Recent developments in AI and deep learning have the potential to transform the way that banks and financial services firms do business, including but not limited to customer service, portfolio management, and fraud detection.
But will 2022 be the year that advanced AI and machine learning techniques take off in the world of financial services?
As we approach RE•WORK’s upcoming London AI Finance Summit on 17-18 March, we asked some of our expert speakers what they predict for AI and deep learning in Finance, Insurance and RegTech in the coming year.
Leon Saunders Calvert, Head of Research & Portfolio Analytics, London Stock Exchange Group
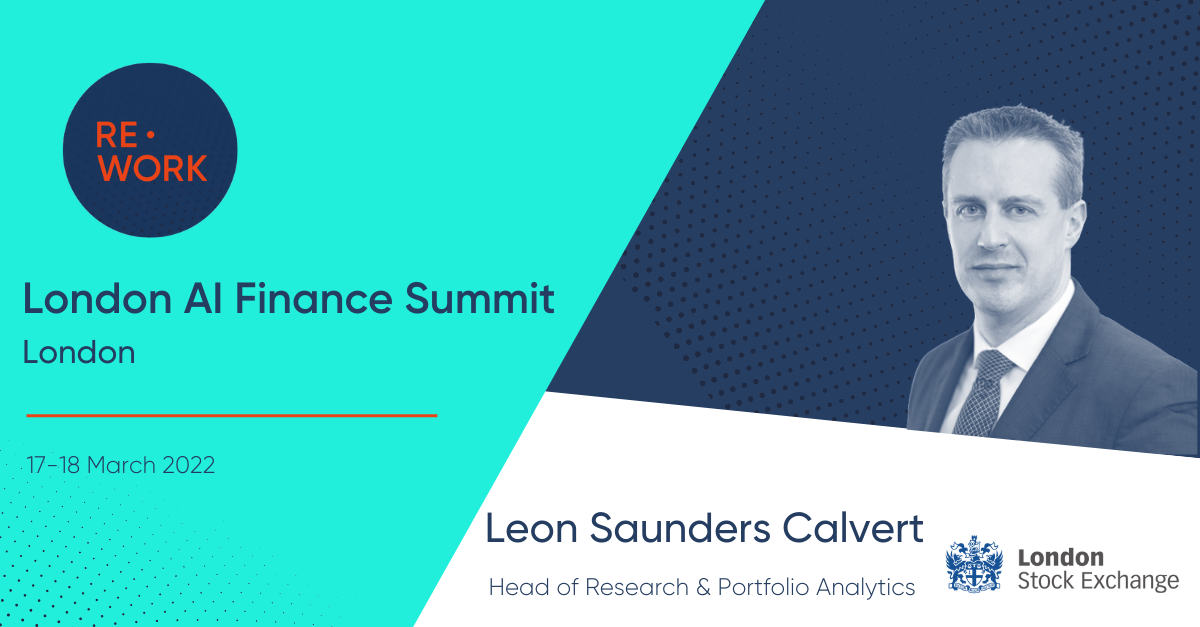
Question: Which trend associated with deep learning and AI are you most interested in or passionate about? Why do you think this is so relevant today?
Text summarization and sentiment. Recent improvements in deep learning have meant that outputs on text analysis have improved significantly to become more actionable.
Financial analysts have an overload of information and data to parse, synthesize, disregard, etc. Text is one of the more complex datasets to work with – to appropriately capture nuances, themes, sentiments – to summarize these and create useful metadata from them. These improvements have not yet led to full integration into financial practices. This is still to be scaled.
Question: What are the top three challenges that the industry will face in 2022, and why?
1. Realizing the limitations of self-supervised AI. Technologists tend to overplay their hands with regards to what deep learning and AI can meaningfully accomplish.
Sophisticated pattern recognition is powerful and can be extremely useful. But the inability for AI to truly change the workflow of the financial analyst and replace much of the highly specific analytical work that informs the creation of investment-grade data and models threatens to create a backlash against the profile of AI.
2. A backlash against AI outputs. The inherent biases of user-defined assumptions and/or content being analyzed carry prejudices. Damage has been done to AI’s reputation due to its contribution to the creation of the ‘filter bubbles’ and ‘echo chambers’ that have dogged ‘big tech’. This will not help the adoption of AI in financial markets where analysts are specifically attempting to create insights that are non-conformist and create value outside of the consensus view.
