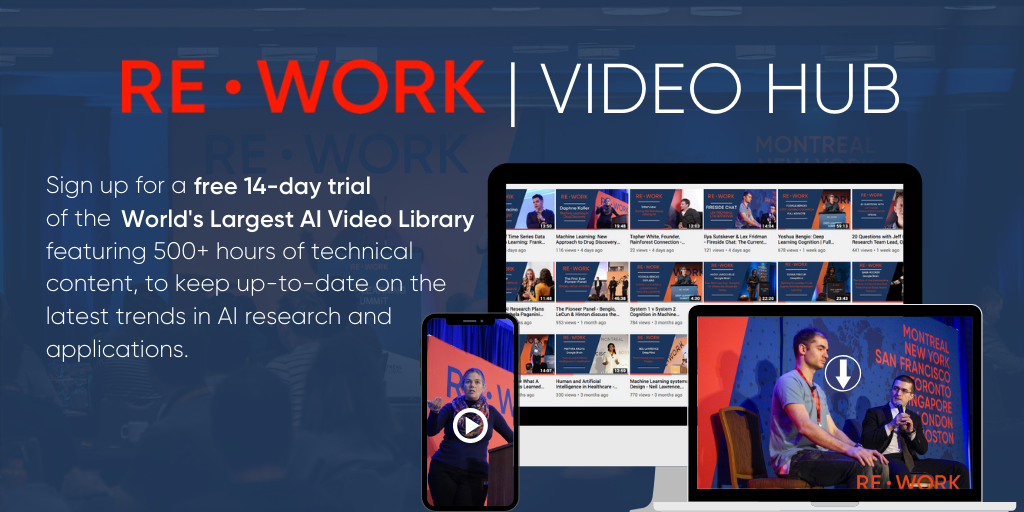