It is essential that trust is built into the deployment, and development of AI systems. With the prevalence of AI's role in almost every area of society, trust and robustness are more important now than ever for the deployment of AI at scale.
We caught up with superwise.ai CEO & Co-Founder, Ofer Razon, after their involvement in the AI Applications Virtual Summit in September, to hear more about how they address the challenge of AI assurance through understanding insights about the ML process to scale AI with confidence.
How Did You Begin Your Career in the AI Space, and What Led to You Co-Founding superwise.ai?
I've been doing AI since before it was popular to call it AI—it was big data, and before that predictive analytics. In my previous role at Amdocs, I worked with a lot of C-level executives, for pretty much every telco on the planet.
That was always interesting because it was back when people started to realize that AI and machine learning were something they needed to have on their agenda. You could see the confusion as most of them were in the phase where they hired a group of data scientists and put some big data Hadoop infrastructure in place to collect a lot of data. In some cases, they came up with something interesting that could be developed. But when you would ask the CIO or the CTO: “what is it going to look like when it's used at scale?”, or “how is that going to happen?”, and “what’s going to happen on the day after that?” They had no idea.
Besides, my co-founder, Oren Razon, also led multiple Machine Learning projects, developing solutions and AI Solutions for customers across all verticals. When the solution was ready, some customers were afraid to launch it and trust it because they didn't know how it was going to operate on Day 2. It was clear to us that there was a huge gap that needed to be addressed.
Can You Explain What You Mean by ‘AI Assurance’?
AI Assurance is all about ensuring that your AI is going to operate in an optimal and risk-free method over time. This is the objective. This statement comprises different things:
1 - The need to monitor models and ensure they don't have any performance pitfalls. Maybe there’s a specific audience or specific subgroup of your data, or a specific segment of your customers where your model is not performing as well as you expect, and you need to understand that to make optimal use of your AI’s predictions.
2 - The ability to have the observability and the transparency you want—to have the analytics and insights to be able to look and understand how your model operates, whether it's about the data it is processing, the inference of the model, or the performance, and then the ability to slice and dice it to different subgroups to identify weak spots in your model.
3 - Bias and fairness. A lot of the issues around bias have to do with the way you develop and build your datasets during the development of your models. But, how do you make sure that your bias levels, or unbiased levels, remain in your safety zone when the model goes live? You don't have control anymore over the data and over the feedback that's going to come back.
And last but not least, there is the organizational challenge: who owns the models in production? Is it the data science team? The operational ones? This question comes back in every meeting we have with our prospects and customers.
What Insights Should AI Assurance Tools Provide?
At superwise.ai, we look at AI assurance as a combination of the following: practical capabilities to extract, in time, easily understandable insights about the ML process, and tools to create a common language between data science and operational teams.
The types of insights we derive are as follows:
- A thorough understanding of the model’s health: with metrics and performance tracking over time and versions, and automatic predictions of performance levels to circumvent blindspot periods.
- Alerts on drifts/biases to enable more proactivity and prevent AI failures: data and concept drifts, biases, performance issues, and correlated events to avoid too much noise.
- Business insights through granular information. AI assurance is about bridging the gap between data science and operational teams and this requires high resolution and a sub-segment view of the populations impacted by the model’s predictions.
- Key insights on the real-life behaviour of the models for future optimizations.
How Can This Be Applied to Solve Challenges in the Real-World, for Example for Fraud Detection?
We address the needs of data science and operational teams.
For the data science teams we support, AI assurance means being alerted on concept drifts before they become a liability, or understanding when it is worth creating a model for specific merchants. Last but not least, this requires them to have a clear view of when to retrain their models, and with which data to do so while avoiding unnecessary "noise”.
For fraud analyst teams, who often find themselves drowning in anomalous and suspicious activity alerts, it is crucial to validate the decisions automatically taken by the AI to avoid merchants’ and end customers dissatisfaction - or worse, $$ loss and brand damage. For instance, one of the fraud teams we support managed to dramatically reduce the time it took them to detect a new fraud pattern in a specific industry - from roughly 3 weeks to 3 days, by getting a timely alert on specific segments that contain a high level of uncertainty, and by understanding the data changes that caused it.