3. As much as deep learning enthusiasts would have you believe otherwise; big data still underpins AI activity in institutional finance. This is because investment decisions need to be based on key facts, which cannot afford to be wrong, to create the inferences that AI tools can help generate. The cliché of ‘rubbish in, rubbish out’ still holds sway in most financial use cases and is hard to bypass.
Adam McMurchie, Lead Cloud Data Engineer, NatWest
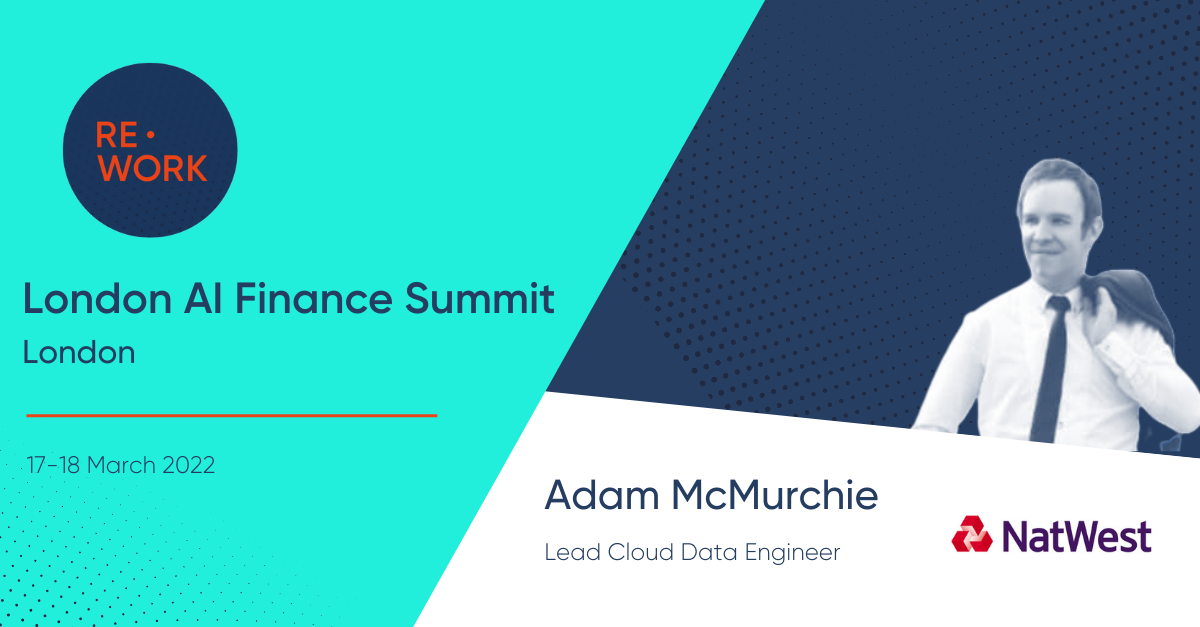
Question: What are the top three deep learning trends you think will take AI to the next level in 2022, and why?
1. Model fusion. This means integrating more than one AI/ML function into a product or service.
Sounds simple? It’s not – just ask Tesla. We are not talking about simply having lots of ML models but integrating them, so they function constructively.
Tesla fuses computer vision with prediction and path/planning to create a seamless driver experience. Banks are also going this way too, where chatbots don’t just hand off to an advisor or service function but oversee the end-to-end fulfillment of a customer’s request.
2. Human augmentation and its subset, hyper-personalization,are making big moves in 2022.
Most financial institutions are no longer asking ‘what can we do with customer data?’ but ‘what data do we have that our competitors don’t which can deliver 10x improvements in the customer experience’.
In other words, the focus is now on digging deeper and enriching the consumer journey with subtleties that only well-designed ML services can pick up on. While Facebook’s metaverse stocks are tanking, the need for ‘fusion apps’ has not, as the Chinese integrated social media and payments app WeChat proves.
3. AI mechanization is simply the fulfillment of the past 10 years of progress in ML. The current wave of AI has long iterated out of the lab, out of bespoke usage, even beyond that of the expert-only domain.
Much like Excel or the internet, everyone will utilize AI in their day-to-day activities; expect cheaper ML platforms, out the box solutions for the non-techies, a variety of options, and ease of use. Many mature products in finance already have some form of premium AI service baked in.