What Are the Key Challenges Faced for Companies Adopting and Trusting AI at Scale?
There’s a consensus among ML professionals today that there’s a gap in the industry. As more and more models are moving to the deployment or production phase, ML professionals lack a clear and practical understanding of the KPIs necessary to monitor the health and performance of their models.
But metrics are not the only missing piece in the MLOps puzzle. Monitoring ML in production also requires bridging an organizational gap: one that exists between the needs of the data science teams for clear KPIs, and the needs of the operational/business teams for more visibility into, and a better understanding of, the processes that lead to predictions being made. And this gap can only be bridged with a more encompassing view, and not solely through the eyes of the data science teams. Especially if companies want to scale their ML activities.
At the end of the day, the main challenges I’ve witnessed are around these two gaps: the technical one that focuses on the needs of the data science teams, and the organisational one that’s about ownership and the question of whose responsibility it is to manage the AI and its results in the organization. Because once the models go live, they have a life of their own that’s closer to the business and the operations.
What Are the Main Challenges of Data Quality in the AI Ecosystem?
Traditional data quality practices look at the health of the data through the lens of the infrastructure: is the data consistent? Is it valid ? Yet, MLOps and AI assurance require the establishment of a new layer, one that will look at the quality of the data from the point of view of the quality of the predictions, and that touches upon the different stages of the ML process: at the inference level, and at the predictions level. Is the data being used during the ongoing period, different from the data used to train the model? What does that mean for the predictions?
How Crucial Is MLOps to Help Businesses Realise MLS Full Potential?
ML cannot do without MLOps and without monitoring. As most organizations are scaling their use AI, they need the best possible infrastructure for their ML activities. For our customers, this means answering the following questions:
- How are my models going to behave in the real world, with the scale and velocity of data to be processed?
- How can I ensure the health of my models at the right time?
- Who owns the models in production?
- How do I drive efficiency with the teams involved?
How Has covid19 Impacted superwise.ai and Your Work in Progressing AI?
COVID-19 has had several impacts on the market dynamics around AI in general and AI monitoring in particular. First of all, COVID-19 has shown the whole world that models are only as reliable as the data they have been trained on and that models are fragile and prone to errors. In this sense, it has increased awareness of the AI monitoring and AI assurance space, and in the fact that the world was changing rapidly and that a layer of solutions was needed to keep models safe.
Are There Any Projects or New Releases You Have Coming up That You Can Tell Us About?
At this stage we are expanding the variety of use cases we support through our multiple installations: from adversarial types of use cases with fraud prevention or security, to more marketing-focused applications with CLV, churn, intent predictions; as well as healthcare, credit scoring and underwriting, just to name a few.
What Do superwise.ai Have Planned for the Rest of 2020 and Move Into 2021?
Moving into 2021, we really want to support the growth of the AI assurance space through more education and meaningful conversations on what it means to monitor your AI in production. Just a few weeks ago, we led a webinar during which we polled the participants. Amongst 50 attendees, almost half of them had between 5-15 models in production but less than 5% really had monitoring in place for their models in production. These figures confirm that organisations are accelerating their use of AI but are not yet ready for the next steps: the real world.
About superwise.ai
Their solution enables data science and business operations teams to reduce the labour intensive efforts invested in the maintenance of AI in production, and shorten the time to detect and fix issues. They monitor and assure the health of AI-based models for global players in the area of financial services, fraud prevention, insurances, and marketing.
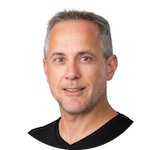
Ofer Razon, CEO at superwise.ai has had over 20 years of experience as a business and technology leader, innovating, incubating and growing enterprise software products across industries and geographies. He has been involved with dozens of big data, analytics and AI implementations.
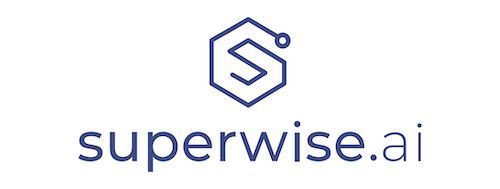
You can re-watch all the presentations from the AI Applications Virtual Summit held in September 2020 here. Sign up today for a free 7-day trial.