Ansgar Koene, Senior Research Fellow at Horizon Digital Economy Research Institute, University of Nottingham
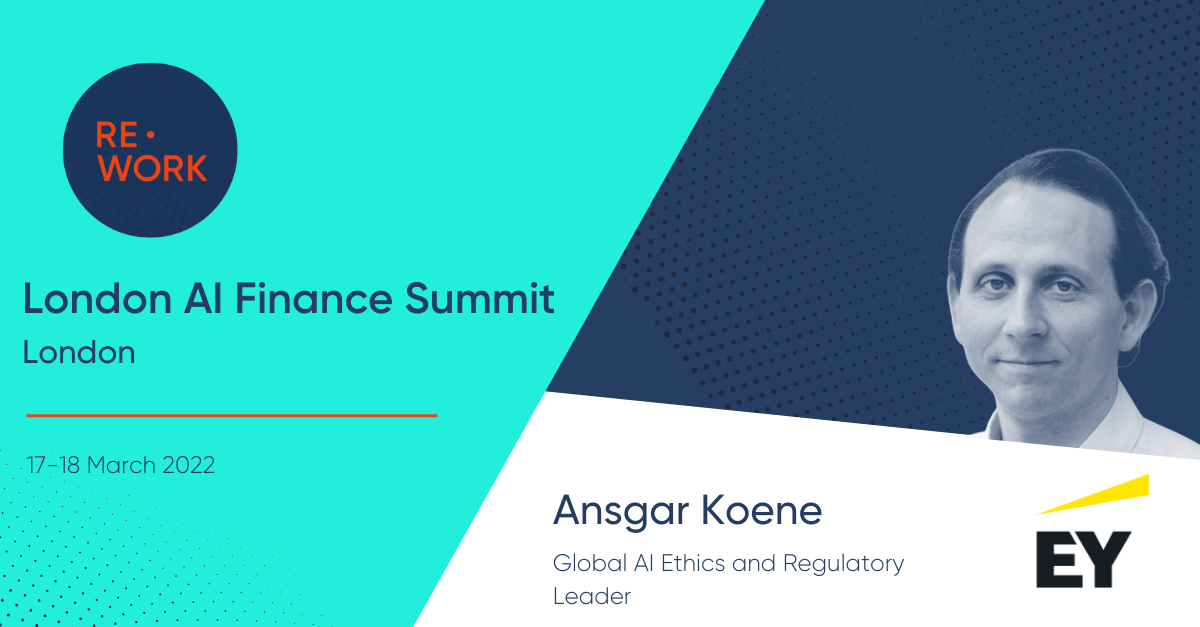
Question: Which trend associated with deep learning and AI are you most interested in or passionate about? Why do you think this is so relevant today?
When it comes to AI, I am most passionate about the move to pro-actively think about the larger implications that the use of this technology may bring to people, society, and the environment.
The fact that discussion about AI ethics principles, and their implementation, is being recognized as a core part of thinking about AI development, deployment, and regulation.
Question: What are the top three deep learning trends you think will take AI to the next level in 2022, and why?
The most important developments for taking AI to the next level are related to improving the understandability of models. This is so that we can be sure they are doing what we want, and we can better understand the deployment context envelope where the system can reliably be used.
Associated with this development is the vital maturation of AI/MLOps to ensure that the AI systems are properly documented and monitored.
Question: What are the top three challenges that the industry will face in 2022, and why?
From a regulatory perspective, the big challenge that the industry will face is the introduction of new legislation that will raise the bar on governance procedure, documentation, and reporting requirements.
For a globally operating industry like the AI sector, the focus will have to be on maximizing global coherence and synergy between regulatory approaches to AI.
Growing concerns about the environmental impacts of developing models with ever-increasing data and compute requirements are going to pose a significant challenge that will require a rebalancing of priorities in some areas.
Factors contributing to this will be not just energy consumption but also concerns about e-waste as compute hardware is upgraded.
Luke Vilain, Data Ethics Senior Manager, Lloyds Banking Group
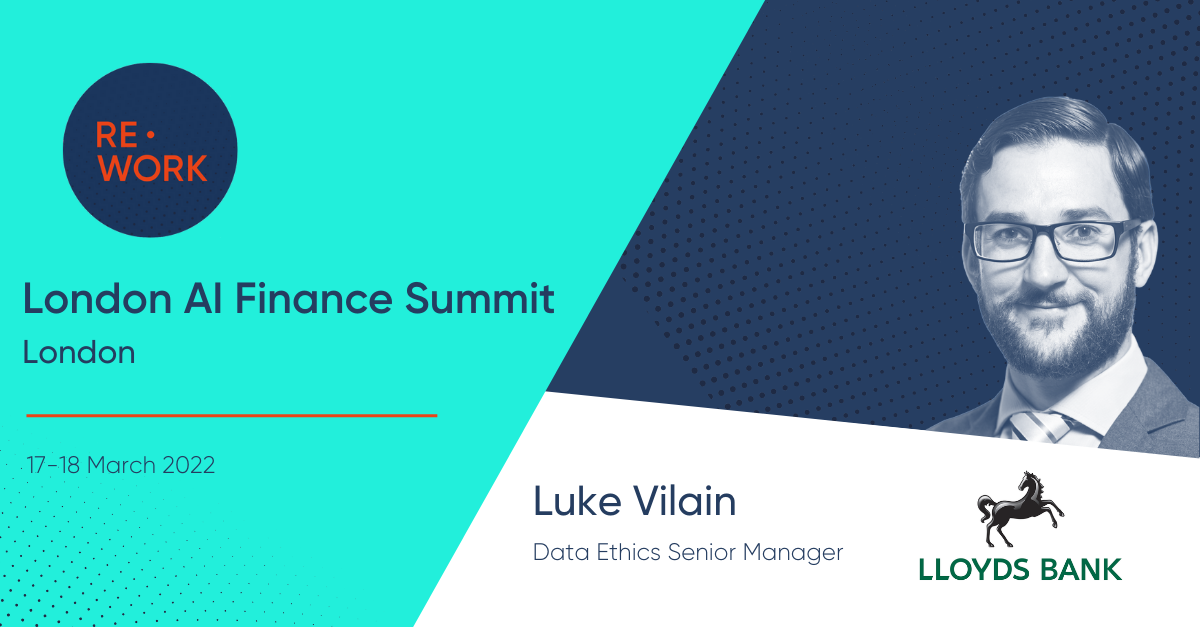
Question: Which trend associated with deep learning and AI are you most interested in or passionate about? Why do you think this is so relevant today?
It’s a tie between explainability and fairness. This is a make-or-break topic for people’s trust in AI systems. I’m not from an AI background, but seeing pipelines and models being built has convinced me that this technology holds great promise and value, but only if we use it responsibly
Question: What are the top three challenges that the industry will face in 2022, and why?
1. Data. To make data useful there needs to be both investment and cultural change to improve its quality and availability. It can be boring to manage data well, and the payoff can be invisible to a data handler
2. Expectations. There has been a lot of investment in machine learning teams and platforms, however, these teams are still struggling to implement production-grade solutions at scale, for a variety of reasons. These include platform and data issues, governance and oversight burdens, business stakeholders not wanting to use unproven technology on core customer-facing technologies, to name a few.
3. Regulation. Upcoming legislation creates uncertainty about what compliance will need to look like
Frans van Bruggen, Policy Officer FinTech and AI, De Neverlands Bank
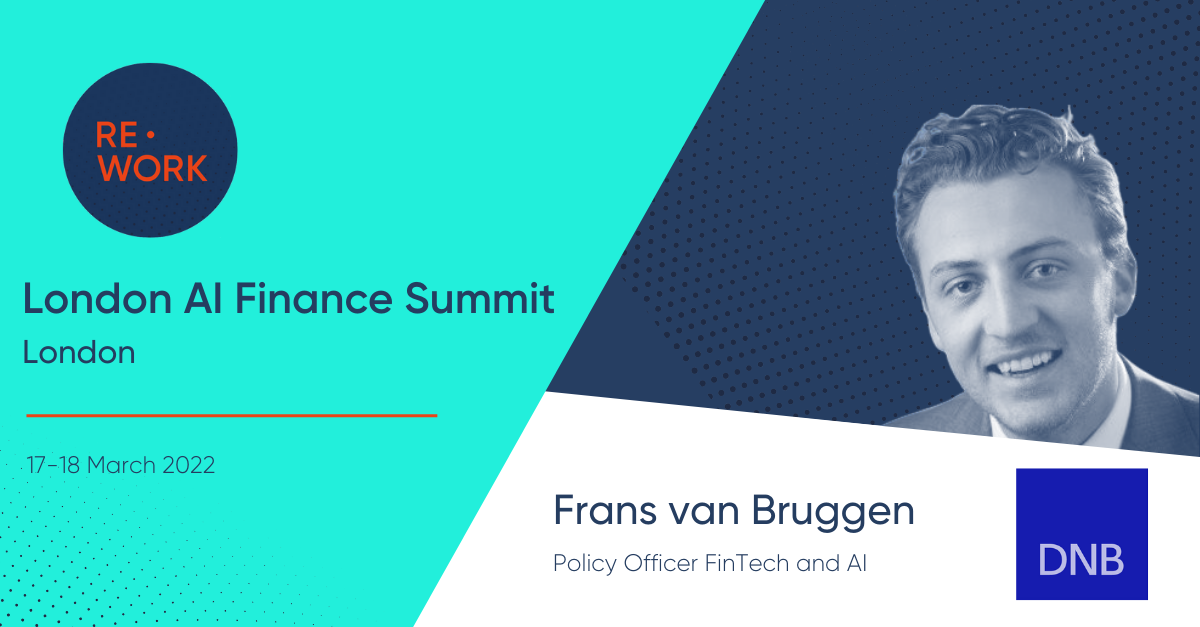
Question: Which trend associated with deep learning and AI are you most interested in or passionate about? Why do you think this is so relevant today?
I’m most passionate about mind/machine collaboration. There are a lot of dystopic visions around AI. But I think that if we combine the capabilities of humans with machines, we will be able to achieve amazing things.
Question: What are the top three deep learning trends you think will take AI to the next level in 2022, and why?
1. Integrated neural networks: when the now very specific tasks that AI can perform can be combined.
2. Generative adversarial networks (GAN): especially the use in science and gaming is looking impressive.
3. Federated learning: this enables multiple actors to build a common, robust machine learning model without sharing data.
Question: What are the top three challenges that the industry will face in 2022, and why?
1. Algorithmic interaction: how do we open the black box within the black box?
2. GAN’s (deep fakes): how will we be able to detect deepfakes?
3. Use of synthetic data: how do we decide what the ‘fair’ dataset looks like.
Discover the full line-up of world-leading AI and ML experts at our upcoming London AI Finance Summit on 17–18 March 2022.
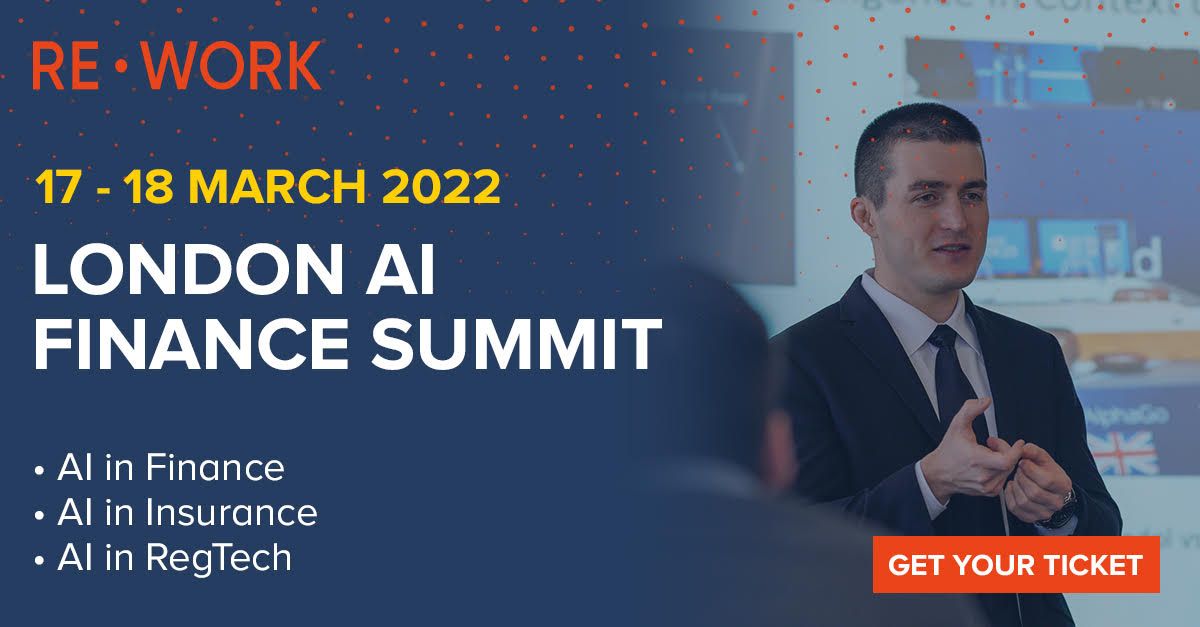